Research Article | OPEN ACCESS
Strength Reliability Analysis of Turbine Blade Using Surrogate Models
Wei Duan, Liqiang An and Zhangqi Wang
Department of Mechanical Engineering, North China Electric Power University,
Baoding, Hebei, China
Research Journal of Applied Sciences, Engineering and Technology 2014 18:3699-3708
Received: March 08, 2013 | Accepted: April 22, 2013 | Published: May 10, 2014
Abstract
There are many stochastic parameters that have an effect on the reliability of steam turbine blades performance in practical operation. In order to improve the reliability of blade design, it is necessary to take these stochastic parameters into account. In this study, a variable cross-section twisted blade is investigated and geometrical parameters, material parameters and load parameters are considered as random variables. A reliability analysis method as a combination of a Finite Element Method (FEM), a surrogate model and Monte Carlo Simulation (MCS), is applied to solve the blade reliability analysis. Based on the blade finite element parametrical model and the experimental design, two kinds of surrogate models, Polynomial Response Surface (PRS) and Artificial Neural Network (ANN), are applied to construct the approximation analytical expressions between the blade responses (including maximum stress and deflection) and random input variables, which act as a surrogate of finite element solver to drastically reduce the number of simulations required. Then the surrogate is used for most of the samples needed in the Monte Carlo method and the statistical parameters and cumulative distribution functions of the maximum stress and deflection are obtained by Monte Carlo simulation. Finally, the probabilistic sensitivities analysis, which combines the magnitude of the gradient and the width of the scatter range of the random input variables, is applied to evaluate how much the maximum stress and deflection of the blade are influenced by the random nature of input parameters.
Keywords:
Finite element method, Monte Carlo simulation, probabilistic sensitivity analysis, reliability analysis, surrogate model, turbine blade,
References
-
Choi, S.K. and R.V. Grandhi and R.A. Canfield, 2007. Reliability-based Structural Design. Springer-Verlag, London.
-
Deng, J., D.S. Gu and X.B. Li, 2005. Structural reliability analysis for implicit performance functions using artificial neural network. Struct. Saf., 25(1): 25-48.
CrossRef
-
Disciuva, M. and D. Lomario, 2003. A comparison between Monte Carlo and FORMS in calculating the reliability of a composite structure. Comput. Struct., 59(1): 155-162.
CrossRef
-
Duan, W., 2009. Research on response surface method of strength reliability analysis for steam turbine blade. Ph.D. Thesis, North China Electric Power University, Baoding, China.
-
Duan, W. and F. Zhao, 2009. Comparative study of response surface methods for structural reliability analysis. Chin. J. Constr. Mach., 7(4): 392-394.
-
Duan, W. and Z.Q. Wang, 2010. Vibration reliability analysis of turbine blade based on ANN and Monte Carlo simulation. Proceeding of the 6th International Conference on Natural Computation. Yantai, Shandong, Aug. 22-24, pp: 1934-1939.
CrossRef
-
Elheewy, A.H., E. Meshahi and Y. Pu, 2006. Reliability analysis of structures using neural network method. Probabilist. Eng. Mech., 21(1): 44-53.
CrossRef
-
Grandhi, R.V. and L.P. Wang, 1998. Reliability-based structural optimization using improved two-point adaptive nonlinear approximations. Finite Elem. Anal. Des., 29(1): 35-48.
CrossRef
-
Haldar, A. and S. Mahadevan, 2000. Reliability Assessment using Stochastic Finite Element Analysis. John Wiley and Sons, New York.
CrossRef
-
Haykin, S., 1994. Neural Networks: A Comprehensive Foundtion. Macmillan, New York.
-
Herbert, M.G. and M.A. Armando, 2004. Comparison of response surface and neural network with other methods for structural reliability analysis. Struct. Saf., 26(1): 49-67.
CrossRef
-
Liu, Y.W. and F. Moses, 1994. A sequential response surface method and its application in the reliability analysis of aircraft structural system. Struct. Saf., 16(1-2): 39-46.
CrossRef
-
Liu, D.Y. and Q.J. Meng, 1999. Dynamic stress calculation and optimization of steam turbine blade. Proc. CSEE, 19(2): 15-20.
PMCid:PMC4688489
-
Park, J.S., 1994. Optimal latin-hypercube designs for computer experiments. J. Stat. Plan. Infer., 39(1): 95-111.
CrossRef
-
Queipo, N.V., R.T. Haftka, W. Shyy, T. Goel, R. Vaidyanathan and P.K. Tucker, 2005. Surrogate-based analysis and optimization. Prog. Aerosp. Sci., 41(1): 1-28.
CrossRef
-
Rao, C., 2002. Linear Statistical Inference and Its Applications. 2nd Edn., Wiley, New York.
-
Xie, D.M., Z.H. Liu and C.Z. Yang, 2005. Power and mechanical engineering turbo-generator twisted vibration sensitivity mechanical parameters frequency-tuning. Power Eng., 25(4): 23-29.
-
Yan, S.P., S.H. Huang and S.M. Han, 2005. Transfer matrix methods of static and dynamic Frequencies calculation for turbine blades by using Euler beam model. Proc. CSEE, 20(1): 68-71.
-
Youn, B.D. and K.K. Choi, 2004. A new response surface methodology for reliability-based design optimization. Comput. Struct., 82(2-3): 241-256.
CrossRef
Competing interests
The authors have no competing interests.
Open Access Policy
This article is distributed under the terms of the Creative Commons Attribution 4.0 International License (http://creativecommons.org/licenses/by/4.0/), which permits unrestricted use, distribution, and reproduction in any medium, provided you give appropriate credit to the original author(s) and the source, provide a link to the Creative Commons license, and indicate if changes were made.
Copyright
The authors have no competing interests.
|
|
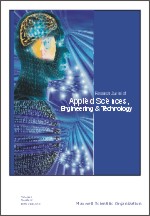 |
ISSN (Online): 2040-7467
ISSN (Print): 2040-7459 |
 |
Information |
|
|
|
Sales & Services |
|
|
|