Research Article | OPEN ACCESS
Service Composition Optimization Using Differential Evolution and Opposition-based Learning
M.A. Remli, S. Deris, M. Jamous, M.S. Mohamad and A. Abdullah
Department of Software Engineering, Faculty of Computing, Universiti Teknologi Malaysia, 81310 Johor Bahru, Johor, Malaysia
Research Journal of Applied Sciences, Engineering and Technology 2015 2:229-234
Received: May 20, 2015 | Accepted: June 19, 2015 | Published: September 15, 2015
Abstract
The numbers of web services are increasing rapidly over the last decades. One of the most interesting challenges in using web services is the usage of service composition that allows users to select and invoke composite services. In addition, the characteristic of each service is distinguished based on the quality of service (QoS). QoS is utilized in optimizing decisive factors such as cost or response time that is required by the user in the runtime system. Thus, QoS and service composition problem can be modeled as an optimization problem. In this study, differential evolution and opposition-based learning optimization methods have been proposed to obtain the optimal solution from candidate services. The results show that the proposed method converges faster than others. Therefore, the method is capable to select better composite services in short time.
Keywords:
Differential evolution, opposition-based learning, quality of services, service optimization, web service composition,
References
-
Alrifai, M. and T. Risse, 2010. Efficient QoS-aware service composition. Ws. So. Ag. Te., 3: 75-87.
CrossRef - Canfora, G., M.D. Penta, R. Esposito and M.L. Villani, 2005. An approach for QoS-aware service composition based on genetic algorithms. Proceedings of the 7th Annual Conference on Genetic and Evolutionary Computation (GECCO'05), pp: 1069-1075.
CrossRef
- Das, S. and P.N. Suganthan, 2010. Differential evolution: A survey of the state-of-the-art. IEEE T. Evolut. Comput., 15(1): 4-31.
CrossRef
-
Jula, A., E. Sundararajan and Z. Othman, 2014. Cloud computing service composition: A systematic literature review. Expert Syst. Appl., 41(8): 3809-3824.
CrossRef
-
Mardukhi, F., N. NematBakhsh, K. Zamanifar and A. Barati, 2013. QoS decomposition for service composition using genetic algorithm. Appl. Soft Comput., 13(7): 3409-3421.
CrossRef
- Ngoko, Y., A. Goldman and D. Milojicic, 2013. Service selection in web service compositions optimizing energy consumption and service response time. J. Internet Serv. Appl., 4: 19.
CrossRef
- Pop, F.C., D. Pallez, M. Cremene, A. Tettamanzi, M. Suciu and M. Vaida, 2011a. QoS-based service optimization using differential evolution. Proceeding of the 13th Annual Conference on Genetic and Evolutionary Computation (GECCO’11), pp: 1891-1898.
CrossRef
- Pop, F.C., M. Cremene, M.F. Vaida and A. Serbanescu, 2011b. Medical services optimization using differential evolution. Proceeding of International Conference on Advancements of Medicine and Health Care through Technology, 36: 72-77.
-
Rahnamayan, S., H.R. Tizhoosh and M.M.A. Salama, 2008. Opposition-based differential evolution. IEEE T. Evolut. Comput., 12(1): 64-79.
CrossRef
- Siadat, S.H., A.M. Ferreira and P. Milano, 2013. Performance analysis of QoS-based web service selection through integer programming, World Appl. Sci. J., 28(4): 463-472.
-
Storn, R. and K. Price, 1997. Differential evolution: A simple and efficient heuristic for global optimization over continuous spaces. J. Global Optim., 11: 341-359.
CrossRef
- Xu, Q., L. Wang, N. Wang, X. Hei and L. Zhao, 2014. A review of opposition-based learning from 2005 to 2012. Eng. Appl. Artif. Intel., 29: 1-12.
CrossRef
Competing interests
The authors have no competing interests.
Open Access Policy
This article is distributed under the terms of the Creative Commons Attribution 4.0 International License (http://creativecommons.org/licenses/by/4.0/), which permits unrestricted use, distribution, and reproduction in any medium, provided you give appropriate credit to the original author(s) and the source, provide a link to the Creative Commons license, and indicate if changes were made.
Copyright
The authors have no competing interests.
|
|
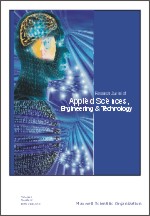 |
ISSN (Online): 2040-7467
ISSN (Print): 2040-7459 |
 |
Information |
|
|
|
Sales & Services |
|
|
|