Research Article | OPEN ACCESS
A Dynamic Effective Fault Tolerance System in Robotic Manipulator using a Hybrid Neural Network based Controller
1G. Jiji and 2M. Rajaram
1Department of ECE, PSN College of Engineering and Technology, Tirunelveli, India
2Department of EEE, Government College of Technology, Coimbatore, Tamilnadu, India
Research Journal of Applied Sciences, Engineering and Technology 2014 14:2863-2867
Received: February 02, 2013 | Accepted: March 21, 2013 | Published: April 12, 2014
Abstract
Robot manipulator play important role in the field of automobile industry, mainly it is used in gas welding application and manufacturing and assembling of motor parts. In complex trajectory, on each joint the speed of the robot manipulator is affected. For that reason, it is necessary to analyze the noise and vibration of robot's joints for predicting faults also improve the control precision of robotic manipulator. In this study we will propose a new fault detection system for Robot manipulator. The proposed hybrid fault detection system is designed based on fuzzy support vector machine and Artificial Neural Networks (ANNs). In this system the decouple joints are identified and corrected using fuzzy SVM, here non-linear signal are used for complete process and treatment, the Artificial Neural Networks (ANNs) are used to detect the free-swinging and locked joint of the robot, two types of neural predictors are also employed in the proposed adaptive neural network structure. The simulation results of a hybrid controller demonstrate the feasibility and performance of the methodology.
Keywords:
ANN, co-operation, fault tolerance, fuzzy SVM, neural network, robotics,
References
-
Dhillon, B.S. and A.R.M. Fashandi, 1997. Robotic systems probabilistic analysis. Microelectron. Reliab., 37(2): 211-224.
CrossRef -
Huang, Y.L. and D.R. Chen, 2005. Support vector machines in sonography: Application to decision making in the diagnosis of breast cancer. J. Clin. Imag., 29: 179-184.
CrossRef PMid:15855062 -
Kara, S. and F. Dirgenali, 2007. Asystem to diagnose atherosclerosis via wavelet transforms, principal component analysis and artificial neural networks. Expert Syst. Appl., 32: 632-640.
CrossRef -
Karibasappa, K. and S. Patnaik, 2004. Face recognition by ANN using wavelet transform coefficients. IE (I), J-CP, 85(2004): 17-23.
-
Kiguchi, K. and T. Fukuda, 2000. Position /force control of robot manipulators for geometrically unkown objects using neural networks. IEEE T. Ind. Electron., 47(3): 641-649.
CrossRef -
Kim, D. and J. Park, 2003. Network-based intrusion detection with support vector machines. Lect. Notes Comput. Sc., 2662: 747-756.
CrossRef -
McIntyre, M.L., W.E. Dixon, D.M. Dawson and I.D. Walker, 2005. Fault identi?cation for robot manipulators. IEEE T. Robot., 21(5): 1028-1034.
CrossRef -
Psaltis, D., A. Sideris and A.A. Yamura, 1998. A Multi layered neural network controller. IEEE Contr. Syst. Mag., 8(2): 17-21.
CrossRef -
Quero, J.M. and E.F. Camacho, 1990. Neural generalized predictive self-tuning controllers. Proceeding of IEEE International Conference on Systems Engineering. Pittsburgh, PA, USA, pp: 160-163.
CrossRef -
Schneider, H. and P.M. Frank, 2008. Observer-based supervision and fault-detection in robots using nonlinear and fuzzy logic residual evaluation. IEEE T. Contr. Syst. T., 4(3): 274-282.
CrossRef -
Vapnik, V.N., 1982. Estimation of Dependences Based on Empirical Data (Springer Series in Statistics). Springer-Verlag, New York, Inc., Secaucus, NJ, USA.
-
Vemuri, A.T. and M.M. Polycarpou, 2004. A methodology for fault diagnosis in robotic systems using neural networks. Robotica, 22(4): 419-438.
CrossRef -
Visinsky, M.L., J.R. Cavallaro and I.D. Walker, 1995. A dynamic fault tolerance framework for remote robots. IEEE T. Robotic. Autom., 11(4): 477-490.
CrossRef -
Vukobratovic, M. and A. Tuneski, 1998. Mathematical model of multiple manipulators: Cooperative compliant manipulation on dynamical environments. Mech. Mach. Theory, 33: 1211-1239.
CrossRef -
Zhang, K., H.X. Cao and H. Yan, 2006. Application of support vector machines on network abnormal intrusion detection. Appl. Res. Comput., 5: 98-100.
Competing interests
The authors have no competing interests.
Open Access Policy
This article is distributed under the terms of the Creative Commons Attribution 4.0 International License (http://creativecommons.org/licenses/by/4.0/), which permits unrestricted use, distribution, and reproduction in any medium, provided you give appropriate credit to the original author(s) and the source, provide a link to the Creative Commons license, and indicate if changes were made.
Copyright
The authors have no competing interests.
|
|
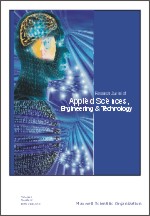 |
ISSN (Online): 2040-7467
ISSN (Print): 2040-7459 |
 |
Information |
|
|
|
Sales & Services |
|
|
|