Research Article | OPEN ACCESS
Mixed Pixel Wise Characterization Based on HDP-HMM and Hyperspectral Image Shape Detection using Hybrid Canny Edge Detection and WPDF
1D. Regan and 2S.K. Srivatsa
1St. Peter’s University,
2Prathyusha Institute of Technology and Management, Chennai, Tamilnadu, India
Research Journal of Applied Sciences, Engineering and Technology 2015 12:1344-1357
Received: May ‎30, ‎2015 | Accepted: August ‎5, ‎2015 | Published: December 25, 2015
Abstract
Hyper Spectral Imaging (HSI) gathers and processes information from across the electromagnetic spectrum. The information enclosed in hyperspectral data permits the characterization, recognition and classification of the land-covers with enhanced accuracy and robustness. On the other hand, quite a lot of vital complications must be considered during the classification process of hyperspectral data, among which the maximum quantity of spectral channels, the spatial unevenness of the spectral signature, shape discovery of the images and the value of data. Above all, the maximum quantity of spectral channels and low number of labeled training samples pose the setback of the curse of dimensionality and, accordingly, result in the possibility of overfitting the training data. With the aim of solving all these complications, in this study presented the framework of Support Vector Machine (SVM) together with Fuzzy Sigmoid Kernel Function (SVM-FSK) in the circumstance of HSI classification and analyzing their features in the hyperspectral domain. A Kernel Fisher Discriminant Analysis (KFDA) model is employed for the purpose of dimensionality reduction of HSI. The KFDA dimensionality reduction scheme depends on the selection of the kernel in a higher-dimensional HSI feature space. In order to enhance the gradient level of spatial information, employed Improved Empirical Mode Decomposition (IEMD) with Gaussian Firefly Algorithm (GFA) (IEMD-GFA) to boost the mixed pixel wise SVM-FSK classification accuracy. During the process of IEMD scheme, the identifiable of Intrinsic Mode Functions (IMFs) of spectral band, weight values of IMFs are computed with the help of GFA. In order to identify the shape of HSI, novel hybrid scheme depending on the canny operator and fuzzy entropy theory is formulated. This scheme computes the fuzzy entropy of gradients from an image to make a decision on the threshold for the canny operator. For the purpose of detecting the edges and to discover the shape of the object Weibull Probability Density Function (WPDF) scheme is used. The obtained both spectral and spatial pixels are classified using SVM-FSK and estimated by using Hierarchical Dirichlet Process (HDP)-Hidden Markov Model (HMM). The proposed SVM-FSK is assessed with hyperspectral AVIRIS Indian Pine dataset. It shows that the proposed dimensionality reduction with SVM-FSK classification shows improved classification accuracy in terms of parameters like overall accuracy, standard deviation and mean.
Keywords:
Canny Edge Detection, Gaussian Firefly Algorithm (GFA), Hierarchical Dirichlet Process (HDP), Hyperspectral image classification, Hidden Markov Model (HMM), Hidden Markov Model (HMM), Hyper Spectral Images (HSI), Pixel wise characterization, Support Vector Machine (SVM), Spectral Gradient enhancement, shape detection, Weibull Probability Distribution Function (WPDF),
Competing interests
The authors have no competing interests.
Open Access Policy
This article is distributed under the terms of the Creative Commons Attribution 4.0 International License (http://creativecommons.org/licenses/by/4.0/), which permits unrestricted use, distribution, and reproduction in any medium, provided you give appropriate credit to the original author(s) and the source, provide a link to the Creative Commons license, and indicate if changes were made.
Copyright
The authors have no competing interests.
|
|
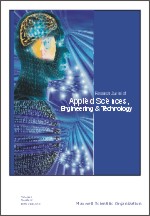 |
ISSN (Online): 2040-7467
ISSN (Print): 2040-7459 |
 |
Information |
|
|
|
Sales & Services |
|
|
|