Research Article | OPEN ACCESS
Robust Classification of Primary Brain Tumor in MRI Images using Wavelet as the Input of ANFIS
1B. Rajesh Kumar and 2S. Karpagaiswarya
1Department of Computer Science and Engineering, RVS College of Engineering and Technology, Coimbatore, India
2Anna University (Regional Centre), Coimbatore, India
Research Journal of Applied Sciences, Engineering and Technology 2014 7:811-816
Received: January 13, 2014 | Accepted: May 08, 2014 | Published: August 20, 2014
Abstract
This study presents a neural network based technique for automatic classification of Magnetic Resonance Images (MRI) of the brain in two categories of benign and malignant. The proposed method consists the following stages; i.e., preprocessing, tumor region segmentation, feature extraction using DWT and classification using ANFIS classifier. Preprocessing involves removing low-frequency surrounding noise, normalizing the intensity of the individual particle images. In the second stage, the fuzzy Connectedness segmentation is used for partitioning the image into meaningful regions. In feature extraction, the obtained feature connected to MRI images using the Discrete Wavelet Transform (DWT). In the classification stage, ANFIS Classifier is used to classify the subjects to normal or abnormal (benign, malignant). The proposed technique gives high-quality results for brain tissue detection and is more robust and efficient compared with other recent works.
Keywords:
ANFIS , classification , Discrete Wavelet Transform (DWT), fuzzy connectedness segmentation,
References
-
Ashby, L.S., M.M. Troester and W.R. Shapiro, 2006. Central nervous system tumors. Cancer Ther., 1: 475-513.
CrossRef -
Chaplot, S., L.M. Patnaik and N.R. Jagannathan, 2006. Classification of magnetic resonance brain images using wavelets as input to support vector machine and neural network. Biomed. Signal Proces., 1: 86-92.
CrossRef -
Doolittle, N.D., 2004. State of the science in brain tumor classification. Semin. Oncol. Nurs., 20: 224-230.
CrossRef -
Gerig, G., O. Kubler, R. Kikinis and F.A. Jolesz, 1992. Nonlinear anisotropic filtering of MRI data. IEEE T. Med. Imaging, 1(2): 221-232.
CrossRef PMid:18218376 -
Hiremath, P.S., S. Shivashankar and J. Pujari, 2006. Wavelet based features for color texture classification with application to CBIR. Int. J. Comput. Sci. Network Sec., 6(9A): 124-133.
-
Jaya, J., K. Thanushkodi and M. Karnan, 2009. Tracking algorithm for denoising of MR brain images. IJCSNS Int. J. Comput. Sci. Netw. Secur. 9(11): 262-267.
-
Jayachandran, A. and R. Dhanasekaran, 2013a. Automatic detection of brain tumor in magnetic resonance images using multi-texton histogram and support vector machine. Int. J. Imag. Syst. Tech., 23: 97-103.
CrossRef -
Jayachandran, A. and R. Dhanasekaran, 2013b. Brain tumor detection using fuzzy support vector machine classification based on a texton co-occurrence matrix. J. Imaging Sci. Techn., 57(1): 10507-1-10507-7(7).
-
Lashkari, A., 2010. A neural network based method for brain abnormality detection in MR images using gabor wavelets. Int. J. Comput. Appl., 4(7): 9-13.
CrossRef -
Lukas, L., A. Devos, J.A. Suykens, L. Vanhamme, F.A. Howe, C. Majo’s, A. Moreno-Torres, M. Van Der Graaf, A.R. Tate, C. Aru’s and S. Van Huffel, 2004. Brain tumor classification based on long echo proton MRS signals. Artif. Intell. Med., 31(1): 73-89.
CrossRef PMid:15182848 -
Mallat, S.G., 1980. A theory of multiresolution signal decomposition: The wavelet representation. IEEE T. Pattern Anal., 11(7): 674-693.
CrossRef -
Schmidt, M., I. Levner, R. Greiner, A. Murtha and A. Bistritz, 2005. Segmenting brain tumors using alignment-based features. Proceeding of 4th International Conference on Machine Learning and Applications, Los Angeles.
CrossRef -
Sengur, A., 2008. An expert system based on principal component analysis, artificial immune system and fuzzy k-NN for diagnosis of valvular heart diseases. Comput. Biol. Med., 51(3): 329-338.
CrossRef PMid:18177849 -
Silipo, R., G. Deco and H. Bartsch, 1999. Brain tumor classification based on EEG hidden dynamics. Intell. Data Anal., 3(4): 287-306.
CrossRef -
Subasi, A., 2007. Application of adaptive neuro-fuzzy inference system for epileptic seizure detection using wavelet feature extraction. Comput. Biol. Med., 37: 227-244.
CrossRef PMid:16480706 -
Zhu, W., N. Zeng and N. Wang, 2010. Sensitivity, specificity, accuracy, associated confidence interval and ROC analysis with practical SAS® implementations. Proceeding of NESUG: Health Care and Life Sciences. Baltimore, Maryland, pp: 1-9.
Competing interests
The authors have no competing interests.
Open Access Policy
This article is distributed under the terms of the Creative Commons Attribution 4.0 International License (http://creativecommons.org/licenses/by/4.0/), which permits unrestricted use, distribution, and reproduction in any medium, provided you give appropriate credit to the original author(s) and the source, provide a link to the Creative Commons license, and indicate if changes were made.
Copyright
The authors have no competing interests.
|
|
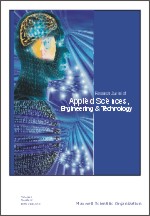 |
ISSN (Online): 2040-7467
ISSN (Print): 2040-7459 |
 |
Information |
|
|
|
Sales & Services |
|
|
|