Research Article | OPEN ACCESS
Optimizing Cash Management Model Using Computational Intelligence
1, 2A. Alli and 1M.M. Ramya
1Department of Computer Applications, Hindustan University, Chennai, Tamilnadu
2Department of Computer Applications, Presidency College, Bangalore, Karnataka, India
Research Journal of Applied Sciences, Engineering and Technology 2015 2:221-228
Received: May ‎16, ‎2015 | Accepted: June ‎22, ‎2015 | Published: September 15, 2015
Abstract
In today’s technical era, the financial organizations have great challenges to optimize the cash management process. Maintaining minimum cash leads to customer frustration. At the same time, upholding excess cash is a loss to the organization. Hence, soft computing based cash management solutions are required to maintain optimal cash balance. An Artificial Neural Network (ANN) is one such technique which plays a vital role in the fields of cognitive science and engineering. In this study, a novel ANN-based cash Forecasting Model (ANNCFM) has been proposed to identify the cash requirement on daily, weekly and monthly basis. The six cash requirement parameters: Reference Year (RY), Month of the Year (MOY), Working Day of the Month (WDOM), Working Day of the Week (WDOW), Salary Day Effect (SDE) and Holiday Effect (HDE) were fed as input to ANNCFM. Trials were carried out for the selection of ANNCFM network parameters. It was found that number of hidden neurons, learning rate and the momentum when set to 10, 0.3 and 0.95, respectively yielded better results. Mean Absolute Percentage Error (MAPE) and Mean Squared Error (MSE) were used to evaluate the performance of the proposed model. MSE that was less than 0.01 proves the capability of the proposed ANNCFM in estimating the cash requirement.
Keywords:
ANN, ANNCFM, back-propagation, cash forecasting,
References
-
Alli, A., M. Ramya, M. Srinivasa and V. Kumar, 2013. Cash management using particle swarm optimization. Proceeding of the International Conference on Data Mining and Soft Computing. SASTRA University, Thanjavur, India.
- Aminian, F., E.D. Suarez, M. Aminian and D.T. Walz, 2006. Forecasting economic data with neural networks. Comput. Econ., 28: 71-88.
CrossRef
- Bishop, C.B., 1999. Neural Networks for Pattern Recognition. Oxford University Press, New York.
- Fraydoon, R.R., H. Farshad and M. Seyed Reza, 2010. A comparison of classic time series models and artificial neural networks in anticipation of cash requirements of banks: A case study in Iran. Proceeding of the International Conference on Academic and Business Research Institute, Orlando, USA.
- Geem, Z.W. and W.E. Roper, 2009. Energy demand estimation of South Korea using artificial neural network. Energ. Policy, 37(10): 4049-4054.
CrossRef
- Gorr, W., D. Nagin and J. Szczypula, 1994. Comparative study of artificial neural network and statistical models for predicting student point averages. Int. J. Forecasting, 10(1): 17-34.
CrossRef
-
Hanna, A.M., D. Ural and G. Saygili, 2007. Evaluation of liquefaction potential of soil deposits using artificial neural networks. Eng. Computation, 24: 5-16.
CrossRef
-
Haykin, S., 1994. Neural Networks: A Comprehensive Foundation. Macmillan College Publishing Co., Inc., New York.
-
Julian, D.O., K.J. Michael and G.D. Russell, 2004. An accurate comparison of methods for quantifying variable importance in artificial neural network using simulated data. Ecol. Modell., 178(3-4): 389-397.
CrossRef
- Kumar, P.C. and E. Walia, 2006. Cash forecasting: An application of artificial neural networks in finance. Int. J. Comput. Sci. Appl., 3: 61-77.
-
Leigh, W., R. Hightower and N. Modani, 2005. Forecasting the New York stock exchange composite index with past price and interest rate on condition of volume spike. Expert Syst. Appl., 28(1): 1-8.
CrossRef
- Mabel, M.C. and E. Fernandez, 2008. Analysis of wind power generation and prediction using ANN: A case study. Renew. Energ., 33(5): 986-992.
CrossRef
-
Manfred, S. and W. Hans-Georg, 1997. Portfolio optimization with a neural network implementation of the coherent market hypothesis. Eur. J. Oper. Res., 100(1): 27-40.
CrossRef
-
Nakamura, E., 2006. Inflation forecasting using a neural network. Econ. Lett., 86: 373-378.
CrossRef
-
Refenes, A.P. and H. White, 1998. Neural networks and financial economics. Int. J. Forecasting, 6(17).
-
Sharda, R. and D. Delen, 2006. Predicting box-office success of motion pictures with neural networks. Expert Syst. Appl., 30(2): 243-254.
CrossRef
-
Sola, J. and J. Sevilla, 1997. Importance of data normalization for the application of neural networks to complex industrial problems. IEEE T. Nucl. Sci., 44(3): 1464-1468.
CrossRef
-
Taubenböck, H., T. Esch, M. Wurm, A. Roth and S. Dech, 2010. Object-based feature extraction using high spatial resolution satellite data of urban areas. J. Spat. Sci., 55(1): 117-132.
CrossRef
- Tenti, P., 1996. Forecasting foreign exchange rates using recurrent neural networks. Appl. Artif. Intell., 10: 567-581.
CrossRef
- Yokoyama, R., T. Wakui and R. Satake, 2009. Prediction of energy demands using neural network with model identification by global optimization. Energ. Convers. Manage., 50(2): 319-327.
CrossRef
- Zhang, G., B.E. Patuwo and M.Y. Hu, 1998. Forecasting with artificial neural networks: The state of the art. Int. J. Forecasting, 14: 35-62.
CrossRef
Competing interests
The authors have no competing interests.
Open Access Policy
This article is distributed under the terms of the Creative Commons Attribution 4.0 International License (http://creativecommons.org/licenses/by/4.0/), which permits unrestricted use, distribution, and reproduction in any medium, provided you give appropriate credit to the original author(s) and the source, provide a link to the Creative Commons license, and indicate if changes were made.
Copyright
The authors have no competing interests.
|
|
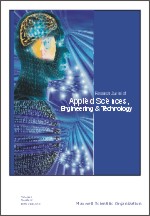 |
ISSN (Online): 2040-7467
ISSN (Print): 2040-7459 |
 |
Information |
|
|
|
Sales & Services |
|
|
|