Research Article | OPEN ACCESS
Genetic Algorithm Optimized Back Propagation Neural Network for Knee Osteoarthritis Classification
Jian WeiKoh, Tian-Swee Tan, Zhi EnChuah, Sarah Samson Soh, Muhammad Arif and KahMeng Leong
Department of Biotechnology and Medical Engineering, Faculty of Biosciences and Medical Engineering (FBME), Medical Implant Technologi Group (MediTEG), Material Manufacturing Research Alliance (MMRA), Universiti Teknologi Malaysia (UTM), 81310 Skudai Johor, Malaysia
Research Journal of Applied Sciences, Engineering and Technology 2014 16:1787-1793
Received: March 22, 2014 | Accepted: July 01, 2014 | Published: October 25, 2014
Abstract
Osteoarthritis (OA) is the most common form of arthritis that caused by degeneration of articular cartilage, which function as shock absorption cushion in our joint. The most common joints that infected by osteoarthritis are hand, hip, spine and knee. Knee osteoarthritis is the focus in this study. These days, Magnetic Resonance Imaging (MRI) technique is widely applied in diagnosis the progression of osteoarthritis due to the ability to display the contrast between bone and cartilage. Traditionally, interpretation of MR image is done manually by physicians who are very inconsistent and time consuming. Hence, automated classifier is needed for minimize the processing time of classification. In this study, genetic algorithm optimized neural network technique is used for the knee osteoarthritis classification. This classifier consists of 4 stages, which are feature extraction by Discrete Wavelet Transform (DWT), training stage of neural network, testing stage of neural network and optimization stage by Genetic Algorithm (GA). This technique obtained 98.5% of classification accuracy when training and 94.67% on testing stage. Besides, classification time is reduced by 17.24% after optimization of the neural network.
Keywords:
Classification, discrete wavelet transform, genetic algorithm, knee osteoarthritis, neural network,
References
-
Andrew, J.B., 2003. Analysing a Neural Network trained by Backpropagation. Report-Introduction to Neural Computation, the University of Birmingham.
-
Arthritis Research UK, 2013. Osteoarthritis in General Practice. Copeman House, Chesterfield.
-
Axelsson, A. and E.B. Björhall, 2003. Real time speech driven face animation. M.A. Thesis, at the Image Coding Group, Department of Electrical Engineering at Linköping University, Linköping.
-
Bandyopadhyay, S. and S.K. Pal, 2007. Classification and Learning using Genetic Algorithms: Application in Bioinformatics and Web Intelligence. Springer-Verlag, Berlin, Heidelberg, pp: 311.
-
Döhler, F., F. Mormann, B. Weber, C.E. Elger and K. Lehnertz, 2008. A cellular neural network based method for classification of magnetic resonance images: Towards an automated detection of hippocampal sclerosis. J. Neurosci. Meth., 170(2): 324-331.
CrossRef PMid:18294696 -
En, C. and T.T. Swee, 2013. Computer-aided knee osteoarthritis classification system using Artificial Neural Network (ANN). J. Med. Imag. Health Inform., 3(4): 561-565.
CrossRef -
Gold, G.E., C.A. Chen, S. Koo, B.A. Hargreaves and N.K. Bangerter, 2009. Recent advances in MRI of articular cartilage. Am. J. Roentgenol., 193(3): 628-638.
CrossRef PMid:19696274 PMCid:PMC2879429 -
Jiang, J., P. Trundle and J. Ren, 2010. Medical image analysis with artificial neural networks. Comput. Med. Imag. Grap., 34(8): 617-631.
CrossRef PMid:20713305 -
Kavzoglu, T., 1999. Determining optimum structure for artificial neural networks. Proceeding of the 24th Annual Technical Conference and Exhibition of the Remote Sensing Society, pp: 675-682.
-
Kotsiantis, S.B., I.D. Zaharakis and P.E. Pintelas, 2007. Supervised machine learning: A review of classification techniques. Proceeding of the 2007 Conference on Emerging Artificial Intelligence Applications in Computer Engineering: Real Word AI Systems with Applications in eHealth, HCI, Information Retrieval and Pervasive Technologies, pp: 3-24.
-
Man, K.F., K.S. Tang and S. Kwong, 1996. Genetic algorithms: Concepts and applications. IEEE T. Ind. Electron., 43(5): 519-534.
CrossRef -
Peterfy, C.G., E. Schneider and M. Nevitt, 2008. The osteoarthritis initiative: Report on the design rationale for the magnetic resonance imaging protocol for the knee. Osteoarthr. Res. Soc., 16(12): 1433.
CrossRef PMid:18786841 PMCid:PMC3048821 -
Sapkal, S.D., S.N. Kakarwal and P.S. Revankar, 2007. Analysis of classification by supervised and unsupervised learning. Proceeding of IEEE International Conference on Computational Intelligence and Multimedia Applications, 1: 280-284.
CrossRef -
Wilson, D.R. and T.R. Martinez, 2001. The need for small learning rates on large problems. Proceeding of International Joint Conference on Neural Networks (IJCNN'01), 1: 115-119.
CrossRef -
Zhang, Y., S. Wang and L. Wu, 2010. A novel method for magnetic resonance brain image classification based on adaptive chaotic PSO. Prog. Electromagn. Res., 109: 325-343.
CrossRef
Competing interests
The authors have no competing interests.
Open Access Policy
This article is distributed under the terms of the Creative Commons Attribution 4.0 International License (http://creativecommons.org/licenses/by/4.0/), which permits unrestricted use, distribution, and reproduction in any medium, provided you give appropriate credit to the original author(s) and the source, provide a link to the Creative Commons license, and indicate if changes were made.
Copyright
The authors have no competing interests.
|
|
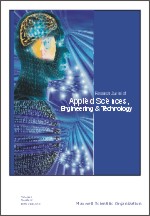 |
ISSN (Online): 2040-7467
ISSN (Print): 2040-7459 |
 |
Information |
|
|
|
Sales & Services |
|
|
|