Research Article | OPEN ACCESS
A Survey on Utilization of the Machine Learning Algorithms for the Prediction of Erythemato Squamous Diseases
N. Badrinath and G. Gopinath
Department of Computer Engineering and Applications, Bharathidasan University,
Tiruchirapalli, Tamil Nadu, India
Research Journal of Applied Sciences, Engineering and Technology 2014 18:3883-3887
Received: November 22, 2014 | Accepted: December 17, 2013 | Published: May 10, 2014
Abstract
The aim of this study list the contributions of various machine learning algorithms for the prediction of Erythemato Squamous Diseases (ESDs) and it is very useful for the budding researchers to do research in this field. In the advent of ozone depletion the ultra violet radiation is the major cause of many skin diseases, which are leading to skin cancer. Early detection of skin cancer is more important to avoid human loses and especially the white skinned people are more affected. The Asian and African race people are less affected as they have melanin in their skin. The American’s are directly and more widely affected by the ozone depletion, due to this ESD, which is predominant among the skin diseases. Due to technology advancements a large amount of data are deposited. In these data the information is hidden as raw data and with latest methodologies and technologies like Data Mining, neural networks, fuzzy systems, Genetic and Evolutionary computing a pattern can be evolved to study them. Guvenir et al. (1998) studied about ESDs and contributed 366 patients data with 34 features consisting of clinical and histopathological data in the dermatology dataset (The data taken from School of Medicine in Gazi University and the department of Computer Science in Bilkent University, Turkey; and it is available in the URL (http://archive.ics.uci.edu/ml/datasets/Dermatology) in the year 1998. This survey study gives a brief description about the contribution of what in the field of ESDs in Chronological order from the year 1998 till 2013. In this study we intend to contribute various machine learning algorithms dealing with ESDs.
Keywords:
AdaBoost, Adaptive Neuro-Fuzzy Interference Systems (ANFIS), ESD, Extreme Learning Machine (ELM), fuzzy based ELM, fuzzy logic, preprocessing techniques, Support Vector Machine (SVM),
References
-
Abdel-Aal, R.E., M.R. Abdel-Halim and S. Abdel-Aal, 2006. Improving the classification of multiple disorders with problem decomposition. J. Biomed. Inform., 39(6): 612-625.
CrossRef PMid:16442851
-
Abdi, M.J. and D. Giveki, 2013. Automatic detection of erythemato-squamous diseases using PSO-SVM based on association rules. Eng. Appl. Artif. Intel., 26: 603-608.
CrossRef
-
Abu Naser, S.S. and A.N. Akkila, 2008. A proposed expert system for skin diseases diagnosis. J. Appl. Sci. Res., 4(12):1682-1693.
-
Agarwal, P.K., 1997. Pattern of skin diseases in the Al Jouf region. Ann. Saudi Med., 17: 112-114.
CrossRef PMid:17377479
-
Akin, O. and G. Arif, 2012. A robust multi-class feature selection strategy based on rotation forest ensemble algorithm for diagnosis of erythemato-squamous diseases. J. Med. Syst., 36: 941-949.
CrossRef PMid:20703639
-
Akin, Ö. and G. Arif, 2013. Genetic algorithm wrapped Bayesian network feature selection applied to differential diagnosis of erythemato-squamous diseases. Digit. Signal Process., 23: 230-237.
CrossRef
-
Al-Zoman, A.Y., M.D. Facharizt and A.K. Al-Asmair, 2008. Pattern of skin diseases at Riyadh military hospital. Egypt. Dematol. Online J., 4(2-4): 01-10.
-
Aruna, S., L.V. Nandakishore and S.P. Rajagopalan, 2012. A hybrid feature selection method based on IGSBFS and Naïve Bayes for the diagnosis of erythemato-squamous disease. Int. J. Comput. Appl., 41(7): 114-123.
CrossRef
-
Badrinath, N., G. Ganapathy and K.S. Ravichandran, 2013. Estimation of automatic detection of erythemato-squamous diseases through Adaboost and its hybrid classifiers. J. Artif. Intell. Rev., (under review).
CrossRef
-
Castellano, G., C. Castiello, A.M. Fanelli and C. Leone, 2003. Diagnosis of dermatological diseases by a neuro-fuzzy system. Proceedings of the 3rd Conference of the European Society for Fuzzy Logic and Technology. Zittau, Germany, September 10-12, 2003.
PMCid:PMC1298953
-
Davar, G., S. Hamid, A.B. Amir and K. Younes, 2011. Detection of erythemato-squamous diseases using AR-Catfish BPSO-KSVM. Signal Image Process. Int. J., 2(4): 57-72.
CrossRef
-
Fabien, M. and U. Alfred, 2005. U*F Clustering: A New Performant Cluster-Mining method based on Segmentation of Self-Organizing Maps. Proceeding of the 5th Workshop on Self-Organizing Maps (WSOM'2005), Paris: France, pp: 25-31.
-
Francesco, G., 2011. Instance-based classi?ers applied to medical databases: Diagnosis and knowledge extraction. Arti?. Intell. Med., 52: 123-139.
CrossRef PMid:21621400
-
Guvenir, H.A. and N. Emeksiz, 2000. An expert system for the differential diagnosis of erythemato-squamous diseases. Expert Syst. Appl., 18: 43-49.
CrossRef
-
Guvenir, H.A., G. Demiroz and N. Ilter, 1998. Learning differential diagnosis of erythemato-squamous diseases using voting feature intervals. Artif. Intell. Med., 13: 147-165.
CrossRef
-
Juanying, X. and W. Chunxia, 2011. Using support vector machines with a novel hybrid feature selection method for diagnosis of Erythemato-squamous diseases. Expert Syst. Appl., 38: 5809-5815.
CrossRef
-
Juanying, X., X. Weixin, W. Chunxia and G. Xinbo, 2010. A novel hybrid feature selection method based on IFSFFS and SVM for the diagnosis of erythemato-squamous diseases. Proceeding of JMLR: Workshop and Conference, pp: 142-151.
-
Juanying, X., L. Jinhu, X. Weixin, S. Yong and L. Xiaohui, 2013. Two-stage hybrid feature selection algorithms for diagnosing erythemato-squamous diseases. Health Inform. Sci. Syst., pp: 1-10.
-
Kenneth, R., G. Florin, S. Abdel-Badeeh and E.S. El-Dahshan, 2009. Evaluation of the feature space of an erythemato squamous dataset using rough sets. Math. Comp. Sci. Ser., 36(2): 123-130.
-
Lekkas, S. and L. Mikhailov, 2010. Evolving fuzzy medical diagnosis of Pima Indians diabetes and of dermatologica diseases. Artif. Intell. Med., 50: 117-126.
CrossRef PMid:20566274
-
Lopes, C.C., H.S. Freitas and A.A. Bojarczuk, 2001. Data mining with constrained-syntax genetic programming: Applications in medical data set. Proceeding of Data Analysis in Medicine and Pharmacology (IDAMAP-2001), a Workshop at Medinfo-2001, London, UK, 2001.
-
Loris, N., 2006. An ensemble of classi?ers for the diagnosis of erythemato-squamous diseases. Neuro Comput., 69: 842-845.
-
Nani, L., 2006. An ensemble of classifiers for the diagnosis of erythemato-squamous diseases. Neurocomputing, 69: 842-845.
CrossRef
-
Polat, K. and S. Gunes, 2009. A novel hybrid intelligent method based on C4. 5 decision tree classifiers and one-against-all approach for multi-class classification problems. Expert Syst. Appl., 36(2): 1587-1592.
CrossRef
-
Raddadi, A.A., S.A. Abdullah and Z.B. Damanhouri, 1999. Pattern of skin diseases at King Khalid National Guard hospital: A 12-month prospective study. Ann. Saudi Med., 19(5): 453-454.
CrossRef PMid:17277519
-
Ravichandran, K.S., B. Narayanamurthy, G. Ganapathy, S. Ravalli and J. Sindhura, 2013. An efficient approach to an automatic detection of erythemato-squamous diseases. Neural Comput. Appl., DOI: 10.1007/s00521-013-1452-5.
CrossRef
-
Übeyli, E.D., 2009. Combined neural networks for diagnosis of erythemato-squamous diseases. Expert Syst. Appl., 36: 5107-5112.
CrossRef
-
Ubeyli, E.D. and G. Inan, 2005. Automatic detection of erythemato-squamous diseases using adaptive neuro-fuzzy inference systems. Comput. Bio. Med., 35: 421-433.
CrossRef PMid:16136651
-
Übeyli, E.D. and D. Erdogan, 2010. Automatic detection of erythemato-squamous diseases using k-means clustering. J. Med. Syst., 34:179-184.
CrossRef PMid:20433056
-
Xie, J. and C. Wang, 2011. Using support vector machines with a novel hybrid feature selection method for diagnosis of erythemato-squamous diseases. Expert Syst. Appl., 38(5): 5809-5815.
CrossRef
-
Zhu, S., T. Li and M. Ogihara, 2002. An algorithm for non-distance based clustering in high dimensional spaces. Lect. Notes Comput. Sci., 2454: 52-62.
CrossRef
Competing interests
The authors have no competing interests.
Open Access Policy
This article is distributed under the terms of the Creative Commons Attribution 4.0 International License (http://creativecommons.org/licenses/by/4.0/), which permits unrestricted use, distribution, and reproduction in any medium, provided you give appropriate credit to the original author(s) and the source, provide a link to the Creative Commons license, and indicate if changes were made.
Copyright
The authors have no competing interests.
|
|
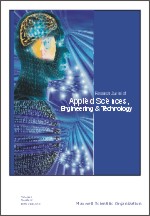 |
ISSN (Online): 2040-7467
ISSN (Print): 2040-7459 |
 |
Information |
|
|
|
Sales & Services |
|
|
|