Research Article | OPEN ACCESS
Testing Homogeneity of Mixture of Skew-normal Distributions Via Markov Chain Monte Carlo Simulation
1Rahman Farnoosh 2Morteza Ebrahimi and 2SetarehDalirian
1Department of Mathematics, South Tehran Branch, Islamic Azad University, Tehran
2Department of Mathematics, Karaj Branch, Islamic Azad University, Karaj
3School of Mathematics, Iran University of Science and Technology, Narmak, Tehran 16846, Iran
Research Journal of Applied Sciences, Engineering and Technology 2015 2:112-117
Received: May 06, 2012 | Accepted: July 09, 2012 | Published: May 20, 2015
Abstract
The main purpose of this study is to intoduce an optimal penalty function for testing homogeneity of finite mixture of skew-normal distribution based on Markov Chain Monte Carlo (MCMC) simulation. In the present study the penalty function is considered as a parametric function in term of parameter of mixture models and a Baysian approach is employed to estimating the parameters of model. In order to examine the efficiency of the present study in comparison with the previous approaches, some simulation studies are presented.
Keywords:
Homogeneity test, markov chain monte carlo simulation, penalty function, skew-normal mixtures,
References
-
Azzalini, A., 1985. A class of distributions which includes the normal ones. Scand. J. Stat., 12: 171-178.
-
Azzalini, A. and A. Capitanio, 2003. Distributions generated by perturbation of symmetry with emphasis on a multivariate skew t-distribution. J. Roy. Stat. Soc. B, 65: 367-389.
CrossRef -
Basso, R.M., V.H. Lachos, C.R. Cabral and P. Ghosh, 2010. Robust mixture modeling based on scale mixtures of skew-normal distributions. Comput. Stat. Data An., 54(12): 2926-2941.
CrossRef -
Bohning, D., 2000. Computer-assisted Analysis of Mixtures and Applications. Chapman and Hall/CRC, Boca Raton.
-
Branco, M.D. and D.K. Dey, 2001. A general class of multivariate skew-elliptical distributions. J. Multivariate Anal., 79: 99-113.
CrossRef -
Cabral, C.R.B., H. Bolfarine and J.R.G., Pereira, 2008. Bayesian density estimation using skew student-t-normal mixtures. Comput. Stat. Data An., 52: 5075-5090.
CrossRef -
Chen, H. and J. Chen, 2001. The likelihood ratio test for homogeneity in the finite mixture models. Can. J. Stat., 29: 201-215.
CrossRef -
Chen, H., J. Chen and J.D.K. Kalbfleisch, 2004. Testing for a finite mixture model with two components. J. Roy. Stat. Soc. B, 66: 95-115.
CrossRef -
Chen, J., 1998. Penalized likelihood ratio test for finite mixture models with multinomial observations. Can. J. Stat., 26: 583-599.
CrossRef -
Dempster, A., N. Laird and D. Rubin, 1977. Maximum likelihood from incomplete data via the EM algorithm. J. Roy. Stat. Soc. B, 39: 1-38.
-
Diebolt, J. and C.P. Robert, 1994. Estimation of finite mixture distributions through Bayesian sampling. J. Roy. Stat. Soc. B, 56: 363-375.
CrossRef -
Escobar, M.D. and M. West, 1995. Bayesian density estimation and inference using mixtures. J. Am. Stat. Assoc., 90: 577-588.
CrossRef -
Farnoosh, R., M. Ebrahimi and A. Hajirajabi, 2012. Optimal penalty functions based on MCMC for testing homogeneity of mixture models. Res. J. Appl. Sci. Eng. Technol., 4(14): 2024-2029.
-
Henze, N., 1986. A probabilistic representation of the skew-normal distribution. Scand. J. Stat., 13: 271-275.
-
Lachos, V.H., P. Ghosh and R.B. Arellano-Valle, 2010. Likelihood based inference for skew normal independent linear mixed models. Stat. Sinica, 20: 303-322.
-
Li, P., J. Chen and P. Marrott, 2008. Non-finite Fisher information and homo-geneitty: The EM approach. Biometrika., 96: 411-426.
CrossRef -
Lin, T.I., J.C. Lee and W.J. Hsieh, 2007a. Robust mixture modelling using the skew t distribution. Stat. Comput., 17: 81-92.
CrossRef -
Lin, T.I., J.C. Lee and S.Y. Yen, 2007b. Finite mixture modelling using the skew normal distribution. Stat. Sinica, 17: 909-927.
-
Lin, T.I., 2009. Maximum likelihood estimation for multivariate skew normal mixture models. J. Multivariate Anal., 100: 257-265.
CrossRef -
Liu, H.B. and X.M. Shao, 2003. Reconstruction of January to April mean temperature in the qinling mountain from 1789 to 1992 using tree ring chronologies. J. Appl. Meteorol. Sci., 14: 188-196.
-
Marin, J.M., K.L. Mengerson and C. Robert, 2005. Bayesian Modelling and Inference on Mixtures of Distributions. In: Dey, D. and C.R. Rao (Eds.), Handbook of Statistics Elsevier, 25: 459-507.
CrossRef -
McLachlan, G.J. and D. Peel, 2000. Finite Mixture Models. Wiely, New York.
CrossRef -
Richardson, S. and P.J. Green, 1997. On Bayesian analysis of mixtures with an unknown number of components. J. Roy. Stat. Soc. B, 59: 731-792.
CrossRef -
Stephens, M., 2000. Bayesian analysis of mixture models with an unknown number of components-an alternative to reversible jump methods. Ann. Stat., 28: 40-74.
CrossRef
Competing interests
The authors have no competing interests.
Open Access Policy
This article is distributed under the terms of the Creative Commons Attribution 4.0 International License (http://creativecommons.org/licenses/by/4.0/), which permits unrestricted use, distribution, and reproduction in any medium, provided you give appropriate credit to the original author(s) and the source, provide a link to the Creative Commons license, and indicate if changes were made.
Copyright
The authors have no competing interests.
|
|
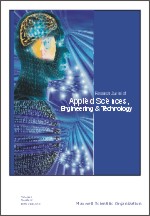 |
ISSN (Online): 2040-7467
ISSN (Print): 2040-7459 |
 |
Information |
|
|
|
Sales & Services |
|
|
|