Research Article | OPEN ACCESS
A Novel Hybrid System for Diagnosing Breast Cancer Using Fuzzy Rough Set and LS-SVM
1R. Jaya Suji and 2S.P. Rajagopalan
1Sathyabama University
2GKM College of Engineering and Technology, Chennai, India
Research Journal of Applied Sciences, Engineering and Technology 2015 1:49-55
Received: November 30, 2014 | Accepted: January 11, 2015 | Published: May 10, 2015
Abstract
With fast development of medical diagnosis technologies, the filtering of the entire relevant feature and time consuming task are challenging tasks. For effective feature selection and reducing the time consuming, we propose a new hybrid system for diagnosing the breast cancer. The proposed hybrid system is the combination of CFRSFS, K-Means Clustering and Least Square Support Vector Machine (LS-SVM). In this hybrid system, we propose a new feature selection algorithm called Correlation based Fuzzy Rough Set Feature Selection (CFRSFS) algorithm for effective initial feature selection process. Moreover, K-Means clustering algorithm has been used for enhancing the feature selection process based on the factors of the selected features in similar manner of the existing hybrid system. Finally, LS-SVM algorithm is also used for classifying the feature selected breast cancer dataset. The experiments have been conducted for evaluating the proposed system using WDBC Data set. The obtained results show that the performance of the proposed system classification accuracy is 99.54%.
Keywords:
Cancer diagnosis, data mining, k-means clustering, least square support vector machine,
References
-
Chen, C.H., 2014. A hybrid intelligent model of analyzing clinical breast cancer data using clustering techniques with feature selection. Appl. Soft Comput., 20: 4-14.
CrossRef -
Chen, M.S., J. Han and P.S. Yu, 1996. Data mining: An overview from a database perspective. IEEE T. Knowl. Data En., 8: 866-883.
CrossRef -
Cortes, C. and V. Vapnik, 1995. Support-vector networks. Mach. Learn., 20: 273-297.
-
Dubois, D. and H. Prade, 1990. Rough fuzzy sets and fuzzy rough sets. Int. J. Gen. Syst., 17: 191-208.
CrossRef -
Fayyad, U., G. Piatetsky-Shapiro and P. Smyth, 1996. From data mining to knowledge discovery in databases. Artif. Intell. Mag., 17: 37-54.
-
Ferreira, A.J. and M.A.T. Figueiredo, 2012. An unsupervised approach to feature discretization and selection. Pattern Recogn., 45: 3048-3060.
CrossRef -
Ganapathy, S., P. Yogesh and A. Kannan, 2012b. Intelligent agent based intrusion detection system using enhanced multiclass SVM. Int. J. Comput. Intell. Neurosci., 20(12): 195-202.
CrossRef -
Ganapathy, S., K. Kulothungan, P. Yogesh and A. Kannan 2012a. A novel weighted fuzzy c-means clustering based on immune genetic algorithm for intrusion detection. Proc. Eng., 38: 1750-1757.
CrossRef' target='_blank'>CrossRef -
Ganapathy, S., K. Kulothungan, S. Muthurajkumar, M. Vijayalakshmi, P. Yogesh and A. Kannan, 2013. Intelligent feature selection and classification techniques for intrusion detection in networks: A survey. EURASIP J. Wirel. Comm., 271: 1-16.
CrossRef -
Hall, M., 1998. Correlation-based feature selection for machine learning. Ph.D. Thesis, Department of Computer Science, Waikato University, Hamilton, NZ.
-
Jain, A.K., M.N. Murty and P.J. Flynn, 1999. Data clustering: A review. ACM Comput. Surv., 31: 264-323.
CrossRef -
Jaisankar, N., S. Ganapathy and A. Kannan, 2012. Intelligent intrusion detection system using fuzzy rough set based C4.5 algorithm. Proceeding of International ACM Conference on Advances in Computing, Communications and Informatics (ICACCI-2012), pp: 596-601.
CrossRef -
Jensen, R. and Q. Shen, 2004. Fuzzy-rough attributes reduction with application to web categorization. Fuzzy Set. Syst., 141(3): 469-485.
CrossRef -
Li, M., S. Deng, L. Wang, S. Feng and J. Fan, 2014. Hierarchical clustering algorithm for categorical data using a probabilistic rough set model. Knowl-Based Syst., 65: 60-71.
CrossRef -
Polat, K. and S. Günes, 2007. Breast cancer diagnosis using least square support vector machine. Digit. Signal Process., 17: 694-701.
CrossRef -
Prasad, Y., K. Biswas and C. Jain, 2010. SVM classifier based feature selection using GA, ACO and PSO for siRNA design. Proceeding of the 1st International Conference on Advances in Swarm Intelligence, pp: 307-314.
CrossRef PMid:21054159 -
Siegel, R., D. Naishadham and A. Jemal, 2012. Cancer statistics. Cancer J. Clin., 62: 10-29.
CrossRef PMid:22237781 -
Venkatadri, M. and C.R. Lokanatha, 2011. A review on data mining from past to the future. Int. J. Comput. Appl., 15: 19-22.
-
Wolberg, W.H., W.N. Street and O.L. Mangasarian, 1995. Image analysis and machine learning applied to breast cancer diagnosis and proganosis. Anal. Quant. Cytol., 17(2): 77-87.
-
Xu, R. and D. Wunsch, 2005. Survey of clustering algorithms. IEEE T. Neural Networ., 16: 645-678.
CrossRef PMid:15940994 -
Zadeh, L.A., 1965. Fuzzy sets. Inform. Control, 8: 338-353.
CrossRef -
Zheng, B., S.W. Yoon and S.S. Lam, 2014. Breast cancer diagnosis based on feature extraction using a hybrid of K-means and support vector machine algorithms. Expert Syst. Appl., 41: 1476-1482.
CrossRef
Competing interests
The authors have no competing interests.
Open Access Policy
This article is distributed under the terms of the Creative Commons Attribution 4.0 International License (http://creativecommons.org/licenses/by/4.0/), which permits unrestricted use, distribution, and reproduction in any medium, provided you give appropriate credit to the original author(s) and the source, provide a link to the Creative Commons license, and indicate if changes were made.
Copyright
The authors have no competing interests.
|
|
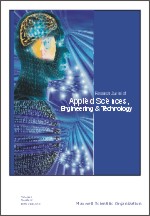 |
ISSN (Online): 2040-7467
ISSN (Print): 2040-7459 |
 |
Information |
|
|
|
Sales & Services |
|
|
|