Research Article | OPEN ACCESS
Dual Tree Complex Wavelet Transform Based Compression of Optical Coherence Tomography Images for Glaucoma Detection using Modular Neural Network
M. Hemalatha and S. Nithya
Department of Computer Science, Dr. SNS Rajalakshmi College of Arts and Science,
Coimbatore-49, India
Research Journal of Applied Sciences, Engineering and Technology 2016 11:825-834
Received: August 5, 2016 | Accepted: September 14, 2016 | Published: December 05, 2016
Abstract
Background/Objectives: In worldwide, Glaucoma is basically a second major retinal disease that results in permanent blindness. Loss of Retinal Nerve Fiber Layer (RNFL) is the outcome of glaucoma disease. RNFL thickness is evaluated as a function of spatial information from Optical Coherence Tomography (OCT) images is a significant diagnostics indicator intended for glaucoma disease. However, due to factors such as low image contrast, speckle noise, high spatial resolution, exact compression of OCT is complex. To solve above issues, a Dual Tree Complex Wavelet Transform (DTCWT) based OCT image compression is proposed in this research work. Methods/Statistical analysis: The proposed method consists of five phases such as pre-processing, feature extraction, RNFL segmentation, glaucoma classification and OCT compression. Initially, OCT image is pre-processed for remove the speckle noise using kuan filter. Secondly, the RNFL based texture features are extracted by using Gray Level Covariance Matrix (GLCM) and the scrupulous features are chosen by Principal Component Analysis (PCA). Then, RNFL in OCT is segmented by Improved Artificial Bee Colony (IABC) clustering algorithm. After that the glaucoma is classified as normal, medium and severe by Modular Neural Network (MNN). Finally, DTCWT is used to compress the OCT image. Results: Experimental results show that the proposed MNN is efficient for detecting glaucoma compared with the existing detection algorithms.
Keywords:
Classification , compression, feature extraction, Glaucoma, kuan filter , optical coherence tomography , RNFL , segmentatio,
References
-
Abu-Mouti, F.S. and M.E. El-Hawary, 2012. Overview of Artificial Bee Colony (ABC) algorithm and its applications. Proceeding of the IEEE International Systems Conference (SysCon, 2012).
Direct Link -
Agarwal, A., S. Gulia, S. Chaudhary, M.K. Dutta, C.M. Travieso and J.B. Alonso-Hernández, 2015. A novel approach to detect glaucoma in retinal fundus images using cup-disk and rim-disk ratio. Proceeding of the 4th International Work Conference on Bioinspired Intelligence (IWOBI, 2015), pp: 139-144.
Direct Link -
Ahmed, N., T. Natarajan and K.R. Rao, 1974. Discrete cosine transform. IEEE T. Comput., C-23(1): 90-93.
Direct Link -
Anantrasirichai, N., A. Achim, J.E. Morgan, I. Erchova and L. Nicholson, 2013. SVM-based texture classification in optical coherence tomography. Proceeding of the IEEE 10th International Symposium on Biomedical Imaging (ISBI, 2013).
Direct Link -
Bock, R., J. Meier, L.G. Nyúl, J. Hornegger and G. Michelson, 2010. Glaucoma risk index: Automated glaucoma detection from color fundus images. Med. Image Anal., 14(3): 471-481.
Direct Link -
Burrus, C.S., R.A. Gopinath and H. Guo, 1998. Introduction to Wavelets and Wavelet Transforms: A Primer. Prentice Hall, Upper Saddle River, NJ, USA.
Direct Link -
Dutta, M.K., A.K. Mourya, A. Singh, M. Parthasarathi, R. Burget and K. Riha, 2014. Glaucoma detection by segmenting the super pixels from fundus colour retinal images. Proceeding of the International Conference on Medical Imaging, m-Health and Emerging Communication Systems (MedCom), pp: 86-90.
Direct Link -
Fuente-Arriaga, J.A.D.L., E.M. Felipe-Riverón and E. Gardu-o-Calderón, 2014. Application of vascular bundle displacement in the optic disc for glaucoma detection using fundus images. Comput. Biol. Med., 47: 27-35.
Direct Link -
Gabriele, M.L., H. Ishikawa, G. Wollstein, R.A. Bilonick, K.A. Townsend, L. Kagemann, M. Wojtkowski, V.J. Srinivasan, J.G. Fujimoto, J.S. Duker and J.S. Schuman, 2008. Optical coherence tomography scan circle location and mean retinal nerve fiber layer measurement variability. Invest. Ophth. Vis. Sci., 49(6): 2315-2321.
Direct Link -
Hatanaka, Y., C. Muramatsu, A. Sawada, T. Hara, T. Yamamoto and H. Fujita, 2012. Glaucoma risk assessment based on clinical data and automated nerve fiber layer defects detection. Proceeding of the Annual International Conference of the IEEE Engineering in Medicine and Biology Society (EMBC, 2012). San Diego, California, USA, pp: 5963-5966.
Direct Link -
Hoyt, W.F., L. Frise´n and N.M. Newman, 1973. Fundoscopy of nerve fiber layer defects in glaucoma. Invest. Ophth. Vis. Sci., 12(11): 814-829.
Direct Link -
Khalil, T., S. Khalid and A.M. Syed, 2014. Review of machine learning techniques for glaucoma detection and prediction. Proceeding of the Science and Information Conference. London, UK, pp: 438-442.
Direct Link -
Mousavi, M., K. Lurie, J. Land, T. Javidi and A.K. Ellerbee, 2014. Telemedicine + OCT: Toward design of optimized algorithms for high-quality compressed images. Proceeding of the SPIE 8936, Design and Quality for Biomedical Technologies VII, pp: 10.
Direct Link -
Mrugacz, M. and A. Bakunowicz-Lazarczyk, 2005. Optical coherence tomography measurement of the retinal nerve fiber layer in normal and juvenile glaucomatous eyes. Ophthalmologica, 219(2): 80-85.
Direct Link -
Muramatsu, C., Y. Hayashi, A. Sawada, Y. Hatanaka, T. Hara, T. Yamamoto and H. Fujita, 2010. Detection of retinal nerve fiber layer defects on retinal fundus images for early diagnosis of glaucoma. J. Biomed. Opt., 15(1): 016021.
Direct Link -
Odstrcilik, J. et al., 2014. Analysis of the retinal nerve fiber layer texture related to the thickness measured by optical coherence tomography. Proceeding of the VIP Image Conference. Funchal-Madeira, Portugal, 19: 105-110.
-
Parikh, R.S., S. Parikh, G.C. Sekhar, R.S. Kumar, S. Prabakaran, J.G. Babu and R. Thomas, 2007. Diagnostic capability of optical coherence tomography (Stratus OCT 3) in early glaucoma. Ophthalmology, 114(12): 2238-2243.
Direct Link -
Poshtyar, A., J. Shanbezadeh and H. Ahmadieh, 2013. Automatic measurement of cup to disc ratio for diagnosis of glaucoma on retinal fundus images. Proceeding of the 6th International Conference on Biomedical Engineering and Informatics (BMEI 2013), pp: 24-27.
Direct Link -
Prageeth, P.G., J. David and A. Sukesh Kumar, 2011. Early detection of retinal nerve fiber layer defects using fundus image processing. Proceeding of the IEEE Recent Advances in Intelligent Computational Systems (RAICS), pp: 930-936.
Direct Link -
Raja, C. and N. Gangatharan, 2015. A Hybrid Swarm Algorithm for optimizing glaucoma diagnosis. Comput. Biol. Med., 63: 196-207.
Direct Link -
Rajan, A. and G.P. Ramesh, 2016. Comparative study of glaucomatous image classification using optical coherence tomography. Int. J. Pharm. Sci. Rev. Res., 36(1): 277-280.
Direct Link -
Ricco, S. and M. Chen, 2009. Classifiction of scan location in retinal optical coherence tomography. Proceeding of the IEEE International Symposium on Biomedical Imaging: From Nano to Macro (ISBI'09), pp: 1031-1034.
Direct Link -
Schelkens, P., A. Munteanu, J. Barbarien, M. Galca, X. Giro-Nieto and J. Cornelis, 2003. Wavelet coding of volumetric medical datasets. IEEE T. Med. Imaging, 22(3): 441-458.
Direct Link -
Schuman, J.S., T. Pedut-Kloizman, E. Hertzmark, M.R. Hee, J.R. Wilkins, J.G. Coker, C.A. Puliafito, J.G. Fujimoto and E.A. Swanson, 1996. Reproducibility of nerve fiber layer thickness measurements using optical coherence tomography. Ophthalmology, 103(11): 1889-1898.
Direct Link -
Skodras, A., C. Christopoulos and T. Ebrahimi, 2001. The JPEG 2000 still image compression standard. IEEE Signal Proc. Mag., 18(5): 36-58.
Direct Link -
Soe, N.N., J. Tian, P. Marziliano and H.T. Wong, 2014. Anterior chamber angle shape analysis and classification of glaucoma in SS-OCT images. J. Ophthalmol., 2014: 942367.
Direct Link -
Wallace, G.K., 1991. The JPEG still picture compression standard. Commun. ACM, 34(4): 30-44.
-
Wollstein, G., J.S. Schuman, L.L. Price, A. Aydin, P.C. Stark, E. Hertzmark, E. Lai, H. Ishikawa, C. Mattox, J.G. Fujimoto and L.A. Paunescu, 2005. Optical coherence tomography longitudinal evaluation of retinal nerve fiber layer thickness in glaucoma. Arch. Ophthalmol., 123(4): 464-470.
Direct Link -
Zhu, Y., P. Li, W. He, Y. Gao, Y. He and H. Ma, 2006. Layer structures localization in optical coherence tomography images. Proceeding of the IEEE International Symposium on Biophotonics, Nanophotonics and Metamaterials, pp: 50-53.
Direct Link
Competing interests
The authors have no competing interests.
Open Access Policy
This article is distributed under the terms of the Creative Commons Attribution 4.0 International License (http://creativecommons.org/licenses/by/4.0/), which permits unrestricted use, distribution, and reproduction in any medium, provided you give appropriate credit to the original author(s) and the source, provide a link to the Creative Commons license, and indicate if changes were made.
Copyright
The authors have no competing interests.
|
|
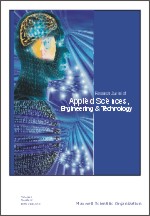 |
ISSN (Online): 2040-7467
ISSN (Print): 2040-7459 |
 |
Information |
|
|
|
Sales & Services |
|
|
|