Research Article | OPEN ACCESS
New Optimized Soft Computing Speed Controller for PMSM Drive
A. El Janati El Idrissi, N. Zahid and M. Jedra
Conception and Systems Laboratory, Faculty of Science, Mohammed V University, Agdal, AV. Ibn Battouta, Rabat, 1014RP, Morocco
Research Journal of Applied Sciences, Engineering and Technology 2014 8:1582-1586
Received: May 17, 2013 | Accepted: June 14, 2013 | Published: February 27, 2014
Abstract
This study proposes a soft computing speed controller where recurrent neural network speed controller is trained by genetic extended kalman filter, based on a predetermined genetic Proportional Integral (PI) controller. A genetic PI gives to this proposed speed controller exact values without mathematical equations, unlike the classical PI who is need for it necessary. With this proposed method the results of simulation obtained is as they are of real state of the Permanent Magnet Synchronous Motor (PMSM). The harmonic ripples of speed response are also minimized with Genetic Extended Kalman Filter (GEKF) and Neural Network Space Vector Modulation (NNSVM). This fusion between the Artificial Intelligence (AI) and optimized method of the extended kalman filter gives more superiority to the method proposed, like shows the simulations results compared to classical Neural Network Controller (NNC).
Keywords:
Direct torque control, extended kalman filter, genetic algorithm, permanent magnet synchronous motor, recurrent neural network, space vector modulation,
References
-
Bimal, K.B., 2002. Modern Power Electronics and AC Drives. Prentice Hall PTR, Upper Saddle River, NJ.
-
El Janati El Idrissi, A. and N. Zahid, 2011. GA speed and dq currents control of PMSM with vector control based space vector modulation using matlab/simulink. J. Theor. Appl. Inform. Technol., 30(1): 1-9.
-
El Janati El Idrissi, A., N. Zahid and M. Jedra, 2012. Neuro direct torque control of permanent magnet synchronous motor with genetic algorithm speed controller. J. Theor. Appl. Inform. Technol., 35(1): 1-6.
-
Elmas, C., O. Ustun and H.H. Sayan, 2008. A neuro-fuzzy controller for speed control of a permanent magnet synchronous motor drive. Exp. Syst. Appl., 34: 657-664.
CrossRef
-
Haykin, S., 2001. Kalman, Filtering and Neural Networks. John Wiley and Sons, Inc., New York, USA.
CrossRef
-
Isabelle, R. and P. Lon, 1998. A recursive algorithm based on the extended Kalman filter for the training of feedforward neural models. Neurocomputing, 20(1-3): 279-294.
CrossRef
-
Jan, R.M., C.S. Tseng and R.J. Liu, 2008. Robust PID control design for permanent magnet synchronous motor: A genetic approach. Electr. Pow. Syst. Res., 78: 1161-1168.
CrossRef
-
Lin, F.J. and S.L. Chiu, 1998. Adaptive fuzzy sliding-mode control for PM synchronous servo motor drives. IEE P-Contr. Theor. Ap., 145(1): 63-72.
-
Linkens, D.A. and H.O. Nyongesa, 2002. Learning systems in intelligent control: An appraisal of fuzzy, neural and genetic algorithm control applications. IEE P-Contr. Theor. Ap., 143(4): 367-386.
CrossRef
-
McCulloch, W.S. and W. Pitts, 1943. A logical calculus of the ideas immanent in nervous activity. Bull. Math. Biophys., 5: 115-133.
CrossRef
-
Oh, W.S., Y.T. Kim, C.S. Kim, T.S. Kwon and H.J Kim, 1999. Speed control of induction motor using genetic algorithm based fuzzy controller. Proceeding of the 25th Annual Conference of the IEEE Industrial Electronics Society (IECON, 99) 2: 625-629.
-
Ren, T.J. and T.C. Chen, 2006. Robust speed-controlled induction motor drive based on recurrent neural network. Electr. Pow. Syst. Res., 76(12): 1064-1074.
CrossRef
-
Rubioa, J.D.J. and W. Yu, 2007. Nonlinear system, Identification with recurrent neural networks and dead-zone Kalman filter algorithm. Neurocomputing, 70: 2460-2466.
CrossRef
-
Vakkas, S. and U. Metin Demirtas, 2009. Modelling and control of V/f controlled induction motor using genetic-ANFIS algorithm. Energ. Convers. Manage., 50: 786-791.
CrossRef
-
Wai, R.J., 2001. Total sliding-mode controller for PM synchronous servo motor drive using recurrent fuzzy neural network. IEEE T. Ind. Appl., 48(5): 926-944.
CrossRef
-
Wang, X. and Y. Huang, 2009. Optimized recurrent neural network-based tool wear modeling in hard turning. Trans. NAMRI/SME, 37: 213-220.
-
Wang, X. and Y. Huang, 2011. Convergence study in extended kalman filter-based training of recurrent neural networks. IEEE T. Neural. Networ., 22(4): 58-600.
-
Zadeh, L.A., 1965. Information and control. Fuzzy Set., 8: 338-353.
Competing interests
The authors have no competing interests.
Open Access Policy
This article is distributed under the terms of the Creative Commons Attribution 4.0 International License (http://creativecommons.org/licenses/by/4.0/), which permits unrestricted use, distribution, and reproduction in any medium, provided you give appropriate credit to the original author(s) and the source, provide a link to the Creative Commons license, and indicate if changes were made.
Copyright
The authors have no competing interests.
|
|
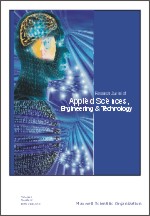 |
ISSN (Online): 2040-7467
ISSN (Print): 2040-7459 |
 |
Information |
|
|
|
Sales & Services |
|
|
|