Research Article | OPEN ACCESS
Alzheimer's Disease Classification Using Hybrid Neuro Fuzzy Runge-Kutta (HNFRK) Classifier
1R. Sampath and 2A. Saradha
1Anna University, Chennai, India
2Department of Computer Science Engineering, Institute of Road Transport and Technology, Erode, Tamil Nadu, India
Research Journal of Applied Sciences, Engineering and Technology 2015 1:29-34
Received: November ‎10, ‎2014 | Accepted: January ‎21, ‎2015 | Published: May 10, 2015
Abstract
Alzheimer’s Disease (AD) exists more prior to the over appearance of clinical symptoms and is characterized by brain changes. In this study, Functional Magnetic Resonance Imaging (FMRI) offers considerable promise as a tool for detecting brain changes in Alzheimer disease pretentious patients. Therefore, FMRI may offer the unique ability to detention of the dynamic state of change in the collapsing brain. Improve the accuracy of brain FMRI image segmentation, a robust Spatial Fuzzy C-Means (SFCM) is utilized and a combination of Adaptive Neuro Fuzzy Inference System and Runge-Kutta Learning Algorithm called Hybrid Neuro Fuzzy Runge-Kutta (HNFRK) classifier is used for prediction of Alzheimer’s Disease (AD). The performance of the proposed classifier is compared with SVM and ANFIS classifier. The results show that the sensitivity and specificity of HNFRK classifier is more compared to the SVM and ANFIS. The sensitivity and specificity of HNFRK is above 90% which is below 90% in case of SVM and ANFIS classifier. Thus it can be shown that HNFRK performs accurate classification than SVM and ANFIS.
Keywords:
Alzheimer, ANFIS (Adaptive Neuro Fuzzy Inference System), FMRI images, Runge- Kutta Learning algorithm (RKLM), Spatial Fuzzy C-Means (SFCM),
References
-
Bassett, S.S., D.M. Yousem, C. Cristinzio, I. Kusevic, M.A. Yassa, B.S. Caffo and S.L. Zeger, 2006. Familial risk for Alzheimer’s disease alters FMRI activation patterns. Brain, 129(5): 1229-1239.
CrossRef PMid:16627465 PMCid:PMC2744898 -
Beckett, L.A., D.J. Harvey, A. Gamst, M. Donohue, J. Kornak, H. Zhang and J.H. Kuo, 2010. The Alzheimer's disease neuroimaging initiative: Annual change in biomarkers and clinical outcomes. Alzheimer's Dement., 6(3): 257-264.
CrossRef PMid:20451874 PMCid:PMC2867839 -
Belmokhtar, N. and N. Benamrane, 2012. Classification of Alzheimer's disease from 3D structural MRI data. Int. J. Comput. Appl., 47(3): 40-44.
-
Boyacioglu, M.A. and D. Avci, 2010. An adaptive network-based fuzzy inference system (ANFIS) for the prediction of stock market return: The case of the Istanbul stock exchange. Expert Syst. Appl., 37(12): 7908-7912.
CrossRef -
Dinesh, E., M.S. Kumar, M. Vigneshwar and T. Mohanraj, 2013. Instinctive classification of Alzheimer's disease using FMRI, PET and SPECT images. Proceeding of 7th International Conference on Intelligent Systems and Control (ISCO), pp: 405-409.
CrossRef -
Dyrba, M., M. Ewers, M. Wegrzyn, I. Kilimann, C. Plant, A. Oswald et al., 2013. Robust automated detection of microstructural white matter degeneration in Alzheimer's disease using machine learning classification of multicenter DTI data. PLoS One, 8(5): e64925.
CrossRef PMid:23741425 PMCid:PMC3669206 -
Hua, X., S. Lee, I. Yanovsky, A.D. Leow, Y.Y. Chou, A.J. Ho et al., 2009. Optimizing power to track brain degeneration in Alzheimer's disease and mild cognitive impairment with tensor-based morphometry: An ADNI study of 515 subjects. NeuroImage, 48(4): 668-681.
CrossRef PMid:19615450 PMCid:PMC2971697 -
Joshi, S., D. Shenoy, G.G. Vibhudendra Simha, P.L. Rrashmi, K.R. Venugopal et al., 2010. Classification of Alzheimer's disease and parkinson's disease by using machine learning and neural network methods. Proceedings of the 2nd International Conference on Machine Learning and Computing, pp: 218-222.
CrossRef -
Liu, M., D. Zhanga and D. Shen, 2012. Ensemble sparse classification of Alzheimer's disease. NeuroImage, 60(2): 1106-1116.
CrossRef PMid:22270352 PMCid:PMC3303950 -
Liu, Y., T. Paajanen, Y. Zhang, E. Westman, L.O. Wahlund et al., 2009. Combination analysis of neuropsychological tests and structural MRI measures in differentiating AD, MCI and control groups--The AddNeuroMed study. Neurobiol. Aging, 32(7): 1198-206.
CrossRef PMid:19683363 -
Matsuda, H., 2007. Role of neuroimaging in Alzheimer’s disease, with emphasis on brain perfusion SPECT. J. Nucl. Med., 48(8): 1289-1300.
CrossRef PMid:17631544 -
McGeown, W.J., M.F. Shanks, K.E. Forbes-McKay and A. Venneri, 2009. Patterns of brain activity during a semantic task differentiate normal aging from early Alzheimer's disease. Psychiat. Res., 173(3): 218-227.
CrossRef PMid:19683419 -
Meena, A. and K. Raja, 2013. Spatial fuzzy C-means PET image segmentation of neurodegenerative disorder. Indian J. Comput. Sci. Eng., 4(1): 50-55.
-
Musa, H., I. Saidu and M.Y. Wazir, 2010. A simplified derivation and analysis of fourth order runge kutta method. Int. J. Comput. Appl., 9(8): 51-55.
CrossRef -
Nixon, M.S. and A.S. Aguado, 2012. Feature Extraction and Image Processing for Computer Vision. Academic Press, Oxford.
-
Padmanabhan, V. and M. Prabakaran, 2014. Object color graph based color image classification using intensity distributional matrix. Int. J. Invent. Comput. Sci. Eng., 1(5).
-
Ramakrishnan, M. and C. Nalini, 2014. A novel computer based statistics for identifying the risk assessment to the patients in the case of congestive heart failure. Int. J. Invent. Comput. Sci. Eng., 1(2).
-
Ramesh Kumar, K.K. and A. Anbumani, 2014. Medical image segmentation using multifractual analysis. Int. J. Invent. Comput. Sci. Eng., 1(3).
-
Shin, J., S.Y. Lee, S.J. Kim, S.H. Kim, S.J. Cho and Y.B. Kim, 2010. Voxel-based analysis of Alzheimer's disease PET imaging using a triplet of radiotracers: PIB, FDDNP and FDG. NeuroImage, 52(2): 488-496.
CrossRef PMid:20385246 -
Tripoliti, E.E., D.I. Fotiadis and M. Argyropoulou, 2008. An automated supervised method for the diagnosis of Alzheimer’s disease based on FMRI data using weighted voting schemes. Proceeding of IEEE International Workshop on Imaging Systems and Techniques (IST, 2008), pp: 340-345.
-
Tripoliti, E.E., D.I. Fotiadis, M. Argyropoulou and G. Manis, 2010. A six stage approach for the diagnosis of the Alzheimer's disease based on FMRI data. J. Biomed. Inform., 43(2): 307-320.
CrossRef PMid:19883796 -
Zhang, Y., S. Wang and Z. Dong, 2014. Classification of Alzheimer disease based on structural magnetic resonance imaging by kernel support vector machine decision tree. Prog. Electromagn. Res., 144: 171-184.
CrossRef
Competing interests
The authors have no competing interests.
Open Access Policy
This article is distributed under the terms of the Creative Commons Attribution 4.0 International License (http://creativecommons.org/licenses/by/4.0/), which permits unrestricted use, distribution, and reproduction in any medium, provided you give appropriate credit to the original author(s) and the source, provide a link to the Creative Commons license, and indicate if changes were made.
Copyright
The authors have no competing interests.
|
|
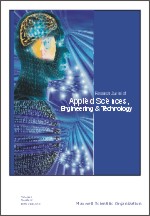 |
ISSN (Online): 2040-7467
ISSN (Print): 2040-7459 |
 |
Information |
|
|
|
Sales & Services |
|
|
|