Research Article | OPEN ACCESS
Predicting Performance of Schools by Applying Data Mining Techniques on Public Examination Results
1J. Macklin Abraham Navamani and 2A. Kannammal
1Department of Computer Applications, Karunya University
2Department of Computer Applications, Coimbatore Institute of Technology, Coimbatore, India
Research Journal of Applied Sciences, Engineering and Technology 2015 4:262-271
Received: July 24, 2014 | Accepted: October 12, 2014 | Published: February 05, 2015
Abstract
This study work presents a systematic analysis of various features of the higher grade school public examination results data in the state of Tamil Nadu, India through different data mining classification algorithms to predict the performance of Schools. Nowadays the parents always targets to select the right city, school and factors which contributes to the success of the results in schools of their children. There could be possible effects of factors such as Ethnic mix, Medium of study, geography could make a difference in results. The proposed work would focus on two fold factors namely Machine Learning algorithms to predict School performance with satisfying accuracy and to evaluate the data mining technique which would give better accuracy of the learning algorithms. It was found that there exist some apparent and some less noticeable attributes that demonstrate a strong correlation with student performance. Data were collected through the credible source data preparation and correlation analysis. The findings revealed that the public examinations results data was a very helpful predictor of performance of school in order to improve the result with maximum level and also improved the overall accuracy with the help of Adaboost technique.
Keywords:
Educational data mining , prediction of school performance , public examination , random forest,
References
-
Abu Afza, A.J.M., F. Dewan and M.R. Chowdhury, 2011. A hybrid classifier using boosting, clustering and naďve Bayesian classifier. World Comput. Sci. Inform. Technol. J., 1(3): 105-109.
-
Adeyemi, T.O., 2008. Predicting students’ performance in senior secondary certificate examinations from performance in junior secondary certificate examinations in Ondo State, Nigeria. Hum. Soc. Sci. J., 3(1): 26-36.
CrossRef -
Anon, 2013. Data Mining Algorithms in R/Classification/kNN. [Online].
Direct Link -
Anon, 2014a. Wikipedia.
Direct Link -
Anon, 2014b. Random Forest.
Direct Link -
Barnes, T., M. Desmarais, C. Romero and S. Ventura, 2009. Educational data mining. Proceeding of 2nd International Conference on Educational Data Mining. Cordoba, Spain.
-
Breiman, L., 2001. Random Forest. Statistics Department University of California, Berkeley, CA 94720.
-
Brijesh, K.B. and P. Saurabh, 2011. Mining educational data to analyze students’ performance. Int. J. Adv. Comput. Sci. Appl., 2(6): 63-69.
-
Cherif, H., L. Vincent and Hocine, 2011. Accuracy Measures for the Comparison of Classifiers.
Direct Link -
Dietterich, T.G., 2014. Ensemble Methods in Machine Learning. Important Milestones of Directorate of Government Examinations.
Direct Link -
Freund, Y. and R.E. Schapire, 2007. Experiments with a new boosting algorithm. Proceeding of the 13th International Conference on Machine Learning, pp: 148-156.
-
Garc´ia-Saiz, D. and M. Zorrilla, 2011. Comparing classification methods for predicting distance students’ performance. Proceeding of the 2nd Workshop on Applications of Pattern Analysis Workshop and Conference, pp: 26-32.
-
Kabakchieva, D., 2013. Predicting student performance by using data mining methods of classification. Cybern. Inform. Technol., 13(1): 61-72.
CrossRef -
Macklin, A.N.J., A. Kannammal and J.T.P. Ranjit, 2014. Building multi-dimensional cube to analyze public examination results: A business intelligence approach. Appl. Mech. Mater., 622: 11-22.
CrossRef -
Markus, H. and K. Ralf, 2013. RapidMiner: Data Mining Use Cases and Business Analytics Applications. Chapman and Hall/CRC.
Direct Link -
Micheline, K. and H. Jiawei, 2008. Data Mining-concepts and Techniques. 2nd Edn., Morgan Kaufmann-Elsevier Publishers.
Direct Link -
Naidoo, P.J., D.G. Anton and P. Jordan, 2014. School Evaluation for Quality Improvement. International Institute for Educational Planning.
Direct Link -
Romero, C. and S. Ventura, 2010. Educational data mining: A review of the state-of-the-art. IEEE T. Syst. Man Cy. C, 40: 601-618.
CrossRef -
Sonali, A., G.N. Pandey and M.D. Tiwari, 2012. Data mining in education: Data classification and decision tree approach. Int. J. e-Educ. e-Bus. e-Learn., 2(2): 140-144.
-
Steven, B., 2009. Natural Language Processing with Python. O'Reilly Media.
-
Sundar, P.V., 2013. A comparative study for predicting student’s academic performance using Bayesian network classifiers. IOSR J. Eng., 3(2): 37-42.
CrossRef -
Zaki, M.J. and W. Meira Jr., 2013. Data Mining and Analysis: Fundamental Concepts and Algorithms.
Competing interests
The authors have no competing interests.
Open Access Policy
This article is distributed under the terms of the Creative Commons Attribution 4.0 International License (http://creativecommons.org/licenses/by/4.0/), which permits unrestricted use, distribution, and reproduction in any medium, provided you give appropriate credit to the original author(s) and the source, provide a link to the Creative Commons license, and indicate if changes were made.
Copyright
The authors have no competing interests.
|
|
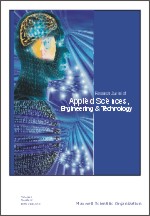 |
ISSN (Online): 2040-7467
ISSN (Print): 2040-7459 |
 |
Information |
|
|
|
Sales & Services |
|
|
|