Research Article | OPEN ACCESS
An Effective Pruning based Outlier Detection Method to Quantify the Outliers
1Kamal Malik, 2Harsh Sadawarti and 3G.S. Kalra
1MMICT and BM, MMU, Mullana, Haryana
2RIMTIET (Affiliated to Punjab Technical University)
3Lovely Professional University, Punjab, India
Research Journal of Applied Sciences, Engineering and Technology 2015 4:257-261
Received: July 18, 2014 | Accepted: October 17, 2014 | Published: February 05, 2015
Abstract
Outliers are the data objects that do not conform to the normal behaviour and usually deviates from the remaining data objects may be due to some outlying property which distinguishes them from the whole dataset. Usually, the detection of outliers is followed by the clustering of the dataset which sometimes ignores the prominency of outliers. In this study, we have tried to detect the outliers and pruned the clustering elements initially so that the outliers can be prominently highlighted. We have proposed an algorithm which effectively prunes the similar data objects from the large datasets and its experimental results compare the neighbouring points and show the better performance than the existing methods.
Keywords:
Clusters , distance-based, pruning,
References
-
Angiulli, F. and C. Pizzuti, 2005. Outlier mining in large high-dimensional data sets. IEEE T. Knowl. Data En., 17: 203-215.
CrossRef
-
Angiulli, F., S. Basta and C. Pizzuti, 2006. Distance-based detection and prediction of outliers. IEEE T. Knowl. Data En., 18(2): 145-160.
CrossRef
-
Barnett, V. and T. Lewis, 1994. Outliers in Statistical Data. John Wiley and Sons, New York.
-
Breunig, M.M., H.P. Kriegel, R.T. Ng and J. Sander, 2000. LOF: Identifying density-based local outliers. SIGMOD Rec., 29(2): 93-104.
CrossRef
-
Guha, S., R. Rastogi and K. Shim, 1998. CURE: An efficient clustering algorithm for large databases. SIGMOD Rec., 27(2): 73-84.
CrossRef
-
Knorr, E.M. and R.T. Ng, 1998. Algorithms for mining distance-based outliers in large datasets. Proceeding of 24th International Conference on Very Large Data Bases (VLDB, 1998), pp: 392-403.
-
Ng, R.T. and J. Han, 1994. Efficient and effective clustering methods for spatial data mining. Proceeding of the 20th International Conference on Very Large Data Bases (VLDB, 1994). Santiago, Chile, pp: 144-155.
-
Pamula, R., J.K. Deka and S. Nandi, 2011. An outlier detection method based on clustering. Proceeding of 2nd International Conference on Emerging Applications of Information Technology, pp: 253-256.
CrossRef
-
Ramaswamy, S., R. Rastogi and K. Shim, 2000. Efficient algorithms for mining outliers from large data sets. Proceeding of the 2000 ACM SIGMOD International Conference on Management of Data (SIGMOD '00), pp: 427-438.
CrossRef PMid:10870986
-
Tucakov, V., E.M. Knorr and R.T. Ng, 2000. Distance-based outliers: algorithms and applications. VLDB J., 8(3-4): 237-253.
CrossRef
-
Zhang, K., M. Hutter and H. Jin, 2009. A new local distance-based outlier detection approach for scattered real-world data. Proceeding of the 13th Pacific-Asia Conference on Advances in Knowledge Discovery and Data Mining (PAKDD ’09), pp: 813-822.
CrossRef
-
Zhang, T., R. Ramakrishnan and M. Livny, 1996. Birch: An efficient data clustering method for very large databases. SIGMOD Rec., 25(2): 103-114.
CrossRef
Competing interests
The authors have no competing interests.
Open Access Policy
This article is distributed under the terms of the Creative Commons Attribution 4.0 International License (http://creativecommons.org/licenses/by/4.0/), which permits unrestricted use, distribution, and reproduction in any medium, provided you give appropriate credit to the original author(s) and the source, provide a link to the Creative Commons license, and indicate if changes were made.
Copyright
The authors have no competing interests.
|
|
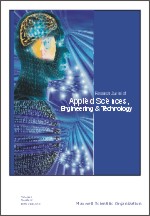 |
ISSN (Online): 2040-7467
ISSN (Print): 2040-7459 |
 |
Information |
|
|
|
Sales & Services |
|
|
|