Research Article | OPEN ACCESS
Prediction of the Yield of Enzymatic Synthesis of Betulinic Acid Ester Using Artificial Neural Networks and Support Vector Machine
1Run Wang, 2Qiaoli Mo, 1Qian Zhang, 3Fudi Chen and 3, 4Dazuo Yang
1College of Light Industry, Textile and Food Science Engineering
2College of Chemistry, Sichuan University, Chengdu, Sichuan 610064, China
3Key Laboratory of Marine Bio-Resources Restoration and Habitat Reparation in Liaoning Province, Dalian Ocean University, Dalian 116023, China
4College of Life Science and Technology, Dalian University of Technology, Dalian 116021, China
Advance Journal of Food Science and Technology 2016 12:653-662
Received: July ‎7, ‎2015 | Accepted: August ‎2, ‎2015 | Published: December 25, 2016
Abstract
3&beta-O-phthalic ester of betulinic acid is of great importance in anticancer studies. However, the optimization of its reaction conditions requires a large number of experimental works. To simplify the number of times of optimization in experimental works, here, we use Artificial Neural Network (ANN) and Support Vector Machine (SVM) models for the prediction of yields of 3&beta-O-phthalic ester of betulinic acid synthesized by betulinic acid and phthalic anhydride using lipase as biocatalyst. General Regression Neural Network (GRNN), Multilayer Feed-forward Neural network (MLFN) and the SVM models were trained based on experimental data. Four indicators were set as independent variables, including time (h), temperature (°C), amount of enzyme (mg) and molar ratio, while the yield of the 3β-O-phthalic ester of betulinic acid was set as the dependent variable. Results show that the GRNN and SVM models have the best prediction results during the testing process, with comparatively low RMS errors (4.01 and 4.23 respectively) and short training times (both 1s). The prediction accuracy of the GRNN and SVM are both 100% in testing process, under the tolerance of 30%.
Keywords:
Artificial neural network, betulinic acid ester, biocatalyst, support vector machine,
References
-
Chen, F., H. Li, Z. Xu, S. Hou and D. Yang, 2015. User-friendly optimization approach of fed-batch fermentation conditions for the production of iturin A using artificial neural networks and support vector machine. Electron. J. Biotechn., 18(4): 273-280.
Direct Link -
Dayhoff, J.E. and J.M. DeLeo, 2001. Artificial neural networks: Opening the black box. Cancer, 91(8 Suppl.): 1615-1635.
Direct Link -
Friesen, D., M. Patterson and B. Harmel, 2011. Comparison of multiple regression and neural networks for forecasting real estate values. Reg. Bus. Rev., 30: 114.
-
Gauthier, C. and J. Legault, 2008. Synthesis of two natural betulinic acid saponins containing a- l -rhamnopyranosyl-(1?2)-a- l -arabinopyranose and their analogues. Tetrahedron, 64: 7386-7399.
-
Goulermas, J.Y., P. Liatsis, X.J. Zeng and P. Cook, 2007. Density-driven generalized regression neural networks (dd-grnn) for function approximation. IEEE T. Neural Networ., 18(6): 1683-1696.
CrossRef PMid:18051185 -
Guo, Q. and Y. Liu, 2010. Modeco: An integrated software package for ecological niche modeling. Ecography, 33(4): 637-642.
CrossRef -
Hopfield, J.J., 1988. Artificial neural networks. IEEE Circuit. Devic., 4(5): 3-10.
CrossRef -
Hoskins, J.C. and D.M. Himmelblau, 1988. Artificial neural network models of knowledge representation in chemical engineering. Comput. Chem. Eng., 12(9-10): 881-890.
CrossRef -
Johansson, E.M., F.U. Dowla and D.M. Goodman, 1991. Backpropagation learning for multilayer feed-forward neural networks using the conjugate gradient method. Int. J. Neural Syst., 2(4): 291-301.
Direct Link -
Kandirmaz, H.M., K. Kaba and M. Avci, 2014. Estimation of monthly sunshine duration in Turkey using artificial neural networks. Int. J. Photoenergy, 2014: 9.
CrossRef -
Kim, D.W., K.Y. Lee, D. Lee and K.H. Lee, 2005. A kernel-based subtractive clustering method. Pattern Recogn. Lett., 26(7): 879-891.
CrossRef -
Kvasnica, M., J. Sarek, E. Klinotova, P. Dzubak and M. Hajduch, 2005. Synthesis of phthalates of betulinic acid and betulin with cytotoxic activity. Bioorgan. Med. Chem., 13(10): 3447-3454.
Direct Link -
Li, H., W. Leng, Y. Zhou, F. Chen, Z. Xiu and D. Yang, 2014a. Evaluation models for soil nutrient based on support vector machine and artificial neural networks. Sci. World. J., 2014: 7.
CrossRef -
Xu and X. Liu, 2014b. Prediction of Henry's law constants for organic compounds using multilayer feedforward neural networks based on linear solvation energy relationship. J. Chem. Pharm. Res., 6(6): 1557-1564.
-
Loughlin, W.A., 2000. Biotransformations in organic synthesis. Bioresource Technol., 74(1): 49-62.
Direct Link -
Moghaddam, M.G., F.B.H. Ahmad, M. Basri and M.B.A. Rahman, 2010. Artificial neural network modeling studies to predict the yield of enzymatic synthesis of betulinic acid ester. Electron. J. Biotechn., 13(3): 2182-2185.
Direct Link -
Mukherjee, R., M. Jaggi, P. Rajendran, M.J.A. Siddiqui, S.K. Srivastava et al., 2004. Betulinic acid and its derivatives as anti-angiogenic agents. Bioorg. Med. Chem. Lett., 14(9): 2181-2184.
Direct Link -
Mukherjee, R., V. Kumar, S.K. Srivastava, S.K. Agarwal and A.C. Burman, 2006. Betulinic acid derivatives as anticancer agents: Structure activity relationship. Anti-Cancer. Agent. Me., 6(3): 271-279.
Direct Link -
Pollar, M., M. Jaroensutasinee and K. Jaroensutasinee, 2007. Morphometric analysis of Tor tambroides by stepwise discriminant and neural network analysis. World Acad. Sci. Eng. Technol., 33: 16-20.
-
Rajendran, P., M. Jaggi, M.K. Singh, R. Mukherjee and A.C. Burman, 2008. Pharmacological evaluation of c-3 modified Betulinic acid derivatives with potent anticancer activity. Invest. New Drug., 26(1): 25-34.
Direct Link -
Smits, J.R.M., W.J. Melssen, L.M.C. Buydens and G. Kateman, 1994. Using artificial neural networks for solving chemical problems: Part I. Multi-layer feed-forward networks. Chemometr. Intell. Lab., 22(2): 165-189.
Direct Link -
Specht, D.F., 1991. A general regression neural network. IEEE T. Neural Networ., 2(6): 568-576.
CrossRef PMid:18282872 -
Svozil, D., V. Kvasnicka and J. Pospichal, 1997. Introduction to multi-layer feed-forward neural networks. Chemometr. Intell. Lab., 39(1): 43-62.
CrossRef -
Thibeault, D., C. Gauthier, J. Legault, J. Bouchard, P. Dufour and A. Pichette, 2007. Synthesis and structure-activity relationship study of cytotoxic germanicane- and lupane-type 3beta-O-monodesmosidic saponins starting from betulin. Bioorgan. Med. Chem., 15(18): 6144-6157.
Direct Link -
Vouk, D., D. Malus and I. Halkijevic, 2011. Neural networks in economic analyses of wastewater systems. Expert Syst. Appl., 38(8): 10031-10035.
CrossRef -
Yang, D., H. Li, C. Cao, F. Chen, Y. Zhou and Z. Xiu 2014. Analysis of the oil content of rapeseed using artificial neural networks based on near infrared spectral data. J. Spectrosc., 2014: 5.
CrossRef -
Yasin, Y., M. Basri, F. Ahmad and A.B. Salleh, 2008. Response surface methodology as a tool to study the lipase-catalyzed synthesis of betulinic acid ester. J. Chem. Technol. Biot., 83(5): 694-698.
Direct Link -
Yegnanarayana, B., 2009. Artificial Neural Networks. PHI Learning Pvt. Ltd., New Delhi.
PMid:19813815 -
Yogeeswari, P. and D. Sriram, 2005. Betulinic acid and its derivatives: A review on their biological properties. Curr. Med. Chem., 12(6): 657-666.
Direct Link -
Zarev�cka, M. and Z. Wimmer, 2008. Plant products for pharmacology: Application of enzymes in their transformations. Int. J. Mol. Sci., 9(12): 2447-2473.
Direct Link -
Zhong, X., J. Li, H. Dou, S. Deng, G. Wang et al., 2013. Fuzzy nonlinear proximal support vector machine for land extraction based on remote sensing image. PloS One, 8(7): e69434.
CrossRef PMid:23936016 PMCid:PMC3720649
Competing interests
The authors have no competing interests.
Open Access Policy
This article is distributed under the terms of the Creative Commons Attribution 4.0 International License (http://creativecommons.org/licenses/by/4.0/), which permits unrestricted use, distribution, and reproduction in any medium, provided you give appropriate credit to the original author(s) and the source, provide a link to the Creative Commons license, and indicate if changes were made.
Copyright
The authors have no competing interests.
|
|
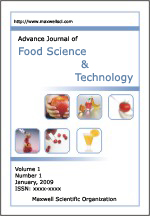 |
ISSN (Online): 2042-4876
ISSN (Print): 2042-4868 |
 |
Information |
|
|
|
Sales & Services |
|
|
|