Research Article | OPEN ACCESS
Simulating the Diesel Engine Vibration with Fuzzy Neural Network
1Sina Abroumand Azar and 2Arash Hosseinian Ahangarnejad
1Department of Mechanical Engineering, Islamic Azad University, Tabriz, Iran
2Department of Mechanical Engineering, Politecnico di Milano, Italy
Research Journal of Applied Sciences, Engineering and Technology 2014 9:1045-1051
Received: April 22, 2013 | Accepted: May 18, 2013 | Published: September 05, 2014
Abstract
This study is conducted in order to evaluate the models of artificial intelligence in predicting the level of diesel vibrations. In this study, the Artificial Neural Network (ANN) and the Adaptive Neuro Fuzzy Inference System (ANFIS) are used in order to simulate the vibration of the whole diesel engine. Vibration in the gasoline or diesel engines has been investigated according to numerous aspects so far. Noise and vibration, which occurs in the engine due to the combustion process, can make direct effects on the users. This is particularly true in the engines with large compression ratios and engines in which the combustion pressure increases rapidly. Results indicate that the vibration of Diesel engines can be predicted with reasonable accuracy by applying the smart models. The results of predicting the Artificial Neural Network are partially better than the Adaptive neuro fuzzy inference system.
Keywords:
Diesel engine , fuzzy neural network , simulation , vibration,
References
-
Andrew, L.G., C.H. Dianne and J.S. Brian, 2007. The effect of piston friction on the torsional natural frequency of a reciprocating engine. Mech. Syst. Signal Pr., 21(7): 2833-2837.
CrossRef
-
Bolan, L., Z. Changlu, Z. Fujun, C. Tao and S. Jianyun, 2013. Misfire detection of a turbocharged diesel engine by using artificial neural networks. Appl. Therm. Eng., 55(1-2): 26-32.
CrossRef
-
Caudill, M., 1987. Neural networks primer, Part I. AI Expert, pp: 46-52.
-
Dayhoff, J.E., 1990. Neural Network Principles. Prentice Hall International, USA.
PMid:11538873
-
Ettefagh, M.M., M.H. Sadeghi, V. Pirouzpanah and H. Arjmandi Tash, 2008. Knock detection in spark ignition engines by vibration analysis of cylinder block: A parametric modeling approach. Mech. Syst. Signal Pr., 22(6): 1495-1514.
CrossRef
-
Geng, Z. and J. Cheng, 2005. Investigation into piston-slap-induced vibration for engine condition simulation and monitoring. J. Sound Vib., 282(3-5): 735-751.
CrossRef
-
Ghobadian, B., H. Rahimi, A.M. Nikbakht, G. Najafi and T.F. Yusaf, 2008. Diesel engine performance and exhaust emission analysis using waste cooking biodiesel fuel with an artificial neural network. Renew. Energ., 34: 976-982.
CrossRef
-
Haddad, S.D. and H.L. Pillen, 1973. Piston slap as a source of noise and vibration in diesel engines. J. Sound Vib., 34(2): 249-260.
CrossRef
-
Harun, M.I., N. Hoon Kiat, W.Q. Cheen and G. Suyin, 2012. Artificial neural networks modeling of engine-out responses for a light-duty diesel engine fuelled with biodiesel blends. Appl. Energ., 92: 769-777.
CrossRef
-
Kesgin, U., 2003. Genetic algorithm and artificial neural network for engine optimisation of efficiency and NOx emission. Fuel, 83: 885-895.
CrossRef
-
Khanna, T., 1990. Foundations of Neural Networks. Addison-Wesley, New York.
-
Massie, D.D., 2001. Neural-network fundamentals for scientists and engineers. ECOS 01, Istanbul, Turkey, pp: 123.
-
Nourani, V. and K. Salehi, 2008. Fourth National Congress on Civil Engineering. University of Tehran.
PMCid:PMC2268442
-
Oguz, H., I. Saritas and H. Emre Baydan, 2010. Prediction of diesel engine performance using biofuels with artificial neural network. Expert Syst. Appl., 37(9): 6579-6586.
CrossRef
-
Parlak, A., Y. Islamoglu, H. Yasar and A. Egrisogut, 2005. Application of artificial neural network to predict specific fuel consumption and exhaust temperature for a diesel engine. Appl. Therm. Eng., 26: 824-828.
CrossRef
-
Talal, F.Y., D.R. Buttsworth, H.S. Khalid and B.F. Yousif, 2010. CNG-diesel engine performance and exhaust emission analysis with the aid of artificial neural network. Appl. Energ., 87(5): 1661-1669.
CrossRef
-
Tokar, A.S. and M. Markus, 2000. Precipitation-runoff modeling using artificial neural networks and conceptual models. J. Hydrol. Eng., 5: 156-161.
CrossRef
-
Yuanwang, D., Z. Meilin, X. Dong and C. Xiaobei, 2002. An analysis for effect of cetane number on exhaust different from the existing tests cycles. Fuel, 81(15): 1963-1970.
CrossRef
-
Yusaf, T.F., D.R. Buttsworth, K.H. Saleh and B.F. Yousif, 2010. CNG-diesel engine performance and exhaust emission analysis with the aid of artificial neural network. Appl. Energ., 87(5): 1661-1669.
CrossRef
Competing interests
The authors have no competing interests.
Open Access Policy
This article is distributed under the terms of the Creative Commons Attribution 4.0 International License (http://creativecommons.org/licenses/by/4.0/), which permits unrestricted use, distribution, and reproduction in any medium, provided you give appropriate credit to the original author(s) and the source, provide a link to the Creative Commons license, and indicate if changes were made.
Copyright
The authors have no competing interests.
|
|
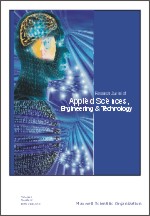 |
ISSN (Online): 2040-7467
ISSN (Print): 2040-7459 |
 |
Information |
|
|
|
Sales & Services |
|
|
|