Abstract
|
Article Information:
Prediction and Simulation the Breakthrough of Residual Chlorine Removal by Granular Activated Carbon Adsorbent Using Artificial Neural Networks
Rusul Naseer, Ala!a Abdulrazaq Jassim, Lü Xi-Wu and Saad Abualhail
Corresponding Author: Lü Xi-Wu
Submitted: January 12, 2012
Accepted: February 09, 2012
Published: July 01, 2012 |
Abstract:
|
This study has included two parts. The first part has dealt with carbon production whereas the date
Palm was used to produce Granular Activated Carbon (GAC) with specific physical characteristics. The new
produced of GAC is used to adsorbate the Residual chlorine from water by deep bed filter column. In the
second part, the experimental results of the breakthrough of residual chlorine curves is predicted and simulated
using artificial neural network with back propagation algorithm whereas the optimum number of neuron was
investigated based on RMSE. The removal of residual chlorine has been used as target function in ANN while
the other properties of adsorption process such as operation conditions, chlorine concentration in raw water and
GAC characteristics has been used as input parameters. The results showed that ANN with back propagation
algorithm is a good tool that can be used to predict the best operating parameter for designing GAC layer in
multimedia filter whereas 35 neuron gave the best fitting with experimental data. In addition to that, the
simulation result was showed that the predictions of breakthrough curve model has been coincided well with
the measured values which explained that the depth 25 cm with grain size 1.5 mm of GAC filter bed will be
give the optimum removal of residual chlorine from chlorinated water.
Key words: Activated carbon, Artificial Neural Network (ANN), breakthrough curve, chlorine adsorption, , ,
|
Abstract
|
PDF
|
HTML |
|
Cite this Reference:
Rusul Naseer, Ala!a Abdulrazaq Jassim, Lü Xi-Wu and Saad Abualhail, . Prediction and Simulation the Breakthrough of Residual Chlorine Removal by Granular Activated Carbon Adsorbent Using Artificial Neural Networks. Research Journal of Applied Sciences, Engineering and Technology, (13): 1883-1887.
|
|
|
|
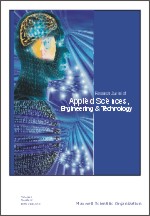 |
ISSN (Online): 2040-7467
ISSN (Print): 2040-7459 |
 |
Information |
|
|
|
Sales & Services |
|
|
|