Research Article | OPEN ACCESS
Discrimination of Rice Varieties using LS-SVM Classification Algorithms and Hyperspectral Data
1Jin Xiaming, 1, 2Sun Jun, 2Mao Hanping, 1Jiang Shuying, 2Li Qinglin and 1Chen Xingxing
1School of Electrical and Information Engineering
2Laboratory Venlo of Modern Agricultural Equipment, Jiangsu University, Zhenjiang 212013, P.R. China
Advance Journal of Food Science and Technology 2015 9:691-696
Received: July ‎18, ‎2014 | Accepted: September ‎13, ‎2014 | Published: March 25, 2015
Abstract
Fast discrimination of rice varieties plays a key role in the rice processing industry and benefits the management of rice in the supermarket. In order to discriminate rice varieties in a fast and nondestructive way, hyperspectral technology and several classification algorithms were used in this study. The hyperspectral data of 250 rice samples of 5 varieties were obtained using FieldSpec®3 spectrometer. Multiplication Scatter Correction (MSC) was used to preprocess the raw spectra. Principal Component Analysis (PCA) was used to reduce the dimension of raw spectra. To investigate the influence of different linear and non-linear classification algorithms on the discrimination results, K-Nearest Neighbors (KNN), Support Vector Machine (SVM) and Least Square Support Vector Machine (LS-SVM) were used to develop the discrimination models respectively. Then the performances of these three multivariate classification methods were compared according to the discrimination accuracy. The number of Principal Components (PCs) and K parameter of KNN, kernel function of SVM or LS-SVM, were optimized by cross-validation in corresponding models. One hundred and twenty five rice samples (25 of each variety) were chosen as calibration set and the remaining 125 rice samples were prediction set. The experiment results showed that, the optimal PCs was 8 and the cross-validation accuracy of KNN (K = 2), SVM, LS-SVM were 94.4, 96.8 and 100%, respectively, while the prediction accuracy of KNN (K = 2), SVM, LS-SVM were 89.6, 93.6 and 100%, respectively. The results indicated that LS-SVM performed the best in the discrimination of rice varieties.
Keywords:
Classification algorithm, hyperspectral technology, rice variety,
References
-
Barbin, D., G. Elmasry, D.W. Sun and P. Allen, 2012. Near-infrared hyperspectral imaging for grading and classification of pork. Meat Sci., 90(1): 259-268.
CrossRef PMid:21821367 -
Cen, H.Y., Y. He and M. Huang, 2007. Combination and comparison of multivariate analysis for the identification of orange varieties using visible and near infrared reflectance spectroscopy. Eur. Food Res. Technol., 225(5-6): 699-705.
CrossRef -
Gao, J.F., X.L. Li, F.L. Zhu and Y. He, 2013. Application of hyperspectral imaging technology to discriminate different geographical origins of Jatropha curcas L. seeds. Comput. Electron. Agr., 99: 186-193.
CrossRef -
Ji-yong, S., Z. Xiao-Bo, Z. Jie-wen, M. Han-ping, W. Kai-liang, C. Zheng-wei and H. Xiao-wei, 2011. Diagnostics of nitrogen deficiency in mini-cucumber plant by near infrared reflectance spectroscopy. Afr. J. Biotechnol., 10(85): 19687-19692.
-
Li, P., G. Du, W. Cai and X. Shao, 2012. Rapid and nondestructive analysis of pharmaceutical products using near-infrared diffuse reflectance spectroscopy. J. Pharmaceut. Biomed., 70: 288-294.
CrossRef PMid:22858521 -
Li, S.J., H. Wu, D.S. Wan and J. Zhu, 2011. An effective feature selection method for hyperspectral imaging classification based on genetic algorithm and support vector machine. Knowl-Based Syst., 24(1): 40-48.
CrossRef -
Liu, L. and M.O. Ngadi, 2013. Detecting fertility and early embryo development of chicken eggs using near-infrared hyperspectral imaging. Food Bioprocess. Technol., 6(9): 2503-2513.
CrossRef -
Peng, X.L., X. Li, X. Shi and S. Guo, 2014. Evaluation of the aroma quality of Chinese traditional soy paste during storage based on principal component analysis. Food Chem., 151: 532-538.
CrossRef PMid:24423567 -
Pholpho, T., S. Pathaveerat and P. Sirisomboon, 2011. Classification of longan fruit bruising using visible spectroscopy. J. Food Eng., 104(1): 169-172.
CrossRef -
Qin, J.W., K.L. Chao, M.S. Kim, 2012. Nondestructive evaluation of internal maturity of tomatoes using spatially offset Raman spectroscopy. Postharvest Biol. Tec., 71: 21-31.
CrossRef -
Sarbu, C., R.D. Nascu-Briciu, A. Kot-Wasik, S. Gorinstein, A. Wasik and J. Namiesnik, 2012. Classification and fingerprinting of kiwi and pomelo fruits by multivariate analysis of chromatographic and spectroscopic data. Food Chem., 130(4): 994-1002.
CrossRef -
Serranti, S., D. Cesare, F. Marini and G. Bonifazi, 2013. Classification of oat and groat kernels using NIR hyperspectral imaging. Talanta, 103: 276-284.
CrossRef PMid:23200388 -
Sirisomboon, P., M. Tanaka, T. Kojima and P. Williams, 2012. Nondestructive estimation of maturity and textural properties on tomato 'Momotaro' by near infrared spectroscopy. J. Food Eng., 112(3): 218-226.
CrossRef -
Teye, E., X.Y. Huang, H., Dai and Q. Chen, 2013. Rapid differentiation of Ghana cocoa beans by FT-NIR spectroscopy coupled with multivariate classification. Spectrochim. Acta A, 114: 183-189.
CrossRef PMid:23770507 -
Wang, J., K. Nakano, S. Ohashi, Y. Kubota, K. Takizawa and Y. Sasaki, 2011. Detection of external insect infestations in jujube fruit using hyperspectral reflectance imaging. Biosyst. Eng., 108(4): 345-351.
CrossRef -
Watanabe, K., I. Kobayashi, S. Saito, N. Kuroda and S. Noshiro, 2013. Nondestructive evaluation of drying stress level on wood surface using near-infrared spectroscopy. Wood Sci. Technol., 47(2): 299-315.
CrossRef -
Wu, D., J. Chen, B. Lu, L. Xiong, Y. He and Y. Zhang, 2012. Application of near infrared spectroscopy for the rapid determination of antioxidant activity of bamboo leaf extract. Food Chem., 135(4): 2147-2156.
CrossRef PMid:22980783 -
Zhang, X.C., J.Z. Wu and Y. Xu, 2012. Near Infrared Spectroscopy Technology and its Application in Modern Agriculture. Publishing House of Electronics Industry, Beijing, pp: 104-105.
-
Zhao, J.W., H. Lin, Q.S. Chen, X. Huang, Z.B. Sun and F. Zhou, 2010. Identification of egg's freshness using NIR and support vector data description. J. Food Eng., 98(4): 408-414.
CrossRef -
Zhu, F.L., D.R. Zhang, Y. He, F. Liu and D.W. Sun, 2013. Application of visible and near infrared hyperspectral imaging to differentiate between fresh and frozen-thawed fish fillets. Food Bioprocess. Technol., 6(10): 2931-2937.
CrossRef
Competing interests
The authors have no competing interests.
Open Access Policy
This article is distributed under the terms of the Creative Commons Attribution 4.0 International License (http://creativecommons.org/licenses/by/4.0/), which permits unrestricted use, distribution, and reproduction in any medium, provided you give appropriate credit to the original author(s) and the source, provide a link to the Creative Commons license, and indicate if changes were made.
Copyright
The authors have no competing interests.
|
|
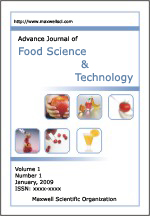 |
ISSN (Online): 2042-4876
ISSN (Print): 2042-4868 |
 |
Information |
|
|
|
Sales & Services |
|
|
|