Research Article | OPEN ACCESS
A Precise Distance Metric for Mixed Data Clustering using Chi-square Statistics
S. Mohanavalli and S.M. Jaisakthi
SSN College of Engineering, Chennai, Tamil Nadu-603110, India
Research Journal of Applied Sciences, Engineering and Technology 2015 12:1441-1444
Received: May 1, 2015 | Accepted: May 10, 2015 | Published: August 25, 2015
Abstract
In today's scenario, data is available as a mix of numerical and categorical values. Traditional data clustering algorithms perform well for numerical data but produce poor clustering results for mixed data. For better partitioning, the distance metric used should be capable of discriminating the data points with mixed attributes. The distance measure should appropriately balance the categorical distance as well as numerical distance. In this study we have proposed a chi-square based statistical approach to determine the weight of the attributes. This weight vector is used to derive the distance matrix of the mixed dataset. The distance matrix is used to cluster the data points using the traditional clustering algorithms. Experiments have been carried out using the UCI benchmark datasets, heart, credit and vote. Apart from these data sets we have also tested our proposed method using a real-time bank data set. The accuracy of the clustering results obtained are better than those of the existing works.
Keywords:
Chi-square statistics , clustering , mixed data attributes,
References
-
Ahmad, A. and L. Dey, 2007. A k-mean clustering algorithm for mixed numeric and categorical data. Data Knowl. Eng., 63(2): 503-527.
CrossRef -
Bai, L., J. Liang, C. Dang and F. Cao, 2011. A novel attribute weighting algorithm for clustering high-dimensional categorical data. Pattern Recogn., 44(12): 2843-2861.
CrossRef -
Cao, F., J. Liang, D. Li, L. Bai and C. Dang, 2012. A dissimilarity measure for the k-Modes clustering algorithm. Knowl-Based Syst., 26: 120-127.
CrossRef -
Cao, F., J. Liang, D. Li and X. Zhao, 2013. A weighting k-modes algorithm for subspace clustering of categorical data. Neurocomputing, 108: 23-30.
CrossRef -
Chatzis, S.P., 2011. A fuzzy c-means-type algorithm for clustering of data with mixed numeric and categorical attributes employing a probabilistic dissimilarity functional. Expert Syst. Appl., 38(7): 8684-8689.
CrossRef -
Core Team, R., 2013. R: A language and environment for statistical computing. R Foundation for Statistical Computing, Vienna, Austria, ISBN 3-900051-07-0.
-
Han, J., M. Kamber and J. Pei, 2006. Data Mining: Concepts and Techniques. Morgan Kaufmann Publishers Inc., San Francisco, CA, USA.
-
He, Z., X. Xu and S. Deng, 2011. Attribute value weighting in k-modes clustering. Expert Syst. Appl., 38(12): 15365-15369.
CrossRef -
Hubert, L. and A. Phipps, 1985. Comparing partitions. J. Classif., 2: 193-218.
CrossRef -
Ji, J., T. Bai, C. Zhou, C. Ma and Z. Wang, 2013. An improved k-prototypes clustering algorithm for mixed numeric and categorical data. Neurocomputing, 120: 590-596.
CrossRef -
Ji, J., W. Pang, C. Zhou, X. Han and Z. Wang, 2012. A fuzzy k-prototype clustering algorithm for mixed numeric and categorical data. Knowl-Based Syst., 30: 129-135.
CrossRef -
Li, Y., C. Luo and S.M. Chung, 2008. Text clustering with feature selection by using statistical data. IEEE T. Knowl. Data En., 20(5): 641-652.
CrossRef -
NIST, 2012. NIST/SEMATECH e-handbook of Statistical Methods. Retrieved form: http://www.itl.nist.gov/div898/handbook/2012.
Direct Link -
Rajalakshmi, R., 2014. Supervised term weighting methods for URL classification. J. Comput. Sci., 10: 1969-1976.
CrossRef -
Ralambondrainy, H., 1995. A conceptual version of the K-means algorithm. Pattern Recogn. Lett., 16(11): 1147-1157.
CrossRef -
Santos, J.M. and M. Embrechts, 2009. On the use of the adjusted rand index as a metric for evaluating supervised classification. In: Alippi, C. et al. (Eds.), ICANN, 2009. Part 2 LNCS 5769, Springer, Berlin, Heidelberg, pp: 175-184.
CrossRef -
UCI ML Dataset, year. UCI Machine Learning Repository. Retrieved form: http://archive.ics.uci.edu/ml. University of California, School of Information and Computer Sciences, Irvine.
-
Vendramin, L., Campello, R.J.G.B. Ricardo and E.R. Hruschka, 2009. On the comparison of relative clustering validity criteria. Proceeding of the 9th SIAM, International Conference on Data Mining (SDM). Sparks, NV, pp: 733-744.
Competing interests
The authors have no competing interests.
Open Access Policy
This article is distributed under the terms of the Creative Commons Attribution 4.0 International License (http://creativecommons.org/licenses/by/4.0/), which permits unrestricted use, distribution, and reproduction in any medium, provided you give appropriate credit to the original author(s) and the source, provide a link to the Creative Commons license, and indicate if changes were made.
Copyright
The authors have no competing interests.
|
|
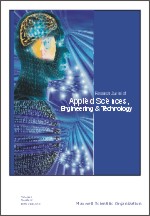 |
ISSN (Online): 2040-7467
ISSN (Print): 2040-7459 |
 |
Information |
|
|
|
Sales & Services |
|
|
|