Research Article | OPEN ACCESS
Research on Data of Food Information Forecasting based on Data Mining Combined Model
Liu Xiuju
Department of Computer and Information Engineering, Heze University, Heze Shandong, China
Advance Journal of Food Science and Technology 2016 7:371-375
Received: October ‎13, ‎2015 | Accepted: December ‎9, ‎2015 | Published: November 05, 2016
Abstract
This study has been explored food information data prediction implementation access based on data mining combination model. With data mining technology as the entry point and in combination with the analysis on food information data prediction characteristics. Research on variable substitution to non-linear regression forecast model precision's influence and seek the modeling method that can improve the forecast precision. Based on the Data mining, the transform in space and the weighted processing combined method, make full use of information that the primary data provide. Given modeling method of combination forecast model based on the Data mining. Based on Data mining’s combination forecast model’s modeling method can reduce the serious influence that the variable substitution brings and has fully used useful information in the primary data. It obviously improved the accuracy of the prediction model.
Keywords:
Data mining technology, data prediction, non-linear model, space trans-formation,
References
-
Alzghoul, A., M. Lofstrand and B. Backe, 2012. Data stream food information forecasting for system fault prediction. Comput. Ind. Eng., 62(4): 972-978.
CrossRef -
Hashemi, S. and Y. Yang, 2009. Flexible decision tree for data stream classification in the presence of concept change, noise and missing values. Data Min. Knowl. Disc., 19(1): 95-131.
-
Junier, S. and E. Mostert, 2014. A decision support system for the implementation of the water framework directive in the Netherlands: Process, validity and useful information. Environ. Sci. Policy, 40: 49-56.
CrossRef Direct Link -
Krause, F.L. and U. Kaufmann, 2007. Meta-modelling for interoperability in data mining. CIRP Ann., 145: 191-196.
-
Lines, J., L.M. Davis, J. Hills and A. Bagnall, 2012. A shapelet transform for time series classification. Proceeding of the 18th ACM SIGKDD International Conference on Knowledge Discovery and Data Mining (KDD '12 ), pp: 289-297.
-
Susnea, E., 2013. Improving decision making process in universities: A conceptual model of intelligent decision support system. Proc. Soc. Behav. Sci., 76: 795-800.
-
Trujillo, J. and S. Luján-Mora, 2003. A UML based approach for modeling ETL processes in data warehouses. In: Song, I.Y. et al. (Eds.), Conceptual Modeling - ER 2003. Lecture Notes in Computer Science, Springer-Verlag, Berlin, Heidelberg, 2813: 307-320.
Competing interests
The authors have no competing interests.
Open Access Policy
This article is distributed under the terms of the Creative Commons Attribution 4.0 International License (http://creativecommons.org/licenses/by/4.0/), which permits unrestricted use, distribution, and reproduction in any medium, provided you give appropriate credit to the original author(s) and the source, provide a link to the Creative Commons license, and indicate if changes were made.
Copyright
The authors have no competing interests.
|
|
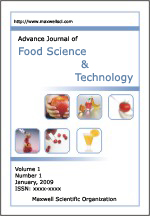 |
ISSN (Online): 2042-4876
ISSN (Print): 2042-4868 |
 |
Information |
|
|
|
Sales & Services |
|
|
|