Research Article | OPEN ACCESS
Inspecting the Proficiency of Novel Algorithms on Sparse Data Domains for Efficient Recommendation-A Glance
Chandni Suresh, B. Krishya Yedugiri, P. Sini Raj and Souparnika Sreedhar
Department of Computer Science and Engineering, Amrita School of Engineering, Amrita Vishwa Vidyapeetham (Amrita University), Ettimadai, India
Research Journal of Applied Sciences, Engineering and Technology 2015 7:812-815
Received: February ‎16, ‎2015 | Accepted: March ‎7, ‎2015 | Published: July 10, 2015
Abstract
Recommender systems have played a major role in almost all the domains today where human interaction happens with system. Depending on the user’s choice, a recommender system presents some promising suggestions by observing all the activities of the user on the web and thus, helps to find out similar users and interested products. All the ratings provided by the user is stored in a rating matrix. Sometimes it so happens that the users may view the item, but not always rate it; which makes the dataset sparse. Performing operations on such sparse datasets by recommender engines may not give precise suggestions to the user. This study aims to make such sparse datasets denser by applying the two novel methods, FST and UTAOS; and thereby implementing any of the collaborative filtering techniques upon it to showcase the efficiency in recommendation. Results reveal that FST outperforms the UTAOS approach in terms of sparse datasets which paves the way for an efficient recommendation.
Keywords:
Feature subspace transfer, recommender engine, sparse datasets , user,
Competing interests
The authors have no competing interests.
Open Access Policy
This article is distributed under the terms of the Creative Commons Attribution 4.0 International License (http://creativecommons.org/licenses/by/4.0/), which permits unrestricted use, distribution, and reproduction in any medium, provided you give appropriate credit to the original author(s) and the source, provide a link to the Creative Commons license, and indicate if changes were made.
Copyright
The authors have no competing interests.
|
|
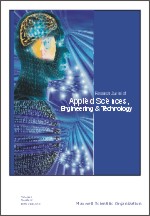 |
ISSN (Online): 2040-7467
ISSN (Print): 2040-7459 |
 |
Information |
|
|
|
Sales & Services |
|
|
|