Research Article | OPEN ACCESS
Detection of Attacks on MAODV Association Rule Mining Optimization
1A. Fidalcastro and 2E. Baburaj
1Department of CSE, Sathyabama University, Chennai, Tamilnadu, India
2Department of CSE, Sun College of Engineering and Technology, Nagercoil, India
Research Journal of Applied Sciences, Engineering and Technology 2015 6:454-459
Received: October 10, 2014 | Accepted: November 26, 2014 | Published: February 25, 2015
Abstract
Current mining algorithms can generate large number of rules and very slow to generate rules or generate few results, omitting interesting and valuable information. To address this problem, we propose an algorithm Optimized Featured Top Association Rules (OFTAR) algorithm, where every attack have many features and some of the features are more important. The Features are selected by genetic algorithm and processed by the OFTAR algorithm to find the optimized rules. The algorithm utilizes Genetic Algorithm feature selection approach to find optimized features. OFTAR incorporate association rules with several rule optimization techniques and expansion techniques to improve efficiency. Increasing popularity of Mobile ad hoc network users of wireless networks lead to threats and attacks on MANET, due to its features. The main challenge in designing a MANET is protecting from various attacks in the network. Intrusion Detection System is required to monitor the network and to detect the malicious node in the network in multi casting mobility environment. The node features are processed in Association Analysis to generate rules, the generated rules are applied to nodes to detect the attacks. Experimental results show that the algorithm has higher scalability and good performance that is an advantageous to several association rule mining algorithms when the rule generation is controlled and optimized to detect the attacks.
Keywords:
Association analysis, black hole attack, data mining, genetic algorithm, intrusion detection system, MANET , spoofing attack , top rules,
References
-
Agrawal, R., T. Imielminski and A. Swami, 1993. Mining association rules between sets of items in large databases. Proceeding of the ACM International Conference on Management of Data, ACM Press, pp: 207-216.
CrossRef -
Bahaya, W.S. and S.A. AlAsady, 2012. Prevention of spoofing attacks in the infrastructureless wireless networks. J. Comput. Sci., 8(10): 1769-1779.
CrossRef -
Chen, Y., W. Trappe and R.P. Martin, 2007. Detecting and localizing wireless spoofing attacks. Proceeding of the 40th Annual IEEE Communication Society Conference on Sensor, Mesh and Ad Hoc Communication and Networks (SECON, 2007), pp: 193-202.
CrossRef -
Cheung, D.W.L., J. Han, V. Ng and C.Y. Wong, 1996. Maintenance of discovered association rules in large databases: An incremental updating technique. In ICDE, pp: 106-114.
-
Fournier-Viger, P. and V.S. Tseng, 2012b. Mining top-K non-redundant association rules. In: Chen, L. et al. (Ed.), ISMIS, 2012. LNAI 7661, Springer-Verlag, Berlin, Heidelberg, pp: 31-40.
CrossRef -
Fournier-Viger, P., C.W. Wu and V.S. Tseng, 2012a. Mining top-K association rules. In: Kosseim, L. and D. Inkpen (Eds.), Canadian AI, 2012. LNAI 7310, Springer-Verlag, Berlin, Heidelberg, pp: 61-73.
CrossRef -
Hassan, H.M., M. Mahmoud and S. El-Kassas, 2006. Securing the AODV protocol using specification-based intrusion detection. Proceeding of the 2nd ACM International Workshop on Quality of Service and Security for Wireless and Mobile Networks (Q2SWinet'06), pp: 33-36.
CrossRef -
Hu, Y.C., A. Perrig and D.B. Johnson, 2003. Rushing attacks and defense in wireless ad-hoc network routing protocols. Proceeding of the 2nd ACM Workshop on Wireless Security. USA, pp: 30-40.
CrossRef -
Karchirski, O. and R. Guha, 2008. Effective intrusion detection using multiple sensors in wireless ad hoc networks. Proceeding of the 36th Hawaii International Conference on System Sciences (HICSS’03), pp: 57.
-
Karmore, P.K. and S.M. Nirkhi, 2011. Detecting intrusion on AODV based mobile ad hoc networks by k-means clustering method of data mining. Int. J. Comp. Sci. Inform. Technol., 2(4): 1774-1779.
-
Peyam, K. and A. Mehran, 2011. Feature analysis for intrusion detection in mobile ad-hoc networks. Int. J. Netw. Secur., 12(1): 42-49.
-
Sadia, P., 2014. Intrusion detection model based on data mining technique. IOSR J. Comput. Sci., 2014: 34-39.
-
Tan, P.N., M. Steinbach and V. Kumar, 2006. Introduction to Data Mining. 2nd Edn., Pearson Education, Boston, Munich.
-
Vikas, M. and M.D. Shirish, 2012. General study of association rule mining in intrusion detection system. Int. J. Emerg. Technol. Adv. Eng., 2(1): 347-356.
-
Yufang, Z. and K. Thomas, 2004. MAODV implementation for NS-2.26. System and Computing Engineering, Carleton University, Technical Report SCE-04-01.
Competing interests
The authors have no competing interests.
Open Access Policy
This article is distributed under the terms of the Creative Commons Attribution 4.0 International License (http://creativecommons.org/licenses/by/4.0/), which permits unrestricted use, distribution, and reproduction in any medium, provided you give appropriate credit to the original author(s) and the source, provide a link to the Creative Commons license, and indicate if changes were made.
Copyright
The authors have no competing interests.
|
|
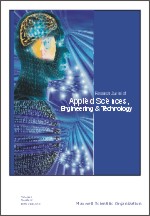 |
ISSN (Online): 2040-7467
ISSN (Print): 2040-7459 |
 |
Information |
|
|
|
Sales & Services |
|
|
|