Research Article | OPEN ACCESS
Food Quality Detection Using Machine Vision Based on Genetic Optimized RBF Network
Wei Ding
Nanyang Institute of Technology, Nanyang 470004, China
Advance Journal of Food Science and Technology 2014 8:981-983
Received: March 22, 2014 | Accepted: April 28, 2014 | Published: August 10, 2014
Abstract
This study aims to investigate the food quality detection using an intelligent method. The machine vision applies image processing softwares to monitor the food quality. The Artificial Neural Network (ANN) in the image processing softwares is crucial for food quality detection precision. However, improper structure parameters of ANN may lead to the low detection performance. In order to overcome this problem, a new detection method based on Genetic Algorithm (GA) -Chaos optimized Radial Basis Function (RBF) neural network is proposed in this study. The GA-Chaos was used to optimize the structure of the RBF as well as its weight values to obtain high generalization ability of the RBF-detection model. Then the RBF model was employed to train and test the food data sets. Experimental results show that the method could enhance the food quality detection rate and outperforms the traditional GA-based methods.
Keywords:
Food quality detection, image processing, machine vision,
References
-
Bennedsen, B. and D. Peterson, 2004. Identification of apple stem and calyx using unsupervised feature extraction. T. ASAE, 47(3): 889-894.
CrossRef -
Cubero, S., N. Aleixos, E. Molt?, J. G?mez-Sanchis and J. Blasco, 2011. Advances in machine vision applications for automatic inspection and quality evaluation of fruits and vegetables. Food Bioprocess Tech., 4(4): 487-504.
CrossRef
-
Fu, S., S. Cai and X. Zhu, 2012. Design of intelligently online detection and analysis system for automobile transmission. Int. J. Digit. Content Technol. Appl., 6(11): 282-288.
CrossRef
- Li, H., 2013. Application research of food quality detection based on computer vision. J. Comput., 8(7): 1758-1762.
CrossRef
- Liang, M. and D. Meng, 2012. Quality detection method research and simulation based on rice images. Comput. Simulat., 14(6): 23-27.
-
Vapnik, V., 1995. The Nature of Statistical Learning Theory. 1st Edn., Springer-Verlag, Berlin.
CrossRef PMid:8555380
- Xiao, X. and L. Peng, 2012. Research of rice figure detection based on improved ant colony algorithm for image edge detection. Microcomp. Appl., 21(13): 85-90.
Competing interests
The authors have no competing interests.
Open Access Policy
This article is distributed under the terms of the Creative Commons Attribution 4.0 International License (http://creativecommons.org/licenses/by/4.0/), which permits unrestricted use, distribution, and reproduction in any medium, provided you give appropriate credit to the original author(s) and the source, provide a link to the Creative Commons license, and indicate if changes were made.
Copyright
The authors have no competing interests.
|
|
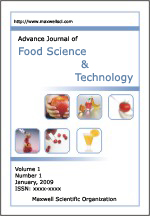 |
ISSN (Online): 2042-4876
ISSN (Print): 2042-4868 |
 |
Information |
|
|
|
Sales & Services |
|
|
|