Research Article | OPEN ACCESS
Research on Demand Prediction of Fresh Food Supply Chain Based on Improved Particle Swarm Optimization Algorithm
He Wang
School of International Trade and Economics, Jiangxi University of Finance and Economics,
Nanchang 330013, China
Advance Journal of Food Science and Technology 2015 10:804-809
Received: October 17, 2014 | Accepted: December 18, 2014 | Published: April 05, 2015
Abstract
Demand prediction of supply chain is an important content and the first premise in supply management of different enterprises and has become one of the difficulties and hot research fields for the researchers related. The paper takes fresh food demand prediction for example and presents a new algorithm for predicting demand of fresh food supply chain. First, the working principle and the root causes of the defects of particle swarm optimization algorithm are analyzed in the study; Second, the study designs a new cloud particle swarm optimization algorithm to guarantee the effectiveness of particles in later searching phase and redesigns its cloud global optimization searching method and crossover operation; Finally, a certain fresh food supply chain is taken for example to illustrate the validity and feasibility of the improved algorithm and the experimental results show that the improved algorithm can improve prediction accuracy and calculation efficiency when used for demand prediction of fresh food supply chain.
Keywords:
Demand prediction, fresh food, particle swarm optimization algorithm, supply chain management,
References
-
Amandle, S., 2010. A new method demand prediction for supply chain management. J. Manage. Sci., 13(1): 29-37.
-
Disney, S.M. and D.R. Towill, 2006. On the equivalence of control theoretic, differential, and difference equation approaches to modeling supply chains. Int. J. Prod. Econ., 101(4): 194-208.
CrossRef -
Duc, K. and R. Drezner, 2014. The quantifying bullwhip effect in a simple supply chain: The impact of forecasting, lead times and information. Manage. Sci., 46(2): 46-53.
-
Fang, S., X. Liu and W. Xiong, 2011. Multi-robot task allocation based on BP neural network algorithm. Ind. Eng. J., 19(5): 124-133.
-
Feng, Y. and J. Ma, 2013. Nonlinear method research on demand forefoundry for supply chain. J. Beijing Inst. Tech. Soc. Sci. Edn., 10(5): 82-86.
Direct Link -
Gao, G. and X. Zhang, 2014. An novel PSO algorithm for teaching evaluation. J. Educ. Manage., 18(12): 1134-1146.
-
Luong, L. and M. Phien, 2012. Measure of bullwhip effect in supply chains: the case of high order autoregressive demand process. Eur. J. Oper. Res., 183(2): 197-209.
CrossRef -
Mieach, S., 2012. An effective clustering algorithm with PSO. J. Manage. Technol., 11(4): 24-31.
-
Tebby, J., 2011. Analyzing the state space property of echo state networks for chaotic system prediction. J. Bus. Manage., 10(11): 1412-1417.
-
Zhu, J. and M. Tang, 2011. A measure of bullwhip effect in supply chains with a mixed autoregressive-moving average demand process. Eur. J. Oper. Res., 182(11): 652-662.
Competing interests
The authors have no competing interests.
Open Access Policy
This article is distributed under the terms of the Creative Commons Attribution 4.0 International License (http://creativecommons.org/licenses/by/4.0/), which permits unrestricted use, distribution, and reproduction in any medium, provided you give appropriate credit to the original author(s) and the source, provide a link to the Creative Commons license, and indicate if changes were made.
Copyright
The authors have no competing interests.
|
|
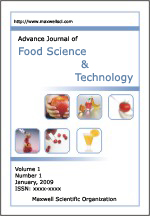 |
ISSN (Online): 2042-4876
ISSN (Print): 2042-4868 |
 |
Information |
|
|
|
Sales & Services |
|
|
|