Research Article | OPEN ACCESS
Bright Lesion Detection in Color Fundus Images Based on Texture Features
V. Ratna Bhargavi, Ranjan K. Senapati, Ganesh Methra and Sujitha Kandanulu
Department of Electronics and Communication Engineering, K L University, Vaddeswaram,
Guntur-522502, Andhra Pradesh, India
Research Journal of Applied Sciences, Engineering and Technology 2016 3:355-360
Received: August 28, 2015 | Accepted: October 11, 2015 | Published: February 05, 2016
Abstract
In this study a computer aided screening system for the detection of bright lesions or exudates using color fundus images is proposed. The proposed screening system is used to identify the suspicious regions for bright lesions. A texture feature extraction method is also demonstrated to describe the characteristics of region of interest. In final stage the normal and abnormal images are classified using Support vector machine classifier. Our proposed system obtained the effective detection performance compared to some of the state-of-art methods.
Keywords:
Classification, computer aided screening, diabetic retinopathy, feature extraction, segmentation,
References
-
Agurto, C., V. Murray, E. Barriga, S. Murillo, M. Pattichis, H. Davis, S. Russell, M. Abramoff and P. Soliz, 2010. Multiscale AM-FM methods for diabetic retinopathy lesion detection. IEEE T. Med. Imaging, 29(2): 502-512.
CrossRef PMid:20129850 PMCid:PMC2825390 -
Canny, J., 1986. Computational approach to edge detection. IEEE T. Pattern Anal., 8(6): 679-698.
CrossRef -
Deepak, K.S. and J. Sivaswamy, 2012. Automatic assessment of macular edema from color retinal images. IEEE T. Med. Imaging, 31(3): 766-776.
CrossRef PMid:22167598 -
Esmaeli, M., H. Rabbani, A.M. Dehnavi and A. Dehgani, 2012. Automatic detection of exudates and optic disk in retinal images using curvelet transform. IET Image Process., 6(7): 1-9.
CrossRef -
Giancardo, L., F. Meriaudeau, T.P. Karnowski, Y. Li, S. Garg et al., 2012. Exudate-based diabetic macular edema detection in fundus images using publicly available datasets. Med. Image Anal., 16: 216-226.
CrossRef PMid:21865074 -
Harangi, B. and A. Hajdu, 2014. Automatic exudates detection by fusing multiple active contours and region wise classification. Comput. Biol. Med., 54: 156-171.
CrossRef PMid:25255154 -
International Diabetes Federation (IDF), 2013. Public Health Foundation of India. Data from the 2013 Fact Sheet Diabetes in India-Fact Sheet. Retrieved form: http://www.cadiresearch.org/topic/diabetes-indians/diabetes-urban-india.
Direct Link -
Jayakumari, C. and T. Santhanam, 2007. Detection of hard exudates for diabetic retinopathy using contextual clustering and fuzzy art neural network. Asian J. Inform. Technol., 8: 842-846.
-
Kauppi, T., V. Kalesnykiene, J.K. Kmrinen, L. Lensu, I. Sorr, A. Raninen, R. Voutilainen, H. Uusitalo, H. Kälviäinen and J. Pietilä, 2007. Diaretdb1 diabetic retinopathy database and evaluation protocol. Proceeding of the 11th Conference on Medical Image Understanding and Analysis, pp: 61-65.
CrossRef -
Lin, P. and Z. Bing-Kun, 2012. An effective approach to detect hard exudates in color retinal image. In: Qian, Z. and et al. (Eds.): Recent Advances in CSIE, 2011. LNEE 124, Springer-Verlag, Berlin, Heidelberg, pp: 541-546.
CrossRef -
Messidor, 2010. Methods to Evaluate Segmentation and Indexing Techniques in the Field of Retinal Ophthalmology. Retrieved from: http://messidor.crihan.fr/download-en.php. (Accessed on: Sep. 23, 2011).
Direct Link -
Pachiyappan, A., U.N. Das, T.V.S.P. Murthy and R. Tatavarti, 2012. Automated diagnosis of diabetic retinopathy and glaucoma using fundus and OCT images. Lipids Health Dis., 11: 73.
CrossRef PMid:22695250 PMCid:PMC3477058 -
Petschnigg, G., R. Szeliski, M. Agrawala, M. Cohen, H. Hoppe and K. Toyama, 2004. Digital photography with flash and no-flash image pairs. ACM T. Graphic., 23(3): 664-672.
CrossRef -
Pires, R., H.F. Jelinek, J. Wainer and S. Goldensteindo, E. Valle and A. Rocha, 2013. Assessing the need for referral in automatic diabetic retinopathy detection. IEEE T. Bio-Med. Eng., 60(12): 3391-3398.
-
Ravishankar, S., A. Jain and A. Mittal, 2009. Automated feature extraction for early detection of diabetic retinopathy in Fundus images. Proceeding of the IEEE Conference on Computer Vision and Pattern Recognition (CVPR, 2009), pp: 210-217.
CrossRef -
Rocha, A., T. Carvalho, H. Jelinek, S. Goldenstein and J. Wainer, 2012. Points of interest and visual dictionaries for automatic retinal lesion detection. IEEE T. Bio-Med. Eng., 59(8): 2244-2253.
-
Roychowdhury, S., D.D. Keshabparhi and K.K. Parhi, 2014. DREAM: Diabetic retinopathy analysis using machine learning. IEEE J. Bio-Med. Health Inform., 18(5): 1717-1728.
CrossRef PMid:25192577 -
Sánchez, C.I., R. Hornero, M.I. López, M. Aboy, J. Poza and D. Abásolo, 2009. A novel automatic image processing algorithm for detection of hard exudates based on retinal image analysis. Med. Eng. Phys., 30(3): 350-357.
CrossRef PMid:17556004 -
Sinthanayothin, C., J. Boyce, H. Cook and T. Williamson, 1999. Automated localisation of the optic disc, fovea and retinal blood vessels from digital colour fundus images. Brit. J. Ophthalmol., 83(8): 902-10.
CrossRef PMid:10413690 PMCid:PMC1723142 -
Sopharak, A., B. Uyyanonvara, S. Barman and T.H. Williamson, 2008. Automatic detection of diabetic retinopathy exudates from non-dilated retinalimages using mathematical morphology methods. Comput. Med. Imag. Grap., 32(8): 720-727.
CrossRef PMid:18930631 -
Van Grinsven, M.J.J.P., A. Chakravartyy, J. Sivaswamy, T. Theelen, B. van Ginneken and C.I. Sánchez, 2013. A bag of words approach for discriminating between retinal images containing exudates or drusen. Proceeding of the IEEE 10th International Symposium on Biomedical Imaging. San Francisco, CA, pp: 1444-1447.
CrossRef -
Welfer, D., J. Scharcanski and D.R. Marinho, 2010. A coarse-to-fine strategy for automatically detecting exudates in color eye fundus images. Comput. Med. Imag. Grap., 34(3): 228-235.
CrossRef PMid:19954928 -
Wisaeng, K., N. Hiransakolwong and E. Pothiruk, 2015. Automatic detection of exudates in retinal images based on thresholding moving average models. Biophysics, 60(2): 288-297.
CrossRef
Competing interests
The authors have no competing interests.
Open Access Policy
This article is distributed under the terms of the Creative Commons Attribution 4.0 International License (http://creativecommons.org/licenses/by/4.0/), which permits unrestricted use, distribution, and reproduction in any medium, provided you give appropriate credit to the original author(s) and the source, provide a link to the Creative Commons license, and indicate if changes were made.
Copyright
The authors have no competing interests.
|
|
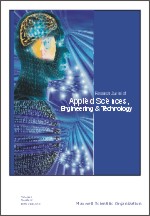 |
ISSN (Online): 2040-7467
ISSN (Print): 2040-7459 |
 |
Information |
|
|
|
Sales & Services |
|
|
|