Research Article | OPEN ACCESS
Artificial Neural Network Techniques in Identifying Plain Woven Fabric Defects
1P. Banumathi and 2G.M. Nasira
1Department of Computer Science and Engineering, Kathir College of Engineering,
Neelambur, Coimbatore
2Department of Computer Science and Applications, Chikkanna Government Arts College, Tirupur, India
Research Journal of Applied Sciences, Engineering and Technology 2015 4:272-276
Received: July 27, 2014 | Accepted: September 20, 2014 | Published: February 05, 2015
Abstract
Textile industry is one of the main sources of revenue generating industry. The price of fabrics is severely affected by the defects of fabrics that represent a major threat to the textile industry. In manual inspection a very small percentage of defects are detected with highly trained, experienced inspectors. An automatic defect detection system can increase the defect detection percentage. It reduces the fabrication cost and economically profitable when we consider the labor cost and associated benefits. In this study we have proposed a method to detect the defects in woven fabric based on the changes in the intensity of fabric. The images are acquired; preprocessed, statistical features based on the gray level co-occurrence matrix are extracted. The Artificial Neural Network is used as classification model. The extracted features are given as input to the artificial neural network, it identifies the defect. The result of proposed method shows that a better performance achieved with less time when compared with the existing methods.
Keywords:
Artificial neural networks, defect detection, gray level co-occurrence matrix, image processing, statistical approach,
References
-
Chandra, J.K., P.K. Banerjee and A.K. Datta, 2010. Neural network trained morphological processing for the detection of defects in woven fabric. J. Text. I., 101(8): 699-706.
CrossRef
-
Haralick, R.M., K. Shanmugan and I. Dinstein, 1973. Textural features for image classification. IEEE T. Syst. Man Cyb., SMC-3: 610-621.
CrossRef
-
Javed, A., M.A. Ullah and A.U. Rehman, 2013. Comparative analysis of different fabric defects detection techniques. I. J. Image Graph. Signal Process., 1: 40-45.
CrossRef
-
Li, P.F., Y. Jiao, J.F. Jing and J. Li, 2013. The high-speed fabric defect detection algorithm based on the image layered model. J. Fiber Bioeng. Inform., 6(2): 161-173.
CrossRef
-
Nagrale, S.K. and S.T. Bagde, 2013. Application of image processing for development of automated inspection system. Int. J. Comput. Eng. Res., 3(3).
-
Srinivasan, G.N. and G. Shobha, 2008. Statistical texture analysis. Proc. Wrld. Acad. Sci. E., Vol. 36, ISSN: 2070-3740.
-
Zhang, Y.H. and W.K. Wong, 2011. An intelligent model for detecting and classifying color-textured fabric defects using genetic algorithms and the Elman neural network. Text. Res. J., 81(17): 1772-1787.
CrossRef
Competing interests
The authors have no competing interests.
Open Access Policy
This article is distributed under the terms of the Creative Commons Attribution 4.0 International License (http://creativecommons.org/licenses/by/4.0/), which permits unrestricted use, distribution, and reproduction in any medium, provided you give appropriate credit to the original author(s) and the source, provide a link to the Creative Commons license, and indicate if changes were made.
Copyright
The authors have no competing interests.
|
|
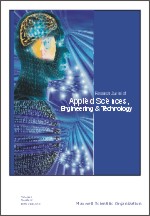 |
ISSN (Online): 2040-7467
ISSN (Print): 2040-7459 |
 |
Information |
|
|
|
Sales & Services |
|
|
|