Research Article | OPEN ACCESS
Research on Fault Diagnosis System of a Diesel Engine Based on Wavelet Analysis and LabVIEW Software
1, 2Eidam Ahmed Hebiel, 1Zhu Zhou, 1Dong Sheng Wang, 1Liu Jie, 1Mohamed Ahmed Elbashier, 1Wen Dongdong, 1Li Peng and 1Li Xiaoyu
1Department of Agricultural Engineering Automation and Measurement Technology Research Section, College of Engineering, Huazhong Agricultural University, Wuhan, China
2Department of Agricultural Engineering, Faculty of Natural Resources and Environmental Studies, University of Western Kordofan, Elnahoud, Sudan
Research Journal of Applied Sciences, Engineering and Technology 2014 18:3821-3836
Received: November 04, 2013 | Accepted: November 13, 2013 | Published: May 10, 2014
Abstract
Experiment presented in this study, used vibration data obtained from a four-stroke, 295 diesel engine. Fault of the internal-combustion engine was detected by using the vibration signals of the cylinder head. The fault diagnosis system was designed and constructed for inspecting the status and fault diagnosis of a diesel engine based on discrete wavelet analysis and LabVIEW software. The cylinder-head vibration signals were captured through a piezoelectric acceleration sensor, that was attached to a surface of the cylinder head of the engine, while the engine was running at two speeds (620 and 1300 rpm) and two loads (15 and 45 N·m). Data was gathered from five different conditions, associated with the cylinder head such as single cylinder shortage, double cylinders shortage, intake manifold obstruction, exhaust manifold obstruction and normal condition. After decomposing the vibration signals into some of the details and approximations coefficients with db5 mother wavelet and decomposition level 5, the energies were extracted from each frequency sub-band of healthy and unhealthy conditions as a feature of engine fault diagnosis. By doing so, normal and abnormal conditions behavior could be effectively distinguished by comparing the energy accumulations of each sub-band. The results showed that detection of fault by discrete wavelet analysis is practicable. Finally, two techniques, Back-Propagation Neural Network (BPNN) and Support Victor Machine (SVM) were applied to the signal that was collected from the diesel engine head. The experimental results showed that BPNN was more effective in fault diagnosis of the internal-combustion engine, with various fault conditions, than SVM.
Keywords:
Diesel engine, fault detection, internal-combustion engine, LabVIEW, vibration signal, wavelet analysis,
References
-
Addison, P.S., 2002. The Illustrated Wavelet Transform Handbook. IOP Publishing Ltd., ISBN: 0-7503-0692-0.
CrossRef
-
Amaris, A.H., C. Alonso, M.D. Florez, T.J.P. Lobos, J. Rezmer and Z. Waclawek, 2008. Application of advanced signal processing methods for accurate detection of voltage dips. Proceeding of 13th International Conference on Harmonics and Quality of Power, pp: 1-6.
CrossRef
-
Barzilay, O. and V.L. Brailovsky, 1999. On domain knowledge and feature selection using a support vector machine. Pattern Recog. Lett., 20(5): 475-484.
CrossRef
-
Biqing, W., A. Saxena, T.S. Khawaja and R. Patrick, 2004. An approach to fault diagnosis of helicopter planetary gears. Proceeding of IEEE AUTOTESTCON, pp: 475-481.
CrossRef
-
Boser, B., I. Guyon and V.N. Vapnik, 1992. A training algorithm for optimal margin classifiers. Proceedings of the 5th Annual Workshop on Computational Learning Theory, New York.
CrossRef
-
Carlucci, A.P., F. Chiara and D. Laforgia, 2006. Analysis of the relation between injection parameter variation and block vibration of an internal combustion diesel engine. J. Sound Vib., 295: 141-164.
CrossRef
-
Chandroth, G.O. and W.J. Staszewski, 1999. Fault detection in internal combustion engines using wavelet analysis. Proceedings Comadem 99. Sunderland, UK, 3: 8.
-
Chao, H., J. Xu and C. Ji, 2008. Experimental study on the knock control of HCCI engine by methanol and ethanol additives at high load. T. Chinese Soc. Agric. Mach., 3: 23-27.
-
Chebil, J., G. Noel, M. Mesbah and M. Deriche, 2009. Wavelet decomposition for the detection and diagnosis of faults in rolling element bearings. Jordan J. Mech. Ind. Eng., 3(4): 260-267.
-
Chung, Y., H. Kim and S. Choi, 2000. Flow characteristics of misfired gas in the exhaust manifold of a spark ignition engine. J. Autom. Eng., 4: 373-381.
CrossRef
-
Daubechies, I., 1992. Ten lectures on wavelets. Proceeding of CBMS-NSF Regional Conference Series in Applied Mathematics. Society for Industrial and Applied Mathematics (SIAM). Philadelphia, PA, Vol. 61.
CrossRef
-
Donoho, D., 1995. Denoising by soft thresholding. IEEE T. Inform. Theory, 4l: 6l3-627.
CrossRef
-
Donoho, D. and I. Johnstone, 1995. Adapting to unknown smoothness via wavelet shrinkage. J. Am. Stat. Assoc., 90: l200-l224.
CrossRef
-
Francois, A. and F. Patrick, 1995. Improving the readability of time frequency and time scale representations by the reassignment method. IEEE T. Signal. Process., 43: 1068-1089.
CrossRef
-
Ftoutou, E., M. Chouchane and N. Besbès, 2011. Internal combustion engine valve clearance fault classification using multivariate analysis of variance and discriminate analysis. T. I. Meas. Control, DOI: 10.1177/ 0142331211408492.
-
Girdhar, P., C. Scheffer and S. Mackay, 2004. Practical machinery vibration analysis and predictive maintenance. Newness, Elsevier, Oxford.
PMCid:PMC1304821
-
Hu, J.W. and C. Kw, 2010. Wavelet analysis of vibration signal denoising. J. Mech. Eng. Autom., 1: 128-130.
-
Hurvich, C.M. and C. Tsai, 1989. Regression and time series model selection in small samples, Biometrika, 76: 297-307.
CrossRef
-
Jiang, A., X. Li, W. Wang, X. Huang, Z. Zhang and H. Hua, 2007. Misfire failure diagnosis of engine based on wavelet analysis. T. Chinese Soc. Agric. Eng., 4: 153-157.
-
Lahouar, S., 2003. Development of data analysis algorithms for interpretation of ground-penetrating radar data. Ph.D. Thesis, Department of Elect. Eng. Virginia Tech. Blacksburg, VA.
-
Liu, Y., R. Du and Y. Shu-Zi, 1999. Internal combustion engine cylinder head vibration acceleration signal of the features and diagnostic applications. Huazhong Univ., Technol., 1: 11-15.
-
Mallat, S.G., 1998. A theory of multiresolution signals decomposition: The wavelet representation. IEEE T. Pattern Anal., 11: 647-693.
-
Merry, R.J.E., 2005. Wavelet Theory and Applications, a literature study. Eindhoven University of Technology, Control Systems Technology Group. Eindhoven, DCT 2005.53.
-
Mingzan, W., J. Qie, Q. Gu and H. Shao, 2003. Wavelet package-neural network based on rough set diesel engine vibration signal identification model. Proceedings of the International Conference on Neural Networks and Signal Processing, pp: 185-190.
CrossRef
-
Misiti, M., Y. Misity, G. Oppenheim and J.M. Poggi, 1996. Wavelet Toolbox User's Guide. MathWorks.
-
Modgil, G., R.F. Orsagh and M.J. Roemer, 2004. Advanced vibration diagnostics for engine test cells. Proceedings of IEEE Aerospace Conference, pp: 3361-3371.
CrossRef
-
Parlak, A., H. Yasar, C. Hasimoglu and A. Kolip, 2005. The effects of injection timing on NOx emissions of a low heat rejection indirect diesel injection engine. Appl. Therm. Eng., 25: 3042-3052.
CrossRef
-
Peretto, L., R. Sasdelli and R. Tinarelli, 2005. On uncertainty in wavelet based signal analysis. IEEE T. Instrum. Meas., 54(4): 1593-1599.
CrossRef
-
Sanchez, S.G., N.G. Prelcic and S.J.G. Galan, 1996. Uvi Wave-wavelet Toolbox for Matlab, Ver 3.0, University of Vigo, [Online]. Apr.
Direct Link
-
Vapnik, V., S. Golowich and A. Smola, 1996. Support vector method for function approximation, regression estimation and signal processing. Adv. Neur. In., 9: 281-287.
-
Villarino, R. and J. Bohme, 2004. Pressure reconstruction and misfire detection from multichannel structure-borne sound. Proceeding of IEEE International Conference on Acoustics, Speech and Signal Processing (ICASSP'04), pp: 141-144.
CrossRef
-
Wang, Y. and L. Zhou, 2003. Investigation of the detection of knock and misfire of a spark ignition engine with the ionic current method. P. I. Mech. Eng. D-J. Aut., 217(7): 617-621.
-
Wang, C., Z. Zhong and Y. Zhang, 2008. Fault diagnosis for diesel valve trains based on time-frequency images. Mech. Syst. Signal Pr., 22: 1981-1983.
CrossRef
-
Wu, T.K., S.C. Huang and Y.R. Meng, 2008. Evaluation of ANN and SVM classifiers as predictors to the diagnosis of student with learning disability. Expert Syst. Appl., 34(3): 1846-1856.
CrossRef
-
Ying, D., H. Yigang and S. Yichuang, 2010. Fault diagnosis of analog circuits with tolerances using artificial neural networks. Proceeding of IEEE Asia-Pacific Conference on Circuits and Systems, (APCCAS), pp: 292-295.
-
Zhen, W., L. Ji, D. Zijia and S. Yanhui, 2004. Non-stationary Fault Diagnosis based on Local-wave Neural Network. In: Yin, F., J. Wang and C. Guo (Eds.), ISNN 2004. LNCS 3174, Springer-Verlag, Berlin, Heidelberg, pp: 549-554.
Competing interests
The authors have no competing interests.
Open Access Policy
This article is distributed under the terms of the Creative Commons Attribution 4.0 International License (http://creativecommons.org/licenses/by/4.0/), which permits unrestricted use, distribution, and reproduction in any medium, provided you give appropriate credit to the original author(s) and the source, provide a link to the Creative Commons license, and indicate if changes were made.
Copyright
The authors have no competing interests.
|
|
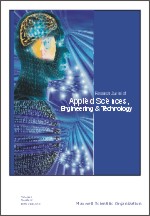 |
ISSN (Online): 2040-7467
ISSN (Print): 2040-7459 |
 |
Information |
|
|
|
Sales & Services |
|
|
|