Research Article | OPEN ACCESS
Evaluating Classification Strategies in Bag of SIFT Feature Method for Animal Recognition
Leila Mansourian, Muhamad Taufik Abdullah, Lilli Nurliyana Abdullah and Azreen Azman
Department of Multimedia, Faculty of Computer Science and Information Technology,
Universiti Putra Malaysia, 43400 UPM Serdang, Selangor, Malaysia
Research Journal of Applied Sciences, Engineering and Technology 2015 11:1266-1272
Received: October 29, 2014 | Accepted: December 27, 2014 | Published: August 15, 2015
Abstract
These days automatic image annotation is an important topic and several efforts are made to solve the semantic gap problem which is still an open issue. Also, Content Based Image Retrieval (CBIR) cannot solve this problem. One of the efficient and effective models for solving the semantic gap and visual recognition and retrieval is Bag of Feature (BoF) model which can quantize local visual features like SIFT perfectly. In this study our aim is to investigate the potential usage of Bag of SIFT Feature in animal recognition. Also, we specified which classification method is better for animal pictures.
Keywords:
Bag of feature, Content Based Image Retrieval (CBIR), feature quantization, image annotation, SIFT feature, Support Vector Machines (SVM),
References
-
Chang, C. and C. Lin, 2013. LIBSVM?: A library for support vector machines. ACM T. Intell. Syst. Technol., 2(3): 1-39.
CrossRef -
Chiang, C.C., 2013. Interactive tool for image annotation using a semi-supervised and hierarchical approach. Comp. Stand. Inter., 35(1): 50-58.
CrossRef -
Choi, M.J., J.J. Lim, A. Torralba and A.S. Willsky, 2010. Exploiting hierarchical context on a large database of object categories. Proceeding of the IEEE Computer Society Conference on Computer Vision and Pattern Recognition, pp: 129-136.
CrossRef -
Corinna Cortes, V.V., 1995. Support-vector networks. Mach. Learn., 20(3): 273-297.
CrossRef -
Deza, M.M. and E. Deza, 2009. Encyclopedia of Distances. Springer-Verlag, Berlin, Heidelberg, pp: 1-583.
CrossRef -
Dimitrovski, I., D. Kocev, S. Loskovska and S. Džeroski, 2011. Hierarchical annotation of medical images. Pattern Recogn., 44(10-11): 2436-2449.
CrossRef -
Fakhari, A. and A.M.E. Moghadam, 2013. Combination of classification and regression in decision tree for multi-labeling image annotation and retrieval. Appl. Soft Comput., 13(2): 1292-1302.
CrossRef -
Griffin, G., A. Holub and P. Perona, 2007. Caltech-256 object category dataset. Technical Report 7694, California Institute of Technology.
-
Hong, R., M. Wang, Y. Gao, D. Tao, X. Li and X. Wu, 2014. Image annotation by multiple-instance learning with discriminative feature mapping and selection. IEEE T. Cybern., 44(5): 669-680.
CrossRef PMid:23797314 -
Jiang, Y.G., Q. Dai, J. Wang, C.W. Ngo, X.Y. Xue and S.F. Chang, 2012. Fast semantic diffusion for large-scale context-based image and video annotation. IEEE T. Image Process., 21(6): 3080-3091.
CrossRef PMid:22345543 -
Lee, C.H., H.C. Yang and S.H. Wang, 2011. An image annotation approach using location references to enhance geographic knowledge discovery. Expert Syst. Appl., 38(11): 13792-13802.
CrossRef -
Liu, Y., D. Zhang, G. Lu and W.Y. Ma, 2007. A survey of content-based image retrieval with high-level semantics. Pattern Recogn., 40(1): 262-282.
CrossRef -
Liu, W., D. Tao, J. Cheng and Y. Tang, 2014. Multiview Hessian discriminative sparse coding for image annotation. Comput. Vis. Image Und., 118: 50-60.
CrossRef -
Lowe, D.G., 1999. Object recognition from local scale-invariant features. Proceeding of the 7th IEEE International Conference on Computer Vision, 2: 1150-1157.
CrossRef -
Mikolajczyk, K., B. Leibe and B. Schiele, 2005. Local features for object class recognition. Proceeding of the 10th IEEE International Conference on Computer Vision. Washington, DC, USA, pp: 1792-1799.
CrossRef -
Perona, P., 2005. A bayesian hierarchical model for learning natural scene categories. Proceeding of the IEEE Computer Society Conference on Computer Vision and Pattern Recognition (CVPR’05), 2: 524-531.
-
Qi, H. and W.E. Snyder, 1999. Content-based image retrieval in picture archiving and communications systems. J. Digit. Imaging, 12(2): 81-83.
CrossRef PMid:10342174 PMCid:PMC3452879 -
Tousch, A.M., S. Herbin and J.Y. Audibert, 2012. Semantic hierarchies for image annotation: A survey. Pattern Recogn., 45(1): 333-345.
CrossRef -
Tsai, C.F., 2012. Bag-of-words representation in image annotation: A review. ISRN Artif. Intell., 2012: 1-19.
CrossRef -
Zhang, D., M.M. Islam and G. Lu, 2012. A review on automatic image annotation techniques. Pattern Recogn., 45(1): 346-362.
CrossRef -
Zhou, X.S. and T.S. Huang, 2000. CBIR: From low-level features to high-level semantics. Proceeding of the SPIE, Image and Video Communication and Processing. San Jose, CA, 3974: 426-431.
PMid:11780524
Competing interests
The authors have no competing interests.
Open Access Policy
This article is distributed under the terms of the Creative Commons Attribution 4.0 International License (http://creativecommons.org/licenses/by/4.0/), which permits unrestricted use, distribution, and reproduction in any medium, provided you give appropriate credit to the original author(s) and the source, provide a link to the Creative Commons license, and indicate if changes were made.
Copyright
The authors have no competing interests.
|
|
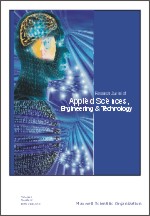 |
ISSN (Online): 2040-7467
ISSN (Print): 2040-7459 |
 |
Information |
|
|
|
Sales & Services |
|
|
|