Research Article | OPEN ACCESS
Comparative Study of MLP and RBF Neural Networks for Estimation of Suspended Sediments in Pari River, Perak
M.R. Mustafa and M.H. Isa
Department of Civil Engineering, Universiti Teknologi PETRONAS, 31750 Tronoh, Perak, Malaysia
Research Journal of Applied Sciences, Engineering and Technology 2014 18:3837-3841
Received: November 06, 2013 | Accepted: November 18, 2013 | Published: May 10, 2014
Abstract
Estimation of suspended sediments in rivers using soft computing techniques has been extensively performed around the world since 1990’s. However, accuracy in the results was always found to be highly desired and a profound crucial task. This study presents a thorough comparison between the performances of best basis function of Radial Basis Functions (RBF) and the best training algorithm in Multilayer Perceptron (MLP) neural networks for prediction of suspended sediments in Pari River, Perak, Malaysia. Time series data of water discharge and suspended sediments was used to develop MLP and RBF models. A comparison between six basis functions was performed to identify the most appropriate and best basis function for the selected time series of the river’s data. The performance of the models was compared using several statistical measures including coefficient of determination, coefficient of efficiency and mean absolute error. The performance of the best RBF function was compared with the previously identified best training algorithm of MLP neural networks. The results showed that comparison of various basis functions is always advantageous to achieve the most appropriate basis function for the accurate prediction of the time series data. The results also showed that the performances of both particular RBF and MLP models were close to each other and capable to capture the exact pattern of the sediment data in the river. However, the RBF model showed some inconsistency while predicting the time series data. Furthermore, RBF modeling required more investigation to choose appropriate value for the predefined parameters as compared to MLP modeling.
Keywords:
Artificial neural networks, discharge, evaluation, prediction, sediment,
References
-
Alp, M. and H.K. Cigizoglu, 2007. Suspended sediment load simulation by two artificial neural network methods using hydrometeorological data. Environ. Modell. Softw., 22: 2-13.
CrossRef
-
Cigizoglu, H.K., 2004. Estimation and forecasting of daily suspended sediment data by multi-layer perceptrons. Adv. Water Resour., 27: 185-195.
CrossRef
-
Cigizoglu, H.K. and M. Alp, 2006. Generalized regression neural network in modelling river sediment yield. Adv. Eng. Softw., 37: 63-68.
CrossRef
-
Jain, S.K., 2001. Development of integrated sediment rating curves using ANNs. J. Hydraul. Eng. ASCE, 127: 30-37.
CrossRef
-
Kakaei Lafdani, E., A. Moghaddam Nia and A. Ahmadi, 2013. Daily suspended sediment load prediction using artificial neural networks and support vector machines. J. Hydrol., 478: 50-62.
CrossRef
-
Kisi, O., 2005. Suspended sediment estimation using neuro-fuzzy and neural network approaches. Hydrol. Sci. J., 50: 683-696.
CrossRef
-
Kisi, O., 2008. Constructing neural network sediment estimation models using a data-driven algorithm. Math. Comput. Simulat., 79: 94-103.
CrossRef
-
Kisi, O., I. Yuksel and E. Dogan, 2008. Modelling daily suspended sediment of rivers in Turkey using several data-driven techniques/Modélisation de la charge journalière en matières en suspension dans des rivières turques à l'aide de plusieurs techniques empiriques. Hydrol. Sci. J., 53: 1270-1285.
CrossRef
-
Liu, Q.J., Z.H. Shi, N.F. Fang, H.D. Zhu and L. Ai, 2013. Modeling the daily suspended sediment concentration in a hyperconcentrated river on the loess Plateau, China, using the wavelet-ANN approach. Geomorphology, 186: 181-190.
CrossRef
-
Long, C.M. and T.M. Pavelsky, 2013. Remote sensing of suspended sediment concentration and hydrologic connectivity in a complex wetland environment. Remote Sensing Environ., 129: 197-209.
CrossRef
-
Melesse, A.M., S. Ahmad, M.E. McClain, X. Wang and Y.H. Lim, 2011. Suspended sediment load prediction of river systems: An artificial neural network approach. Agr. Water Manage., 98: 855-866.
CrossRef
-
Mustafa, M.R., M.H. Isa and R.B. Rezaur, 2011a. A comparison of artificial neural networks for prediction of suspended sediment discharge in river-a case study in Malaysia. World Acad. Sci. Eng. Technol., 81: 372-376.
-
Mustafa, M.R., M.H. Isa and R.R. Bhuiyan, 2011b. Prediction of river suspended sediment load using radial basis function neural network-a case study in Malaysia. Proceeding of National Postgraduate Conference (NPC), pp: 1-4.
CrossRef
-
Mustafa, M.R., M.H. Isa and R.B. Rezaur, 2012a. Artificial neural networks modeling in water resources engineering: Infrastructure and applications international. World Acad. Sci. Eng. Technol., 62: 341-349.
-
Mustafa, M.R., R.B. Rezaur, H. Rahardjo and M.H. Isa, 2012b. Prediction of pore-water pressure using radial basis function neural network. Eng. Geol., 135-136: 40-47.
CrossRef
-
Mustafa, M.R., R.B. Rezaur, S. Saiedi and M.H. Isa, 2012c. River suspended sediment prediction using various multilayer perceptron neural network training algorithms: A case study in Malaysia. Water Resour. Manag., 26: 1879-1897.
CrossRef
-
Mustafa, M.R., R.R. Bhuiyan, M.H. Isa, S. Saiedi and H. Rahardjo, 2012d. Effect of antecedent conditions on prediction of pore-water pressure using artificial neural networks. Modern Appl. Sci., 6: 6-15.
CrossRef
-
Mustafa, M., R. Rezaur, S. Saiedi, H. Rahardjo and M. Isa, 2013. Evaluation of MLP-ANN training algorithms for modeling soil pore-water pressure responses to rainfall. J. Hydrol. Eng., 18: 50-57.
CrossRef
-
Nagy, H.M., K. Watanabe and M. Hirano, 2002. Prediction of sediment load concentration in rivers using artificial neural network model. J. Hydraul. Eng-ASCE., 128: 588-595.
CrossRef
-
Sinnakaudan, S.K., A. Ab. Ghani, M.S.S. Ahmad and N.A. Zakaria, 2003. Flood risk mapping for pari river incorporating sediment transport. J. Environ. Model. Software, 18(2): 119-130.
CrossRef
Competing interests
The authors have no competing interests.
Open Access Policy
This article is distributed under the terms of the Creative Commons Attribution 4.0 International License (http://creativecommons.org/licenses/by/4.0/), which permits unrestricted use, distribution, and reproduction in any medium, provided you give appropriate credit to the original author(s) and the source, provide a link to the Creative Commons license, and indicate if changes were made.
Copyright
The authors have no competing interests.
|
|
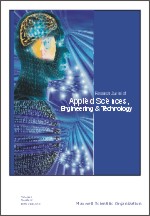 |
ISSN (Online): 2040-7467
ISSN (Print): 2040-7459 |
 |
Information |
|
|
|
Sales & Services |
|
|
|