Research Article | OPEN ACCESS
A Survey on Quantitative Metrics for Assessing the Quality of Fused Medical Images
1S. Kavitha and 2K.K. Thyagharajan
1Department of CSE, SSN College of Engineering, Chennai-603 110, Tamilnadu, India
2Department of ECE, RMD Engineering College, Chennai-601 206, Tamilnadu, India
Research Journal of Applied Sciences, Engineering and Technology 2016 3:282-293
Received: August 3, 2015 | Accepted: September 3, 2015 | Published: February 05, 2016
Abstract
The fused image derived from multimodality, multi-focus, multi-view and multidimensional, for real world applications in the field of medical imaging, remote sensing, satellite imaging, machine vision etc., are gaining much attention in the recent research. Therefore, it is important to validate the fused image in different perspectives such as information, edge, structure, noise and contrast for quality analysis. From this aspect, the information of fused image should be better than the source images without loss of information and false information. This survey is focused on analyzing the various quantitative metrics that are used in the literature to measure the enhanced information of fused image when it is compared to the source/reference images. The objective of this study is to group or classify the metrics under different categories such as information, noise, error, correlation and structural similarity measures for discussion and analysis. In reality, the calculated metric values are useful in determining the suitable fusion technique of the particular dataset with its required perspective as an outcome of the fusion process.
Keywords:
Correlation metrics, error metrics, fused image, information metrics, noise metrics, qualitative measures, quantitative metrics, structural similarity metrics,
References
-
Anusha, R. and S. Kavitha, 2015. Comparative analysis of DWT based image fusion techniques using a new quality fusion metric. Int. J. Appl. Eng. Res., 10(24): 27277-27282.
-
Blasch, E., X. Li, G. Chen and W. Li, 2008. Image quality assessment for performance evaluation of image fusion. Proceeding of 11th International Conference on Information Fusion, pp: 1-6.
-
Brain Images Source, 2014a. Retrieved from: http://www.med.harvard.edu/aanlib/.
Direct Link
-
Brain Images Source, 2014b. Retrieved from: http://radiopaedia.org/.
Direct Link
-
Chandler, D.M. and S.S. Hemami, 2007. VSNR: A wavelet-based visual signal-to-noise ratio for natural images. IEEE T. Image Process., 16(9): 2284-2298.
CrossRef PMid:17784602
-
Chang, C.I., 2000. An information theoretic based approach to spectral variability, similarity and discriminability for hyper spectral image analysis. IEEE T. Inform. Theory, 46(5): 1927-1932.
CrossRef
-
Chen, G., C. Yang and S. Xie, 2006a. Edge-based structural similarity for image quality assessment. Proceeding of International Conference on Acoustics, Speech, Signal Processing, pp: 14-19.
-
Chen, G., C. Yan and S. Xie, 2006b. Gradient-based structural similarity for image quality assessment. Proceeding of International Conference on Image Process, pp: 2929-2932.
CrossRef
-
Choi, Y., E. Sharifahmadian and S. Latifi, 2014. Quality assessment of image fusion methods in transform domain. Int. J. Inform. Theor. (IJIT), 3(1).
-
Cosman, P.C., R.M. Gray and R.A. Olshen, 1994. Evaluating quality of compressed medical images: SNR, subjective ratingand diagnostic accuracy. P. IEEE, 82(6): 919-932.
CrossRef
-
Cvejic, N., C.N. Canagarajah and D.R. Bull, 2006. Image fusion metric based on mutual information and Tsallis entropy. Electron. Lett., 42: 626-627.
CrossRef
-
Eskicioglu, A.M. and P.S. Fisher, 1995. Image quality measures and their performance. IEEE T. Commun., 43(12): 2959-2965.
CrossRef
-
Gao, C., K. Panetta and S. Agaian, 2013. No reference color image quality measures. Proceeding of IEEE International Conference on Cybernetics (CYBCONF), pp: 243-248.
CrossRef
-
Image Fusion, 2014. Retrieved form: http://www.imagefusion.org/.
Direct Link
-
Jagalingam, P. and A.V. Hegdeb, 2015. A review of quality metrics for fused image. Aquat. Proc., 4: 133-142.
CrossRef
-
James, A.P. and B.V. Dasarathy, 2014. Medical image fusion: A survey of the state of the art. Inform. Fusion, 19: 4-19.
CrossRef
-
Kavitha, S. and K.K. Thyagharajan, 2014. Dual channel pulse coupled neural network algorithm for fusion of multimodality brain Images with quality analysis. Appl. Med. Inform., 35(3): 31-39.
-
Kim, D., H. Han and R. Park, 2010. Gradient information-based image quality metric. IEEE T. Consum. Electr., 56(2): 930-936.
CrossRef
-
Larson, E.C. and D.M. Chandler, 2010. Most apparent distortion: Full-reference image quality assessment and the role of strategy. J. Electron. Imaging, 19(1): 011006:1-011006:21.
-
Lin, W. and C.C. Jay-Kuo, 2011. Perceptual visual quality metrics: A survey. J. Vis. Commun. Image R., 22(4): 297-312.
CrossRef
-
Liu, Z., D.S. Forsyth and R. Laganiere, 2008. A feature-based metric for the quantitative evaluation of pixel-level image fusion. Comput. Vis. Image Und., 109(1): 56-68.
CrossRef
-
Mitchell, H.B., 2010. Image fusion: Theories, techniques and applications, Springer, Berlin.
CrossRef
-
Naidu, V.P.S. and J.R. Raol, 2008. Pixel-level image fusion using wavelets and principal component analysis. Defence Sci. J., 58(3): 338-352.
CrossRef
-
Petrovic, V., 2007. Subjective tests for image fusion evaluation and objective metric validation. Inform. Fusion, 8(2): 208-216.
CrossRef
-
Piella, G. and H. Heijmans, 2003. A new quality metric for image fusion. Proceeding of International Conference on Image Processing, pp: 173-176.
CrossRef
-
Qu, G., D. Zhang and P. Yan, 2002. Information measure for performance of image fusion. Electron. Lett., 38(7): 313-315.
CrossRef
-
Radhika, R. and S. Kavitha, 2013. Neuro-fuzzy logic based fusion algorithm for multimodality images. Proceeding of International Conference on Advanced Computer Science and Information Technology, Asian Society for Academic Research held at Coimbatore, pp: 81-84, ISBN: 978-81-927147-4-5.
-
Rahmani, S., M. Strait, D. Merkurjev, M. Moeller and T. Wittman, 2010. An adaptive IHS pan-sharpening method. IEEE Geosci. Remote S., 7(4): 746-750.
CrossRef
-
Rajkumar, S. and S. Kavitha, 2010. Redundancy discrete wavelet transform and contourlet transform for multimodality medical image fusion with quantitative analysis. Proceeding of International Conference on Emerging Trends in Engineering and Technology (ICETET, 2010), pp: 134-139.
CrossRef
-
Ranchln, T. and L. Wald, 2000. Fusion of high spatial and spectral resolution images: The ARSlS concept and its implementation. Photogramm. Eng. Rem. S., 66(1): 49-61.
-
Rockinger, O., 2013. Fusion Toolbox. Retrieved form: http://www.metapix.de/.
Direct Link
-
Sampat, M.P., Z. Wang, S. Gupta, A.C. Bovik and M.K. Markey, 2009. Complex wavelet structural similarity: A new image similarity index. IEEE T. Image Process., 18(11): 2385-2401.
CrossRef PMid:19556195
-
Sheikh, H.R., A.C. Bovik and G. De Veciana, 2005. An information fidelity criterion for image quality assessment using natural scene statistics. IEEE T. Image Process., 14(12): 2117-2128.
CrossRef PMid:16370464
-
Sheikh, H.R. and A.C. Bovik, 2006. Image information and visual quality. IEEE T. Image Process., 15(2): 430-444.
CrossRef PMid:16479813
-
Singh, R. and A. Khare, 2013. Multiscale medical image fusion in wavelet domain. Sci. World J., 2013: 10, Article ID: 521034, Doi: 10. 1155/2013/521034.
-
Vijayaraj, V., N.H. Younan and C.G. O'Hara, 2006. Quantitative analysis of pansharpened images. Opt. Eng., 45(4).
-
VQEG, 2000. Final Report from the Video Quality Experts Group on the Validation of Objective Models of Video Quality Assessment. Retrieved form: http://www.vqeg.org/.
Direct Link
-
Wang, Q., D. Yu and Y. Shen, 2009. An overview of image fusion metrics. Proceeding of IEEE Conference on Instrumentation and Measurement Technology (12MTC’09), pp: 918-923.
CrossRef
-
Wang, Q., Y. Shen and J. Jin, 2008. Performance evaluation of image fusion techniques. In: Stathaki T., (Ed.), Image Fusion: Algorithms and Applications, Ch. 19, pp: 469-492.
CrossRef
-
Wang, P. and B. Liu, 2008. A novel image fusion metric based on multi-scale analysis. Proceeding of IEEE International Conference on Signal Processing, pp: 965-968.
-
Wang, Z. and A.C. Bovik, 2002. A universal image quality index. IEEE Signal Proc. Let., 9(3): 81-84.
CrossRef
-
Wang, Z. and A.C. Bovik, 2009. Mean squared error: Love it or leave it? -A new look at signal fidelity measures. IEEE Signal Proc. Mag., 26(1): 98-117.
CrossRef
-
Wang, Z. and Q. Li, 2011. Information content weighting for perceptual image quality assessment. IEEE T. Image Process., 20(5): 1185-1198.
CrossRef PMid:21078577
-
Wang, Z., A.C. Bovik and E.P. Simancelli, 2005. Structural Approaches to Image Quality Assessment. In: Bovik, A. (Ed.), 2nd Edn., Handbook of Image and Video Processing, Academic Press, London.
CrossRef
-
Wang, Z., A.C. Bovik and L. Lu, 2002. Why is image quality assessment so difficult? Proceeding of the IEEE International Conference on Acoustics, Speech and Signal Processing.
CrossRef
-
Wang, Z., A.C. Bovik, H.R. Sheikh and E.P. Simoncelli, 2004. Image quality assessment: From error measurement to structural similarity. IEEE T. Image Process., 13(1): 1-14.
CrossRef
-
Wei, C. and R.S. Blum, 2009. Theoretical analysis of correlation-based quality measures for weighted averaging image fusion. Inform. Fusion, 11: 301-310.
CrossRef
-
Xydeas, C.S. and V. Petrovic, 2000. Objective image fusion performance measure. Electron. Lett., 36(4): 308-309.
CrossRef
-
Yang, C., J. Zhang, X. Wang and X. Liu, 2008a. A novel similarity based quality metric for image fusion. Inform. Fusion, 9: 156-160.
CrossRef
-
Yang, C., W. Gao and L. Po, 2008b. Discrete wavelet transform-based structural similarity for image quality assessment. Proceeding of International Conference on Image Process, pp: 377-380.
-
Yang, Y., W. Zheng and S. Huang, 2014. Effective multifocus image fusion based on HVS and BP neural network. Sci. World J., 2014: 10, Article ID: 281073, Doi: 10.1155/2014/281073.
CrossRef
-
Zhang, P., C. Fei, Z. Peng, J. Li and H. Fan, 2015. Multifocus image fusion using biogeography-based optimization. Math. Probl. Eng., 2015: 14, Article ID: 340675, Doi: 10.1155/2015/340675.
CrossRef
-
Zhang, Y., 2008. Methods for image fusion quality assessment-a review, comparison and analysis. Proceeding of the International Archives of the Photogrammetry, Remote Sensing and Spatial Information Sciences, 37(B7), Beijing, China.
-
Zhang, Z. and R.S. Blum, 1999. A categorization and study of multiscale-decomposition-based image fusion schemes. P. IEEE, 4: 1315-1328.
CrossRef
-
Zheng, Y., Z. Qin, L. Shao and X. Hou, 2008. A novel objective image quality metric for image fusion based on Renyi entropy. J. Inform. Technol., 7(6): 930-935.
CrossRef
-
Zhou, W., 2009. SSIM Metric. Retrieved form: http: //www.ece.uwaterloo.ca/~z70wang/research ssim/.
Direct Link
-
Zhu, S.L., 2002. Image fusion using wavelet transform. Proceeding of Symposium on Geospatial Theory, Process and Applications, Ottawa.
Competing interests
The authors have no competing interests.
Open Access Policy
This article is distributed under the terms of the Creative Commons Attribution 4.0 International License (http://creativecommons.org/licenses/by/4.0/), which permits unrestricted use, distribution, and reproduction in any medium, provided you give appropriate credit to the original author(s) and the source, provide a link to the Creative Commons license, and indicate if changes were made.
Copyright
The authors have no competing interests.
|
|
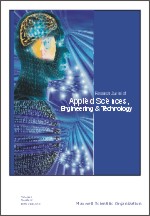 |
ISSN (Online): 2040-7467
ISSN (Print): 2040-7459 |
 |
Information |
|
|
|
Sales & Services |
|
|
|