Research Article | OPEN ACCESS
Taguchi and ANN Modeling of Turbidity Removal Using New Hybrid Flocculant
1, 2Ammar Salman Dawood and 1Yilian Li
1Environmental Engineering Department, China University of Geosciences, Wuhan, 430074, China
2College of Engineering, University of Basrah, Basrah, Iraq
Research Journal of Applied Sciences, Engineering and Technology 2014 18:3691-3698
Received: February 06, 2013 | Accepted: May 29, 2013 | Published: May 10, 2014
Abstract
In this study, aluminum chloride-poly (acrylamide-co-dimethyldiallyammonium chloride) inorganic-organic hybrid copolymer was synthesized by free radical solution polymerization. The polymerization was initiated by the redox initiation system (NH4)2S2O8 and NaHSO3 at 45°C in an aqueous medium. The AlCl3-P(AM-co- DADAAC) inorganic-organic hybrid copolymer was characterized by Fourier Transform Infrared spectroscopy (FT- IR) and Transmission Electron Microscopy (TEM). The AlCl3-P(AM-co-DADAAC) hybrid copolymer was employed to treat the turbidity of kaolin suspension. Taguchi’s experimental design method was used to determine the optimal conditions for turbidity removal. The design variables in this research were the initial concentration of kaolin suspension, pH and the AlCl3-P(AM-co-DADAAC) hybrid copolymer dosage. Taguchi Orthogonal arrays, the Signal-to-Noise (S/N) ratio and Analysis of Variance (ANOVA) were utilized to determine the optimal level and to analyze the effect of design variables in the flocculation process on the turbidity removal. ANN model was per formed to predict the final turbidity. According to the values of the error analysis and the coefficient of determination, ANN model was found that the proposed model was more appropriate to describe the turbidity reduction using the AlCl3-P(AM-co-DADAAC) hybrid copolymer in the flocculation process. The Levenberg- Marquardt Algorithm (LMA) was found to be the best of the six proposed Back Propagation (BP) algorithms with a minimum Mean Squared Error (MSE). The optimum neuron number in the hidden layer of the LMA was 12 neurons with MSE of 0.0000004438. Hence, ANN presented a very good performance for turbidity response value.
Keywords:
ANN model, ANOVA, flocculation, inorganic-organic, Taguchi, turbidity,
References
-
Aber, S., N. Daneshvar, S.M. Soroureddin, A. Chabok and K. Asadpour-Zeynali, 2007. Study of acid orange 7 removal from aqueous solutions by powdered activated carbon and modeling of experimental results by artificial neural network. Desalination, 211(1): 87-95.
CrossRef
-
Aghav, R., S. Kumar and S. Mukherjee, 2011. Artificial neural network modeling in competitive adsorption of phenol and resorcinol from water environment using some carbonaceous adsorbents. J. Hazard. Mater., 188(1): 67-77.
CrossRef PMid:21316853
-
Aleboyeh, A., M.B. Kasiri, M.E. Olya and H. Aleboyeh, 2008. Prediction of azo dye decolorization by UV/H2O2 using artificial neural networks. Dyes Pigments, 77(2): 288-294.
CrossRef
-
Baruch, I.S., G. Petia, B.C. Josefinam and F.D.A. Sebastiao, 2005. Adaptive recurrent neural network control of biological wastewater treatment. Int. J. Intell. Syst., 20(2): 173-193.
CrossRef
-
Bhatia, S., Z. Othman and A.L. Ahmad, 2007. Coagulation-flocculation process for POME treatment using Moringa oleifera seeds extract: Optimization studies. Chem. Eng. J., 133(1): 205-212.
CrossRef
-
Bhatti, M.S., D. Kapoor, R.K. Kalia, A.S. Reddy and A.K. Thukral, 2011. RSM and ANN modeling for electrocoagulation of copper from simulated wastewater: Multi objective optimization using genetic algorithm approach. Desalination, 274(1-3): 74-80.
CrossRef
-
Çelekli, A. and F. Geyik, 2011. Artificial Neural Networks (ANN) approach for modeling of removal of Lanaset Red G on Chara contraria. Bioresource Technol., 102(10): 5634-5638.
CrossRef PMid:21388806
-
Daneshvar, N., A. Khataee and N. Djafarzadeh, 2006. The use of Artificial Neural Networks (ANN) for modeling of decolorization of textile dye solution containing C. I. Basic Yellow 28 by electrocoagulation process. J. Hazard. Mater.,137(3): 1788-1795.
CrossRef PMid:16806684
-
Dawood, A.S. and Y.L. Li, 2012. Response Surface Methodology (RSM) for wastewater flocculation by a novel (AlCl3-PAM) hybrid polymer. Adv. Mater. Res., 560: p. 529-537.
CrossRef
-
Elmolla, E.S., M. Chaudhuri and M.M. Eltoukhy, 2010. The use of Artificial Neural Network (ANN) for modeling of COD removal from antibiotic aqueous solution by the Fenton process. J. Hazard. Mater., 179(1): 127-134.
CrossRef PMid:20307930
-
Gontarski, C.A., P.R. Rodrigues, M. Mori and L.F. Prenemb, 2000. Simulation of an industrial wastewater treatment plant using artificial neural networks. Comput. Chem. Eng., 24(2): 1719-1723.
CrossRef
-
Grieu, S., A. Traoré, M. Polit and J. Colprim, 2005. Prediction of parameters characterizing the state of a pollution removal biologic process. Eng. Appl. Artif. Intell., 18(5): 559-573.
CrossRef
-
Hong, S.H., M.W. Lee, D.S. Lee and J.M. Park, 2007. Monitoring of sequencing batch reactor for nitrogen and phosphorus removal using neural networks. Biochem. Eng. J., 35(3): 365-370.
CrossRef
-
Kardam, A., K.R. Raj, J.K. Arora, M.M. Srivastava and S. Srivastava, 2010. Artificial neural network modeling for sorption of cadmium from aqueous system by shelled Moringa Oleifera seed powder as an agricultural waste. J. Water Res. Protect., 2(4): 339-344.
CrossRef
-
Khataee, A.R., G. Dehghan, A. Ebadi, M. Zarei and M. Pourhassan, 2010. Biological treatment of a dye solution by Macroalgae Chara sp.: Effect of operational parameters, intermediates identification and artificial neural network modeling. Bioresource Technol.,101(7): 2252-2258.
CrossRef PMid:20022487
-
Lee, K.E., T.T. Tjoon, M. Norhashimah, T.P. Beng and F.H. Yong, 2010. Flocculation of kaolin in water using novel calcium chloride-polyacrylamide (CaCl2-PAM) hybrid polymer. Sep. Purif. Technol., 75(3): 346-351.
CrossRef
-
Lee, K.E., T.T. Tjoon, M. Norhashimah, T.P. Beng and M. Mohanapriya, 2011. Flocculation activity of novel ferric chloride-polyacrylamide (FeCl3-PAM) hybrid polymer. Desalination, 266(1): 108-113.
CrossRef
-
Lu, S., S. Lin and K. Yao, 2004. Study on the synthesis and application of starch-graft-poly (AM-co-DADMAC) by using a complex initiation system of CS-KPS. Starch-Stärke, 56(3-4): 138-143.
CrossRef
-
Machón, I., H. López, J. Rodríguez-Iglesias, E. Marañón and I. Vázquez, 2007. Simulation of a coke wastewater nitrification process using a feed-forward neuronal net. Environ. Model. Softw., 22(9): 1382-1387.
CrossRef
-
Mjalli, F.S., S. Al-Asheh and H. Alfadala, 2007. Use of artificial neural network black-box modeling for the prediction of wastewater treatment plants performance. J. Environ. Manage., 83(3): 329-338.
CrossRef PMid:16806660
-
Moral, H., A. Aksoy and C.F. Gokcay, 2008. Modeling of the activated sludge process by using artificial neural networks with automated architecture screening. Comput. Chem. Eng., 32(10): 2471-2478.
CrossRef
-
Murugan, A.A., T. Ramamurthy, B. Subramanian, C. Srinivasa Kannan and M. Ganesan, 2009. Electrocoagulation of Textile Effluent: RSM and ANN modeling. Int. J. Chem. React. Eng., 7(1).
CrossRef
-
Pai, T., Y.P. Tsai, H.M. Lo, C.H. Tsai and C.Y. Lin, 2007. Grey and neural network prediction of suspended solids and chemical oxygen demand in hospital wastewater treatment plant effluent. Comput. Chem. Eng., 31(10): 1272-1281.
CrossRef
-
Phadke, M.S., 1995. Quality Engineering using Robust Design. Prentice Hall PTR, Upper Saddle River, New Jersey.
-
Pretsch, E., P. Bühlmann and M. Badertscher, 2009. Structure Determination of Organic Compounds: Tables of Spectral Data. Springer, Berlin.
-
Raj, K.R., A. Kardam, J.K. Arora, M.M. Srivastava and S. Srivastava, 2010. Neural network modeling for Ni (II) removal from aqueous system using shelled moringa oleifera seed powder as an agricultural waste. J. Water Res. Protect., 2(4): 331-338.
CrossRef
-
Ren, N., Z. Chen, X. Wang, D. Hu and A. Wang, 2005. Optimized operational parameters of a pilot scale membrane bioreactor for high-strength organic wastewater treatment. Int. Biodeter. Biodegr., 56(4): 216-223.
CrossRef
-
Taguchi, G., E.A. Elsayed and T.C. Hsiang, 1989. Quality Engineering in Production Systems. McGraw-Hill, New York.
-
Turan, N.G., B. Mesci and O. Ozgonenel, 2011. Artificial Neural Network (ANN) approach for modeling Zn (II) adsorption from leachate using a new biosorbent. Chem. Eng. J., 173(1): 98-105.
CrossRef
-
Yang, W., J. Qian and Z. Shen, 2004. A novel flocculant of Al (OH)3-polyacrylamide ionic hybrid. J. Colloid Interf. Sci., 273(2): 400-405.
CrossRef PMid:15082374
-
Yetilmezsoy, K. and S. Demirel, 2008. Artificial Neural Network (ANN) approach for modeling of Pb (II) adsorption from aqueous solution by Antep pistachio (Pistacia Vera L.) shells. J. Hazard. Mater., 153(3): 1288-1300.
CrossRef PMid:17980484
-
Zeng, G.M., X.S. Qin, L. He, G.H. Huang, H.L. Liu and Y.P. Lin, 2003. A neural network predictive control system for paper mill wastewater treatment. Eng. Appl. Artif. Intell., 16(2): 121-129.
CrossRef
-
Zhu, Z., T. Li, J. Lu, D. Wang and C. Yao, 2009. Characterization of kaolin flocs formed by polyacrylamide as flocculation aids. Int. J. Miner. Process., 91(3): 94-99.
CrossRef
Competing interests
The authors have no competing interests.
Open Access Policy
This article is distributed under the terms of the Creative Commons Attribution 4.0 International License (http://creativecommons.org/licenses/by/4.0/), which permits unrestricted use, distribution, and reproduction in any medium, provided you give appropriate credit to the original author(s) and the source, provide a link to the Creative Commons license, and indicate if changes were made.
Copyright
The authors have no competing interests.
|
|
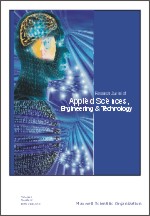 |
ISSN (Online): 2040-7467
ISSN (Print): 2040-7459 |
 |
Information |
|
|
|
Sales & Services |
|
|
|