Research Article | OPEN ACCESS
Swarm Intelligence Approach Based on Adaptive ELM Classifier with ICGA Selection for Microarray Gene Expression and Cancer Classification
1T. Karthikeyan and 2R. Balakrishnan
1P.S.G. College of Arts and Science
2Dr.NGP Arts and Science College, Coimbatore, India
Research Journal of Applied Sciences, Engineering and Technology 2014 21:4445-4454
Received: December 09, 2013 | Accepted: January 11, 2014 | Published: June 05, 2014
Abstract
The aim of this research study is based on efficient gene selection and classification of microarray data analysis using hybrid machine learning algorithms. The beginning of microarray technology has enabled the researchers to quickly measure the position of thousands of genes expressed in an organic/biological tissue samples in a solitary experiment. One of the important applications of this microarray technology is to classify the tissue samples using their gene expression representation, identify numerous type of cancer. Cancer is a group of diseases in which a set of cells shows uncontrolled growth, instance that interrupts upon and destroys nearby tissues and spreading to other locations in the body via lymph or blood. Cancer has becomes a one of the major important disease in current scenario. DNA microarrays turn out to be an effectual tool utilized in molecular biology and cancer diagnosis. Microarrays can be measured to establish the relative quantity of mRNAs in two or additional organic/biological tissue samples for thousands/several thousands of genes at the same time. As the superiority of this technique become exactly analysis/identifying the suitable assessment of microarray data in various open issues. In the field of medical sciences multi-category cancer classification play a major important role to classify the cancer types according to the gene expression. The need of the cancer classification has been become indispensible, because the numbers of cancer victims are increasing steadily identified by recent years. To perform this proposed a combination of Integer-Coded Genetic Algorithm (ICGA) and Artificial Bee Colony algorithm (ABC), coupled with an Adaptive Extreme Learning Machine (AELM), is used for gene selection and cancer classification. ICGA is used with ABC based AELM classifier to chose an optimal set of genes which results in an efficient hybrid algorithm that can handle sparse data and sample imbalance. The performance of the proposed approach is evaluated and the results are compared with existing methods.
Keywords:
Adaptive extreme learning machine, artificial bee colony algorithm, biology and genetics, classifier design and evaluation, feature evaluation and selection,
References
-
Alba, E., J. Garcia-Nieto, L. Jourdan and E. Talbi, 2007. Gene selection in cancer classification using PSO/SVM and GA/SVM hybrid algorithms. Proceeding of IEEE Congress on Evolutionary Computation (CEC, 2007), pp: 284-290.
-
Ein-Dor, L., O. Zuk and E. Domany, 2006. Thousands of samples are needed to generate a robust gene list for predicting outcome in cancer. P. Natl. Acad. Sci. USA, 103(15): 5923-5928.
CrossRef PMid:16585533 PMCid:PMC1458674 -
Eisen, M. and P. Brown, 1999. DNA arrays for analysis of gene expression. Method. Enzymol., 303: 179-205.
CrossRef -
Eisen, M.B., P.T. Spellman, P.O. Brown and D. Bostein, 1998. Cluster analysis and display of genome-wide expression patterns. P. Natl. Acad. Sci. USA, 95: 14863-14868.
CrossRef PMid:9843981 PMCid:PMC24541 -
Golub, T.R., D.K. Slonim, P. Tamayo, C. Huard, M. Gaasenbeek, J.P. Mesirov, H. Coller, M.L. Loh, J.R Downing, M.A. Caligiuri, C.D. Bloomfield and E.S. Lander, 1999. Molecular classification of cancer: Class discovery and class prediction by gene expression monitoring. Science, 286(5439): 531-537.
CrossRef PMid:10521349 -
Guo, Z., J. Wu, H. Lu and J. Wang, 2011. A case study on a hybrid wind speed forecasting method using BP neural network. Knowl-Based Syst., 24(7): 1048-1056.
CrossRef -
Jia, J. and S. Hao, 2013. Water demand forecasting based on adaptive extreme learning machine. Proceeding of 2013 International Conference on Artificial Intelligence and Software Engineering, ISBN: 978-90-78677-71-0.
CrossRef -
Karaboga, D. and B. Basturk, 2007. Artificial Bee Colony (ABC) Optimization Algorithm for Solving Constrained Optimization Problems. LNCS: Advances in Soft Computing: Foundations of Fuzzy Logic and Soft Computing, Springer-Verlag, 4529: 789-798.
CrossRef -
Kavipriya, P. and C. Gomathy, 2013. An efficient dynamic orthogonal variable spreading factor code allocation approach in WCDMA through swarm intelligence technique. Int. J. Eng. Technol. (IJET), 5(5): 3828-3838.
-
Koller, D. and M. Sahami, 1996. Toward optimal feature selection. Proceeding of 13th International Conference on Machine Learning, pp: 284-292.
-
Liao, C., S. Li and Z. Luo, 2006. Gene selection for cancer classification using wilcoxon rank sum test and support vector machine. Proceeding of International Conference on Computational Intelligence and Security, pp: 368-373.
CrossRef -
Lipshutz, R., S. Fodor, T. Gingeras and D. Lockhart, 1999. High density synthetic oligonucleotide arrays. Nat. Genet., 21: 20-24.
CrossRef PMid:9915496 -
Michalewicz, Z., 1994. Genetic Algorithm + Data Structures = Evolution Programs. 3rd Edn., Springer-Verlag, New York, pp: 18-22.
CrossRef -
Peng, S., Q. Xu, X.B. Ling, X. Peng, W. Dua and L. Chen, 2003. Molecular classification of cancer types from microarray data using the combination of genetic algorithms and support vector machine. FEBS Lett., 555(2): 358-362.
CrossRef -
Piatetsky-Shapiro, G. and P. Tamayo, 2003. Microarray data mining: Facing the challenges. SIGKDD Explorations, 5(2): 1-5.
CrossRef -
Ramaswamy, S., P. Tamayo, R. Rifkin, S. Mukherjee, C.H. Yeang, M. Angelo, C. Ladd, M. Reich, E. Latulippe, J.P. Mesirov, T. Poggio, W. Gerald, M. Loda, E.S. Lander and T.R. Golub, 2001. Multiclass cancer diagnosis using tumor gene expression signatures. P. Natl. Acad. Sci. USA, 98(26): 15149-15154.
CrossRef PMid:11742071 PMCid:PMC64998 -
Saeys, Y., I. Inza and P. Larran, 2007. A review of feature selection techniques in bioinformatics. Bioinformatics, 23(19): 2507-2517.
CrossRef PMid:17720704 -
Saraswathi, S., S. Sundaram, N. Sundararajan, M. Zimmermann and M. Nilsen-Hamilton, 2011. ICGA-PSO-ELM approach for accurate multiclass cancer classification resulting in reduced gene sets in which genes encoding secreted proteins are highly represented. IEEE-ACM T. Comput. Bi., 8(2): 452-463.
CrossRef -
Shanmugavadivu, T. and T. Ravichandran, 2013. Gene selection for cancer classification using microarrays. Int. J. Comput. Appl. Technol. Res., 2(5): 609-613.
CrossRef -
Stolovitzky, G., 2003. Gene selection in microarray data: The elephant, the blind men and our algorithms. Curr. Opin. Struc. Biol., 13(3): 370-376.
CrossRef -
Suresh, S., N. Sundarajan and P. Saratchandran, 2008. A sequential multi-category classifier using radial basis function networks. Neuro Comput., 71(7-9): 1345-1358.
CrossRef -
Suresh, S., S. Saraswathi and N. Sundararajan, 2010. Performance enhancement of extreme learning machine for multi-category sparse cancer classification. Eng. Appl. Artif. Intel., 23: 1149-1157.
CrossRef -
Zhang, R., G.B. Huang, N. Sundararajan and P. Saratchandran, 2007. Multicategory classification using an extreme learning machine for microarray gene expression cancer diagnosis. IEEE-ACM T. Comput. Bi., 4(3): 485-495.
Competing interests
The authors have no competing interests.
Open Access Policy
This article is distributed under the terms of the Creative Commons Attribution 4.0 International License (http://creativecommons.org/licenses/by/4.0/), which permits unrestricted use, distribution, and reproduction in any medium, provided you give appropriate credit to the original author(s) and the source, provide a link to the Creative Commons license, and indicate if changes were made.
Copyright
The authors have no competing interests.
|
|
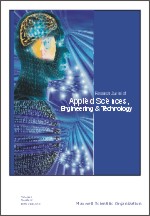 |
ISSN (Online): 2040-7467
ISSN (Print): 2040-7459 |
 |
Information |
|
|
|
Sales & Services |
|
|
|