Research Article | OPEN ACCESS
Face Recognition System Based on Sparse Codeword Analysis
1P. Geetha, 1E. Gomala and 2Vasumathi Narayanan
1Anna University, Chennai
2St.Joseph’s College of Engineering, Old Mamallapuram Road, Kamaraj Nagar, Semmencherry, Chennai, Tamil Nadu 600119, India
Research Journal of Applied Sciences, Engineering and Technology 2014 22:2265-2271
Received: September ‎13, ‎2014 | Accepted: September ‎20, ‎2014 | Published: December 15, 2014
Abstract
In recent times, large-scale content-based face image retrieval has grown up with rapid improvement and it is an enabling technology for many emerging applications. Content based face image retrieval is done by computing the similarity between images in the databases and the input/query face image. Content based face image retrieval systems retrieves the image only using low level features therefore the retrieval rate is low in this system. To improve the retrieval rate sparse codeword based scalable face image retrieval system is developed. This system uses both low level features and high level human attributes. The proposed system has several stages to retrieve the images; 1. Low level features are extracted using LTP descriptor and utilize the automatically detected high level human attributes such as hair, Gender and race. 2. Sparse codeword techniques are applied on the low level features and attributes to generate the codeword. 3. The third stage is an indexing; in the indexing attribute embedded inverted indexing method is used. Using the methods mentioned above, face image retrieval system has achieved promising retrieval result. Experiment is conducted on different dataset such as pub fig, LFW and FERET. Among those dataset LFW dataset achieve higher performance.
Keywords:
Content based image retrieval, face image search, high level features, sparse coding,
References
-
Ahonen, V., A. Hadid and M. Pietikainen, 2004. Face recognition with local binary patterns. Proceeding of the European Conference on Computer Vision, pp: 469-481.
CrossRef
-
Huang, G.B., M. Ramesh, T. Berg and E. Learned-Miller, 2007. Labeled faces in the wild: A database for studying face recognition in unconstrained environments. Technical Report, University of Massachusetts, Amherst, MA, USA, pp: 07-49.
-
Krishnan, B., M. Veerasamy and G. Nagammapudur, 2011. Effect of weight assignment in data fusion based information retrieval. Int. Arab J. Inf. Techn., 8(3): 244-250.
-
Kumar, N., A.C. Berg, P.N. Belhumeur and S.K. Nayar, 2011. Describable visual attributes for face verification and image search. IEEE T. Pattern Anal., 33(10): 1962-1977.
CrossRef PMid:21383395
-
Lazebnik, S., C. Schmid and J. Ponce, 2006. Beyond bags of features: Spatial pyramid matching for recognizing natural scene categories. Proceeding of the IEEE Conference on Computer Vision and Pattern Recognition, pp: 2169-2178.
CrossRef
-
Milborrow, S. and F. Nicolls, 2008. Locating facial features with an extended active shape model. Proceeding of the European Conference on Computer Vision, pp: 504-513.
CrossRef
-
Nayar, S.K., A.C. Berg, P.N. Belhumeur and N. Kumar, 2009. Attribute and simile classifiers for face verification. Proceeding of the International Conference on Computer Vision, pp: 202-207.
-
Parikh, D. and K. Grauman, 2011. Relative attributes. Proceeding of the IEEE International Conference on Computer Vision, pp: 503-510.
CrossRef
-
Phung, S.L., Bouzurdom, A. and D. Chai, 2002. A novel skin color model in YCbCr color space and its application to human face detection. Proceeding of the IEEE International Conference on Image Processing, 1: 289-292.
CrossRef
-
Raina, R., A. Battle, H. Lee, B. Packer and A.Y. Ng, 2007. Self-taught learning: Transfer learning from unlabeled data. Proceeding of the 24th International Conference on Machine Learning (ICML, 2007), pp: 759-766.
-
Scheirer, W., N. Kumar, K. Ricanek, T.E. Boult and P.N. Belhumeur, 2011. Fusing with context: A Bayesian approach to combining descriptive attributes. Proceeding of the International Joint Conference on Biometrics Compendium, pp: 1-8.
CrossRef
-
Torralba, A., K.P. Murphy, W.T. Freeman and M.A. Rubin, 2003. Context based vision system for place and object recognition. Proceeding of the IEEE International Conference on Computer Vision, 1: 273-280.
CrossRef
-
Viola, P. and M. Jones, 2001. Rapid object detection using a boosted cascade of simple features. Proceeding of the IEEE Conference on Computer Vision and Pattern Recognition, 1: 511-518.
CrossRef
-
Yang, J., K. Yu, Y. Gong and T. Huang, 2009, Linear spatial pyramid matching using sparse coding for image classification. Proceeding of the IEEE Conference on Computer Vision and Pattern Recognition, pp: 1794-1801.
PMid:20193549
Competing interests
The authors have no competing interests.
Open Access Policy
This article is distributed under the terms of the Creative Commons Attribution 4.0 International License (http://creativecommons.org/licenses/by/4.0/), which permits unrestricted use, distribution, and reproduction in any medium, provided you give appropriate credit to the original author(s) and the source, provide a link to the Creative Commons license, and indicate if changes were made.
Copyright
The authors have no competing interests.
|
|
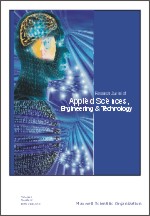 |
ISSN (Online): 2040-7467
ISSN (Print): 2040-7459 |
 |
Information |
|
|
|
Sales & Services |
|
|
|