Research Article | OPEN ACCESS
An Intelligent Type-II Diabetes Mellitus Diagnosis Approach using Improved FP-growth with Hybrid Classifier Based Arm
1T. Karthikeyan, 1K. Vembandasamy and 2B. Raghavan
1PSG College of Arts and Science, Coimbatore, India
2Department of Biochemistry, PSG College of Arts and Science, Coimbatore, India
Research Journal of Applied Sciences, Engineering and Technology 2015 5:549-558
Received: May 16, 2015 | Accepted: June 22, 2015 | Published: October 15, 2015
Abstract
Diabetes mellitus has turned out to be a common chronic disease that affects between 2 and 4% of the total population. Recently, most of the system uses association rule mining for diagnosing type-II diabetes mellitus. The most vital concern of association rules is that rules are derived from the complete data set with no validation on samples. Previously, Association rule based Modified Particle Swarm Optimization and Least Squares Support Vector Machine classification is introduced with the capability to lessen the number of rules, looks for association rules on a training set and at last validates them on an independent test set. On the other hand, it only employs categorical data. In case of Type-II Diabetes Mellitus medical diagnosis, the exploitation of continuous data might be essential. With the aim of solving this complication, Improved Frequent Pattern Growth (IFP-Growth) with Hybrid Enhanced Artificial Bee Colony-Advanced Kernel Support Vector Machine (HEABC-AKSVM-IFP Growth) classification based Association Rule Mining (ARM) system is proposed in this study to create rules. This study introduces improved FP-growth to effectively derive frequent patterns including from a vague database in which items possibly will come into view in medical database. Then, HEABC-AKSVM-IFP Growth classifier is employed to create the association rules from the frequent item sets, also keeping away from the rule redundancy and inconsistencies at the time of mining process. Then, results are simulated and evaluated against few classification techniques in terms of classification accuracy, number of derived rules and processing time.
Keywords:
Advanced kernel support vector machine, association rule mining, enhanced artificial bee colony, frequent patterns, improved frequent pattern growth algorithm, type-II diabetes,
References
- Al Jarullah, A.A., 2011. Decision tree discovery for the diagnosis of type II diabetes. Proceeding of IEEE International Conference on Novations in Information Technology (IIT, 2011), pp: 303-307.
CrossRef -
Anburajan, M., S. Sivanandam, S. Bidyarasmi, B. Venkatraman, M. Menaka and B. Raj, 2011. Changes of skin temperature of parts of the body and serum asymmetric dimethylarginine (ADMA) in type-2 diabetes mellitus Indian patients. Proceeding of 33rd Annual International Conference of the IEEE EMBS, pp: 6254-6259.
CrossRef
-
Bansal, J.C., P.K. Singh, M. Saraswat, A. Verma, S.S. Jadon and A. Abraham, 2011. Inertia weight strategies in particle swarm optimization. Proceeding of 3rd World Congress on in Nature and Biologically Inspired Computing (NaBIC), pp: 633-640.
CrossRef
-
Barakat, N.H., A.P. Bradley and M.N.H. Barakat, 2010. Intelligible support vector machines for diagnosis of diabetes mellitus. IEEE T. Inf. Technol. B., 14(4): 1114-1120.
CrossRef PMid:20071261
-
Guo, Y., G. Bai and Y. Hu, 2012. Using bayes network for prediction of type-2 diabetes. Proceeding of the IEEE International Conference for Internet Technology and Secured Transactions, pp: 471-472.
-
Haupt, R. and S. Haupt, 1998. Practical Genetic Algorithms. Wiley-Interscience Publication, New York.
CrossRef PMCid:PMC108982
-
Karthikeyan, T. and K. Vembandasamy, 2014. A refined continuous ant colony optimization based FP-growth association rule technique on type 2 diabetes. Int. Rev. Comput. Software, 9(8): 1476-1483.
CrossRef
-
Karthikeyan, T. and K. Vembandasamy, 2015. A novel algorithm to diagnosis type II diabetes mellitus based on association rule mining using MPSO-LSSVM with outlier detection method. Indian J. Sci. Technol., April, 8(S8): 310-320.
- Karthikeyan, T., R. Ragavan and K. Vembandasamy, 2013. Hierarchical K-means clustering algorithm for an E-care of diabetes mellitus. Int. J. Adv. Res. Comput. Sci. Software Eng., 3(12): 653-660.
- Kumari, S. and A. Singh, 2013. A data mining approach for the diagnosis of diabetes mellitus. Proceeding of 7th International Conference on In Intelligent Systems and Control (ISCO, 2012), pp: 373-375.
CrossRef
- Lee, C.S. and M.H. Wang, 2011. A fuzzy expert system for diabetes decision support application. IEEE T. Syst. Man Cy B, 41(1): 139-153.
CrossRef PMid:20501347
- Oner, Y., T. Tunc, E. Egrioglu and Y. Atasoy, 2013. Comparisons of logistic regression and artificial neural networks in lung cancer data. Am. J. Intell. Syst., 3(2): 71-74.
- Patel, V.R. and R.G. Mehta, 2011. Impact of outlier removal and normalization approach in modified k-means clustering algorithm. Int. J. Comput. Sci. Issues (IJCSI), 8(5): 331-336.
-
Sankaranarayanan, S. and T.P. Perumal, 2014. A predictive approach for diabetes mellitus disease through data mining technologies. Proceeding of 2014 World Congress on Computing and Communication Technologies (WCCCT), pp: 231-233.
CrossRef
-
Shokouhifar, M. and G.S. Abkenar, 2011. An artificial bee colony optimization for MRI fuzzy segmentation of brain tissue. Proceeding of the International Conference on Management and Artificial Intelligence. Indonesia, pp: 6-10.
- Tudor, I., 2008. Association rule mining as a data mining technique. B. U. Petrol-Gaze Din Ploiesti, LX(1): 49-56.
Competing interests
The authors have no competing interests.
Open Access Policy
This article is distributed under the terms of the Creative Commons Attribution 4.0 International License (http://creativecommons.org/licenses/by/4.0/), which permits unrestricted use, distribution, and reproduction in any medium, provided you give appropriate credit to the original author(s) and the source, provide a link to the Creative Commons license, and indicate if changes were made.
Copyright
The authors have no competing interests.
|
|
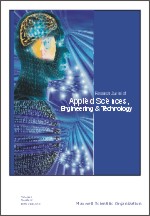 |
ISSN (Online): 2040-7467
ISSN (Print): 2040-7459 |
 |
Information |
|
|
|
Sales & Services |
|
|
|