Research Article | OPEN ACCESS
Biological Nitrogen Removal Process Monitoring Based on Fuzzy Robust PCA
1Nabila Heloulou, 1Messaoud Ramdani and 2Abdenabi Abidi
1Department of Electronics
2Department of Engineering, Laboratoire d'Automatique et Signaux de Annaba (LASA),
Universit
Research Journal of Applied Sciences, Engineering and Technology 2014 21:4434-4444
Received: November 26, 2013 | Accepted: January 16, 2014 | Published: June 05, 2014
Abstract
In this study the Fuzzy Robust Principal Component Analysis (FRPCA) method is used to monitor a biological nitrogen removal process, performances of this method are then compared with classical principal component analysis. The obtained results demonstrate the performances superiority of this robust extension compared with the conventional one. In this method fuzzy variant of PCA uses fuzzy membership and diminish the effect of outliers by assigning small membership values to outliers in order to make it robust. For the purpose of fault detection, the SPE index is used. Then the fault localization by contribution plots approach and SVI index are exploited.
Keywords:
Biological nitrogen removal, fault diagnosis, fuzzy robust PCA, multivariate statistical process control, process monitoring, water treatment plant,
References
-
Aguado, D. and C. Rosen, 2008. Multivariate statistical monitoring of continuous wastewater treatment plants. Eng. Appl. Artif. Intel., 21(7): 1080-1091.
CrossRef -
Alcala, C.F. and S.J. Qin, 2009. Reconstruction-based contribution for process monitoring. Automatica, 45(7): 1593-1600.
CrossRef -
Corona, F., M. Mulas, H. Haimi, L. Sundell, M. Heinonen and R. Vahala, 2013. Monitoring nitrate concentrations in the denitrifying post-filtration unit of a municipal wastewater treatment plant. J. Process Contr., 23(2): 158-170.
CrossRef -
Dunia, R., S.J. Qin, T.F. Edgar and T.J. McAvoy, 1996. Use of principal components Analysis for sensor fault identification. Comput. Chem. Eng., 20: 713-718.
CrossRef -
Gomez-Quintero, C., I. Queinnec and J.P. Babary, 2000. A reduced nonlinear model of an activated sludge process. Proceeding of International Symposium on Advanced Control of Chemical Processes, 2: 1037-1402.
CrossRef -
Haimi, H., M. Mulas, F. Corona and R. Vahala, 2013. Data-derived soft-sensors for biological wastewater treatment plants: An overview. Environ. Model. Software, 47: 88-107.
CrossRef -
Henze, M., C.P. Leslie Grady, W. Gujer, G.V.R. Maris and T. Matsuo, 1987. Activated sludge process model No. 1. Technical Report IAWQ Scienti?c and Technical Report 1.
-
Jackson, J.E., 1991. A User's Guide to Principal Components. John Wiley and Sons, Inc., New York.
CrossRef -
Jolliffe, L.T., 1986. Principal Component Analysis. Springer Verlag, New York.
CrossRef PMCid:PMC1960413 -
Kim, Y.M., H.U. Cho, D.S. Lee, D. Park and J.M. Park, 2011. Influence of operational parameters on nitrogen removal efficiency and microbial communities in a fullscale activated sludge process. Water Res., 45: 5785-5795.
CrossRef PMid:21924454 -
Lee, J.M., C. Yoo and I.B. Lee, 2004b. Statistical monitoring of dynamic processes based on dynamic independent component analysis. Chem. Eng. Sci., 59: 2995-3006.
CrossRef -
Lee, J.M., C. Yoo, S.W. Choi, P.A. Vanrolleghem and I.B. Lee, 2004a. Nonlinear process monitoring using kernel principal component analysis. Chem. Eng. Sci., 59: 223-234.
CrossRef -
Lopez-Arenas, T., A. Pulis and R. Baratti, 2004. On-line monitoring of a biological for wastewater treatment. Rev. Mex. Ing. Quim., 3: 51-63.
-
Luukka, P., 2009. Classification based on fuzzy robust PCA algorithms and similarity classifier. Expert Syst. Appl., 36: 7463-7468.
CrossRef -
Moon, T.S., Y.J. Kim, J.R. Kim, J.H. Cha, D.H. Kim and C.M. Kim, 2009. Identification of process operating state with operational map in municipal wastewater treatment plant. J. Environ. Manage., 90: 772-778.
CrossRef PMid:18313199 -
Oja, E., 1982. A simplified neuron model as a principal component analyzer. J. Math. Biol., 15: 267-273.
CrossRef PMid:7153672 -
Oja, E., 1995. The nonlinear PCA learning rule and signal separation-mathematical analysis. Technical Report A 26, Helsinki University of Technology.
PMCid:PMC157457 -
Oja, E. and J. Karhunen, 1985. On stochastic approximation of the eigenvectors and eigenvalues of the expectation of a random matrix. J. Math Anal. Appl., 106: 69-84.
CrossRef -
Runger, G.C. and F.B. Alt, 1996. Choosing principal components for multivariate statistical process control. Commun. Stat-Theor. M., 25(5): 909-922.
-
Tomita, R.K., S.W. Park and O.A.Z. Sotomayor, 2002. Analysis of activated sludge process using multivariate statistical tools-a PCA approach. Chem. Eng. J., 90: 283-290.
CrossRef -
Wang, D. and J.A. Romagnoli, 2005. Robust multi-scale principal components analysis with applications to process monitoring. J. Process Contr., 15(8): 869-882.
CrossRef -
Wold, S., K. Esbensen and P. Geladi, 1987. Principal component analysis. Chemometr. Intell. Lab., 2: 37-52.
CrossRef -
Xu, L. and L. Yuille, 1995. Robust principal compoenent analysis by self-organizing rules based on statistical physics approach. IEEE T. Neural Networ., 6(1): 131-143.
CrossRef PMid:18263293 -
Yang, T.N. and S.D. Wang, 1999. Robust algorithms for principal component analysis. Pattern Recogn. Lett., 20: 927-933.
CrossRef -
Yoo, C., P.A. Vanrolleghem and I.B. Lee, 2003. Nonlinear modeling and adaptive monitoring with fuzzy and multivariate statistical methods in biological wastewater treatment plants. J. Biotechnol., 105: 135-163.
CrossRef -
Zhao, L.J., T.Y. Chai and Q.M. Cong, 2004. Multivariate statistical modeling and monitoring of SBR wastewater treatment using double moving window PCA. Proceeding of the International Conference on Machine Learning and Cybernetics, pp: 1371-1376.
Competing interests
The authors have no competing interests.
Open Access Policy
This article is distributed under the terms of the Creative Commons Attribution 4.0 International License (http://creativecommons.org/licenses/by/4.0/), which permits unrestricted use, distribution, and reproduction in any medium, provided you give appropriate credit to the original author(s) and the source, provide a link to the Creative Commons license, and indicate if changes were made.
Copyright
The authors have no competing interests.
|
|
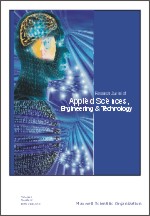 |
ISSN (Online): 2040-7467
ISSN (Print): 2040-7459 |
 |
Information |
|
|
|
Sales & Services |
|
|
|