Research Article | OPEN ACCESS
Deployment of Partitioning Around Medoids Clustering Algorithm on a Set of Objects Derived from Analytical CRM Data
J. Mbarki and E.M. Jaara
Laboratory of Computer Science Research (LARI), Faculty of Sciences, University Mohamed Ier, Oujda, Morocco
Research Journal of Applied Sciences, Engineering and Technology 2014 4:786-790
Received: April 06, 2013 | Accepted: June 25, 2013 | Published: January 27, 2014
Abstract
The aim of this study is to highlight the importance of the unsupervised learning in Data mining and CRM fields. Data mining commonly known by its acronym KDD: knowledge discovery in data base, it refers to all methods and algorithms used for data exploration or prediction in large data bases volumes, Data mining is very important in various fields such as science, business and other areas deal with a large data set. CRM: Customer Relationship Management is an integrated information system that is used to plan, schedule and control the pre-sales and post-sales activities in an organization, both CRM and data mining techniques helps organizations maximize the value of every customer interaction and drive superior corporate performance. Clustering is one of the favoured used methods in data mining: The objective of this study is to implement the clustering algorithm K-Medoids via a shell script applied on a set of Analytical CRM data stored in Teradata environment.
Keywords:
Clustering, CRM, data set, database, teradata environment,
References
-
Fayyad, U.M., G. Piatetsky Shapiro, P. Smyth and R. Uthurusamy, 1996. Advancesin Knowledge Discovery and Data Mining. AAAI Press/the MIT Press, pp: 573-592.
-
Fraley, C. and A.E. Raftery, 1998. How many clusters to which clustering method? Answers viamodel-based cluster analysis. Comput. J., 41: 578-588.
CrossRef -
Han, J. and M. Kamber, 2001. Data Mining: Concepts and Techniques. Morgan Kaufman, Boston.
-
Inmon, W.H., 1996. Building the Data Warehouse. Wiley and Sons, NY.
-
Kaufman, L. and P.J. Rousseeuw, 1987.Clusteringby Means of Medoids. In: Dodge, Y. (Ed.), Statistical Data Analysis based on the L1Norm. Elsevier/North Holland, Amsterdam, pp: 405-416.
-
Murat, E., C. Nazif and S. Sadullah, 2011. A newalgorithm forinitialcluster centersin k-meansalgorithm. Pattern Recogn. Let., 32: 1701-1705.
Competing interests
The authors have no competing interests.
Open Access Policy
This article is distributed under the terms of the Creative Commons Attribution 4.0 International License (http://creativecommons.org/licenses/by/4.0/), which permits unrestricted use, distribution, and reproduction in any medium, provided you give appropriate credit to the original author(s) and the source, provide a link to the Creative Commons license, and indicate if changes were made.
Copyright
The authors have no competing interests.
|
|
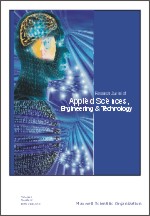 |
ISSN (Online): 2040-7467
ISSN (Print): 2040-7459 |
 |
Information |
|
|
|
Sales & Services |
|
|
|