Research Article | OPEN ACCESS
Application of Artificial Neural Network in Predicting Compressive Strength of Vege Block
1O.A. Johnson, 1N. Madzlan, 1I. Kamaruddin, 1O.O. Oloruntobi and 2H.S. Abdulrahma
1Department of Civil and Environmental Engineering, Universiti Teknologi PETRONAS 31750 Seri Iskandar, Tronoh, Malaysia
2Department of Civil Engineering, Federal University of Technology Minna, Nigeria
Research Journal of Applied Sciences, Engineering and Technology 2016 1:37-42
Received: July 13, 2015 | Accepted: August 30, 2015 | Published: January 05, 2016
Abstract
Vege block is a building or construction block manufactured from the mixture of sand aggregates and waste cooking oil as a sustainable binder. This study explores the use of Artificial Neural Network (ANN) in the prediction of the compressive strength. Nine ANN models were developed with different hidden neurons ranges from 7-15 and it performances were tested after properly trained using the Root Mean Square Error (RMSE) and coefficient of determination(R-square) and correlation coefficient (r). The result shows that model with 8 hidden neurons show a better performance.
Keywords:
Artificial neural network, compressive strength, linear regression, waste cooking oil,
References
-
Deshpande, N., S. Londhe and S. Kulkarni, 2014. Modeling compressive strength of recycled aggregate concrete by artificial neural network, model tree and non-linear regression. Int. J. Sustain. Built Environ., 3(2): 187-198.
CrossRef
-
Duan, Z.H., S.C. Kou and C.S. Poon, 2013. Prediction of compressive strength of recycled aggregate concrete using artificial neural networks. Constr. Build. Mater., 40: 1200-1206.
CrossRef
-
Erdal, M., 2009. Prediction of the compressive strength of vacuum processed concretes using artificial neural network and regression techniques. Sci. Res. Essay, 4(10): 1057-1065.
-
Kewalramani, M.A. and R. Gupta, 2006. Concrete compressive strength prediction using ultrasonic pulse velocity through artificial neural networks. Automat. Constr., 15(3): 374-379.
CrossRef
-
Lande, P. and A. Gadewar, 2012. Application of artificial neural networks in prediction of compressive strength of concrete by using ultrasonic pulse velocities. IOSR J. Mech. Civil Eng., 3(1): 34-42.
CrossRef
-
Pezo, L., M. Arsenovic and Z. Radojevic, 2014. ANN model of brick properties using LPNORM calculation of minerals content. Ceram. Int., 40(7): 9637-9645.
CrossRef
-
Qasrawi, H.Y., 2000. Concrete strength by combined nondestructive methods simply and reliably predicted. Cement Concrete Res., 30(5): 739-746.
CrossRef
-
Tapkin, S., M. Tuncan, Ö. Ariöz, A. Tuncan and K. Ramyar, 2006. Estimation of concrete compressive strength by using ultrasonic pulse velocities and artificial neural networks. Proceeding of 11th FIGES User's Conference for Computer Aided Engineering and System Modeling, September.
Competing interests
The authors have no competing interests.
Open Access Policy
This article is distributed under the terms of the Creative Commons Attribution 4.0 International License (http://creativecommons.org/licenses/by/4.0/), which permits unrestricted use, distribution, and reproduction in any medium, provided you give appropriate credit to the original author(s) and the source, provide a link to the Creative Commons license, and indicate if changes were made.
Copyright
The authors have no competing interests.
|
|
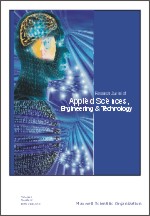 |
ISSN (Online): 2040-7467
ISSN (Print): 2040-7459 |
 |
Information |
|
|
|
Sales & Services |
|
|
|