Research Article | OPEN ACCESS
Segmentation of Lungs via Hybridization of CA and Level Set Algorithm
1A. Anbu Megelin Star and 2P. Subburaj
1Depertment of ECE, The Tamizhan College of Engineering and Technology,
Chenbagaramanputhoor, Tamil Nadu, India
2National Engineering College, Kovilpatti, India
Research Journal of Applied Sciences, Engineering and Technology 2014 9:1117-1124
Received: May 19, 2014 | Accepted: July 07, 2014 | Published: September 05, 2014
Abstract
In this study, we present a fast and robust practical tool for segmentation of solid tumors with minimal user interaction. The lung image is segmented manually by the experts may includes risk and time consuming process. Hence, in this study, the lung image is segmented in order to identify the tumor sector. Initially, the input lung image is applied with the denoising process for removing noises with the aid of multi-wavelet transformation. After this process, the CA algorithm is applied for obtaining the background seeds and foreground seeds (tumor seeds) and then the level set algorithm is applied for acquiring the acute tumor tissues. As a result of the mentioned process, the tumor sector is segmented and the results are depicted. Studies on lung tumor datasets demonstrate 80-85% overlap performance of the proposed algorithm with less sensitivity to seed initialization, robustness with respect to heterogeneous tumor types and its efficiency in terms of computation time.
Keywords:
Background seeds , CA (Cellular Automata) algorithm , foreground seeds , level set algorithm , lungs, segmentation , tumor,
References
-
Agarwal, P., H.K. Sardana and R. Vig, 2010. An efficient visualization and segmentation of lung CT scan images for early diagnosis of cancer. Proceeding of National Conference on Computational Instrumentation CSIO Chandigarh, pp: 100-104.
-
Chen, T.F., 2008. Medical image segmentation using level sets. Technical Report, pp: 1-8.
-
Dhole, S.S., R.V. Mante and P.N. Chatur, 2013. Automation of OPG image segmentation. Int. J. Adv. Res. Comput. Commun. Eng., 2(6): 4379-4381.
-
Hasan, M.A., S.L. Lee, D.H. Kim and M.K. Lim, 2012. Automatic evaluation of cardiac hypertrophy using cardiothoracic area ratio in chest radiograph images. Comput. Method. Prog. Biomed., 105: 95-108.
CrossRef PMid:21831474
-
Hashemi, A., A.H. Pilevar and R. Rafeh, 2013. Mass detection in lung CT images using region growing segmentation and decision making based on fuzzy inference system and artificial neural network. I.J. Image Graph. Signal Process., 6: 16-24.
CrossRef
-
Krishnan, J. and R. Robinson, 2013. A survey on lung segmentation techniques. Int. J. Comput. Sci. Eng. Technol. (IJCSET), 4(5): 616-621.
-
Mesanovic, N., M. Grgic, H. Huseinagic, M. Males, E. Skejic and M. Smajlovic, 2011. Automatic CT image segmentation of the lungs with region growing algorithm. Proceeding of IWSSIP, pp: 395-400.
-
Mohideen, K., A. Perumal, Krishnan and M. Sathik, 2011. Image denoising and enhancement using multiwavelet with hard threshold in digital mammographic images. Int. Arab J. e-Technol., 2(1): 49-55.
-
Mostofi, H., 2009. Fast Level set segmentation of biomedical images using graphics processing units. Final Year Project, Keble Colle.
PMCid:PMC2783186
-
Sasidhar, B., D.R. Ramesh Babu, N. Bhaskarao and B. Jan, 2013. Automated segmentation of lung regions and detection of lung cancer in CT scan. Int. J. Eng. Adv. Technol. (IJEAT), 2(4): 757-758.
-
Sharma, D. and G. Jindal, 2011. Identifying lung cancer using image processing techniques. Proceeding of International Conference on Computational Techniques and Artificial Intelligence (ICCTAI'2011), pp: 115-120.
-
Siddique, M.A., B. Selva Ganesh and R. Ganesan, 2014. ANFIS classifier based lung tumor severity diagnosis. Int. J. Adv. Res. Comput. Sci. Technol., 2(1): 80-83.
-
Sun, S., G. McLennan, E.A. Hoffman and R. Beichel, 2010. Model-based segmentation of pathological lungs in volumetric CT data. Proceeding of 3rd International Workshop on Pulmonary Image Analysis, pp: 31-40.
-
Velayudham, A. and R. Kanthavel, 2012. A survey on medical image denoising techniques. Int. J. Adv. Res. Electron. Commun. Eng. (IJARECE), 2(3): 272-276.
-
Wieclawe, W. and E. Pietka, 2012. Fuzzy clustering in intelligent scissors. Comput. Med. Imag. Grap., 36: 396-409.
CrossRef PMid:22483373
Competing interests
The authors have no competing interests.
Open Access Policy
This article is distributed under the terms of the Creative Commons Attribution 4.0 International License (http://creativecommons.org/licenses/by/4.0/), which permits unrestricted use, distribution, and reproduction in any medium, provided you give appropriate credit to the original author(s) and the source, provide a link to the Creative Commons license, and indicate if changes were made.
Copyright
The authors have no competing interests.
|
|
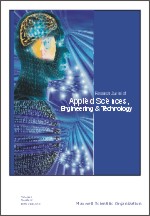 |
ISSN (Online): 2040-7467
ISSN (Print): 2040-7459 |
 |
Information |
|
|
|
Sales & Services |
|
|
|