Research Article | OPEN ACCESS
Construction of a Health Food Demand Prediction Model Using a Back Propagation Neural Network
Han-Chen Huang
Department of Leisure Management, Yu Da University, 36143 Taiwan
Advance Journal of Food Science and Technology 2013 7:896-899
Received: March 19, 2013 | Accepted: April 02, 2013 | Published: July 05, 2013
Abstract
For business operations, determining market demands is necessary for enterprises in establishing appropriate purchase, production and sales plans. However, many enterprises lack this ability, causing them to make risky purchasing decisions. This study combines a back propagation neural network and the Particle Swarm Optimization Algorithm (PSOBPN) to construct a demand prediction model. Using a grey relational analysis, we selected factors that have a high correlation to market demands. These factors were employed to train the prediction model and were used as input factors to predict market demands. The results obtained from the prediction model were compared with those of the experiential estimation model used by health food companies. The comparison showed that the accuracy of PSOBPN predictions was superior to that of the experiential estimation method. Therefore, the prediction model proposed in this study provides reliable and highly efficient analysis data for decision-makers in enterprises.
Keywords:
Artificial neural network, demand prediction, particle swarm optimization algorithm,
Competing interests
The authors have no competing interests.
Open Access Policy
This article is distributed under the terms of the Creative Commons Attribution 4.0 International License (http://creativecommons.org/licenses/by/4.0/), which permits unrestricted use, distribution, and reproduction in any medium, provided you give appropriate credit to the original author(s) and the source, provide a link to the Creative Commons license, and indicate if changes were made.
Copyright
The authors have no competing interests.
|
|
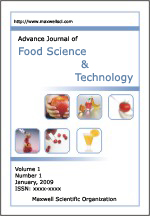 |
ISSN (Online): 2042-4876
ISSN (Print): 2042-4868 |
 |
Information |
|
|
|
Sales & Services |
|
|
|