Research Article | OPEN ACCESS
Prediction and Optimization Approaches for Modeling and Selection of Optimum Machining Parameters in CNC down Milling Operation
1, 3Asaad A. Abdullah, 1Caihua Xiong, 1Xiaojian Zhang, 1Zhuang Kejia and 2Nasseer K. Bachache
1School of Mechanical Science and Engineering
2College of Electrical and Electronic Engineering, Huazhong University of Science and Technology, Wuhan, Hubei 430074, China
3Department of Material Engineering, College of Engineering, University of Basrah, Basrah, Iraq
Research Journal of Applied Sciences, Engineering and Technology 2014 14:2908-2913
Received: May 16, 2013 | Accepted: October 19, 2013 | Published: April 12, 2014
Abstract
In this study, we suggested intelligent approach to predict and optimize the cutting parameters when down milling of 45# steel material with cutting tool PTHK- ((Ø10*20C*10D*75L) -4F-1.0R under dry condition. The experiments were performed statistically according to four factors with three levels in Taguchi experimental design method. Adaptive Neuro-fuzzy inference system is utilized to establish the relationship between the inputs and output parameter exploiting the Taguchi orthogonal array L27. The Particle Swarm Optimized-Adaptive Neuro- Fuzzy Inference System (PSOANFIS) is suggested to select the best cutting parameters providing the lower surface through from the experimental data using ANFIS models to predict objective functions. The PSOANFIS optimization approach that improves the surface quality from 0.212 to 0.202, as well as the cutting time is also reduced from 7.5 to 4.78 sec according to machining parameters before and after optimization process. From these results, it can be readily achieved that the advanced study is trusted and suitable for solving other problems encountered in metal cutting operations and the same surface roughness.
Keywords:
ANFIS, down milling process, Particle Swarm Optimization (PSO), surface roughness,
References
-
Abburi, N. and U. Dixit, 2006. A knowledge-based system for the prediction of surface roughness in turning process. Robot. Cim-Int. Manuf., 22(4): 363-372.
CrossRef -
Asiltürk, I. and H. Akkus, 2011. Determining the effect of cutting parameters on surface roughness in hard turning using the Taguchi method. Measurement, 44(9): 1697-1704.
CrossRef -
Aykut, S., M. Golcu, S. Semiz and H.S. Ergur, 2007. Modeling of cutting forces as function of cutting parameters for face milling of satellite 6 using an artificial neural network. J. Mater. Process. Tech., 190(1): 199-203.
CrossRef -
Bhattacharya, A., S. Das, P. Majumder and A. Batish, 2009. Estimating the effect of cutting parameters on surface finish and power consumption during high speed machining of AISI 1045 steel using Taguchi design and ANOVA. Prod. Engineer., 3(1): 31-40.
CrossRef -
Chavoshi, S.Z. and M. Tajdari, 2010. Surface roughness modelling in hard turning operation of AISI 4140 using CBN cutting tool. Int. J. Mater. Forming, 3(4): 233-239.
CrossRef -
Ding, T., S. Zhang, Y. Wang and X. Zhu, 2010. Empirical models and optimal cutting parameters for cutting forces and surface roughness in hard milling of AISI H13 steel. Int. J. Adv. Manuf. Tech., 51(1): 45-55.
CrossRef -
Dong, M. and N. Wang, 2011. Adaptive network-based fuzzy inference system with leave-one-out cross-validation approach for prediction of surface roughness. Appl. Math. Model., 35(3): 1024-1035.
CrossRef -
Dweiri, F., M. Al-Jarrah and H. Al-Wedyan, 2003. Fuzzy surface roughness modeling of CNC down milling of Alumic-79. J. Mater. Process. Tech., 133(3): 266-275.
CrossRef -
Fischer, W.G. and Y. Kwon, 2002. Fuzzv neuron adaptive modeling to predict surffce roughness under process variations in CNC turning. J. Manuf. Syst., 21(6): 440-450.
CrossRef -
Hsieh, H.T. and C.H. Chu, 2013. Improving optimization of tool path planning in 5-axis flank milling using advanced PSO algorithms. Robot. Cim-Int. Manuf., 29: 3-11.
-
Hwang, Y.K., C.M. Lee and S.H. Park, 2009. Evaluation of machinability according to the changes in machine tools and cooling lubrication environments and optimization of cutting conditions using Taguchi method. Int. J. Precis. Eng. Man., 10(3): 65-73.
CrossRef -
Liu, F.B., 2012. Particle swarm optimization-based algorithms for solving inverse heat conduction problems of estimating surface heat flux. Int. J. Heat Mass Tran., 55(7): 2062-2068.
CrossRef -
Moshat, S., S. Datta, A. Bandyopadhyay and P. Pal, 2010. Optimization of CNC end milling process parameters using PCA-based Taguchi method. Int. J. Eng. Sci. Technol., 2(1): 95-102.
CrossRef -
Nalbant, M., H. Gökkaya, I. Toktas and G. Sur, 2009. The experimental investigation of the effects of uncoated, PVD-and CVD-coated cemented carbide inserts and cutting parameters on surface roughness in CNC turning and its prediction using artificial neural networks. Robot. Cim-Int. Manuf., 25(1): 211-223.
CrossRef -
Robinson, J. and Y.S. Rahmat, 2004. Particle swarm optimization in electromagnetics. IEEE T. Antenn. Propag., 52(2): 397-407.
CrossRef -
Saglam, H., S. Yaldiz and F. Unsacar, 2007. The effect of tool geometry and cutting speed on main cutting force and tool tip temperature. Mater. Design, 28(1): 101-111.
CrossRef -
Sharma, V.S., S. Dhiman, R. Sehgal and S. Sharma, 2008. Estimation of cutting forces and surface roughness for hard turning using neural networks. J. Intell. Manuf., 19(4): 473-483.
CrossRef -
Tsourveloudis, N.C., 2010. Predictive modeling of the Ti6Al4V alloy surface roughness. J. Intell. Robot. Syst., 60(3): 513-530.
CrossRef -
Venkata, R.R. and P. Pawar, 2010. Parameter optimization of a multi-pass milling process using non-traditional optimization algorithms. Appl. Soft Comput., 10(2): 445-456.
CrossRef -
Yazdi, M.R.S. and S.Z. Chavoshi, 2010. Analysis and estimation of state variables in CNC face milling of AL6061. Prod. Eng. Res. Devel., 4: 535-543.
CrossRef -
Yildiz, A.R., 2013. A new hybrid differential evolution algorithm for the selection of optimal machining parameters in milling operations. Appl. Soft Comput., 13(3): 1561-1566.
CrossRef
Competing interests
The authors have no competing interests.
Open Access Policy
This article is distributed under the terms of the Creative Commons Attribution 4.0 International License (http://creativecommons.org/licenses/by/4.0/), which permits unrestricted use, distribution, and reproduction in any medium, provided you give appropriate credit to the original author(s) and the source, provide a link to the Creative Commons license, and indicate if changes were made.
Copyright
The authors have no competing interests.
|
|
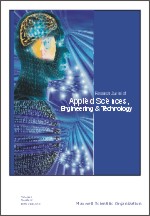 |
ISSN (Online): 2040-7467
ISSN (Print): 2040-7459 |
 |
Information |
|
|
|
Sales & Services |
|
|
|