Research Article | OPEN ACCESS
Restricted Bipartite Graphs Based Target Detection for Hyperspectral Image Classification with GFA-LFDA Multi Feature Selection
1T. Karthikeyan and 2S. Venkatesh Kumar
1P.S.G. College of Arts and Science
2Department of MCA, Dr. SNS Rajalakshmi College of Arts and Science, Coimbatore, India
Research Journal of Applied Sciences, Engineering and Technology 2015 5:504-513
Received: August ‎22, ‎2014 | Accepted: ‎September ‎13, ‎2014 | Published: June 15, 2015
Abstract
Hyper spectral imaging has recently become one of the most active research areas in remote sensing. Hyper spectral imagery possesses more spectral information than multispectral imagery because the number of spectral bands in hyper spectral imagery is in the hundreds rather than in the tens. However, the high dimensions of hyper spectral images cause redundancy in spatial-spectral feature domain and consider only spectral and spatial features only and ability of the classifier to excel even as training HSI images are limited. However, unless develop suitable algorithms for target detection or classification of the hyper spectral images data becomes difficult. Therefore, it is becomes essential to consider different features and find exact target detection rate to improve classification rate. In order to overcome this problem in this study presents a novel classification framework for hyper spectral data. Proposed system uses a graph based representation, Restricted Bipartite Graphs (RBG) for exact detection of the class values. Before that the feature of the HSI images are selected using the Gaussian Firefly Algorithm (GFA) for multiple feature selection and Local-Fisher’s Discriminant Analysis (LFDA) based feature projection are performed in a raw spectral-spatial feature space for effective dimensionality reduction. Then RBG is proposed to represent the reduced feature results into graphical manner to solve exact target class matching problem, in hyper spectral imaginary. Classification is performed using the Hybrid Genetic Fuzzy Neural Network (HGFNN), Genetic algorithm is used to optimize the weights of the fuzzifier and the defuzzifier for labeled and unlabeled data samples. Experimentation results show that the proposed GFA-LFDA-RBG-HGFNN method outperforms in terms of the classification accuracy and less misclassification results than traditional methods.
Keywords:
Gaussian Firefly Algorithm (GFA), Hybrid Genetic Fuzzy Neural Network (HGFNN), Hyper Spectral Imagery (HIS), Local-Fisher, Restricted Bipartite Graphs (RBG), Spatial Gray Level Dependency (SGLD),
Competing interests
The authors have no competing interests.
Open Access Policy
This article is distributed under the terms of the Creative Commons Attribution 4.0 International License (http://creativecommons.org/licenses/by/4.0/), which permits unrestricted use, distribution, and reproduction in any medium, provided you give appropriate credit to the original author(s) and the source, provide a link to the Creative Commons license, and indicate if changes were made.
Copyright
The authors have no competing interests.
|
|
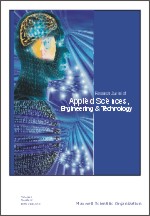 |
ISSN (Online): 2040-7467
ISSN (Print): 2040-7459 |
 |
Information |
|
|
|
Sales & Services |
|
|
|