Research Article | OPEN ACCESS
Research on Tool Wear Monitoring Method based on Project Pursuit Regression for a CNC Machine Tool
Qianjian Guo, Shanshan Yu and Lei He
Shandong Provincial Key Laboratory of Precision Manufacturing and Non-Traditional Machining, Shandong University of Technology, Zibo 255049, China
Research Journal of Applied Sciences, Engineering and Technology 2014 3:438-441
Received: February 06, 2013 | Accepted: February 25, 2013 | Published: January 20, 2014
Abstract
Tool wear is a major contributor to machining errors of a workpiece. Tool wear prediction is an effective way to estimate the wear loss in precision machining. In this study, all kinds of machining conditions are treated as the input variables, the wear loss of the tool is treated as the output variable, and Projection Pursuit Regression (PPR) algorithm is proposed to mapping the tool wear loss. Finally, a real-time prediction device is presented based on the proposed PPR algorithm, and the prediction and measurement results are found to be in satisfied agreement with average error lower than 5%.
Keywords:
CNC machine tool, online prediction, project pursuit regression, tool wear monitoring,
References
-
Dong, J.F., K.V.R. Subrahmanyam and Y.S. Wong, 2006. Bayesian-inference-based neural networks for tool wear estimation. Int. J. Adv. Manuf. Technol., 30: 797-807.
CrossRef -
Girardin, F., D. Remond and J.F. Rigal, 2010. Tool wear detection in milling: An original approach with a non dedicated sensor. Mech. Syst. Signal. Pr., 24: 1907-1920.
CrossRef -
Jacob, C.C and C.C. Joseph, 2005. An artificial-neural-networks-based in-process tool wears prediction system in milling operations. Int. J. Adv. Manuf. Technol., 25: 427-434.
CrossRef -
Luo, Y., 2004. Parametric tool wear estimation solution of HSC appropriate machining. Int. J. Adv. Manuf. Technol., 23: 546-552.
CrossRef -
Palanisamy, P., I. Rajendran and S. Shanmugasundaram, 2008. Prediction of tool wear using regression and ANN models in end-milling operations. Int. J. Mach. Tools Manuf., 37: 29-41.
CrossRef -
Quiza, R., L. Figueira and P. Davim, 2008. Comparing statistical models and artificial neural networks on predicting the tool wear in hard machining D2 AISI steel. Int. J. Adv. Manuf. Technol., 37: 641-648.
CrossRef -
Ratnam, M.M. and H.H. Shahabi, 2008. On-line monitoring of tool wear in turning operation in the presence of tool misalignment. Int. J. Adv. Manuf. Technol., 38: 718-727.
CrossRef -
Tae, J.K and W.C. Dong, 1996. Adaptive modeling of the milling process and application of a neural network for tool wear monitoring. Int. J. Adv. Manuf. Technol., 12: 5-13.
CrossRef -
Wang, X.Y., J. Li, W. Wang and Y. Huang, 2008. Design of neural network-based estimator for tool wear modeling in hard turning. J. Intell. Manuf., 19: 383-396.
CrossRef
Competing interests
The authors have no competing interests.
Open Access Policy
This article is distributed under the terms of the Creative Commons Attribution 4.0 International License (http://creativecommons.org/licenses/by/4.0/), which permits unrestricted use, distribution, and reproduction in any medium, provided you give appropriate credit to the original author(s) and the source, provide a link to the Creative Commons license, and indicate if changes were made.
Copyright
The authors have no competing interests.
|
|
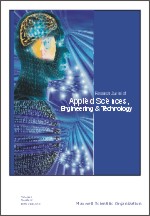 |
ISSN (Online): 2040-7467
ISSN (Print): 2040-7459 |
 |
Information |
|
|
|
Sales & Services |
|
|
|