Research Article | OPEN ACCESS
Traffic Analyzing and Controlling using Supervised Parametric Clustering in Heterogeneous Network
1D. Jayachitra and 2J. Jebamalar Tamilselvi
1Department of Computer Science, Nehru Memorial College, Bharathiar University, Puthanampatti, Coimbatore, Tamil Nadu, India
2Department of MCA, Jaya Engineering College, Chennai, Tamil Nadu, India
Research Journal of Applied Sciences, Engineering and Technology 2015 5:473-479
Received: March ‎14, ‎2015 | Accepted: April ‎1, ‎2015 | Published: October 15, 2015
Abstract
The aim of the study is to maximize class purity level of dynamic network structure using the Supervised Parametric Clustering (SPC) approach. An efficient means to ensure proper flow sequence on heterogeneous network is the effective analysis of network traffic and its smooth maintenance. Several works in the literature have focused on access logs for analyzing network traffic, but investigating supervised clustering model for network analysis has received relatively less attention. On the other hand, Peer-to-Peer Document Clustering maintain privacy inside the neighborhood data boundaries, but fails on extending dynamic structure that reduces the high class purity mapping. To maximize the class purity level of mapping, Supervised Parametric Clustering (SPC) approach is proposed in this study. SPC approach’s initial work is to analyze the flow sequences of packets in the heterogeneous network. During analyzing the data traffic flow, to ensure smoothening of network data flow, a method called binning is designed that smoothen the network data flow information discussing with neighborhood value range. Secondly, traffic data feature selection uses the Candy Best First wrapper technique for balancing the heterogeneous network traffic. The Candy Best First wrapper technique extracts the content to the particular selected feature source and translated into the relational form. Then, SPC follows the separation of medoids value points to cluster the collaborative classes of traffic data points. Finally, mapping of the SPC resultant data clusters is carried out to effectively analyze and control the traffic flow in the heterogeneous network. Experiment is carried out on the factors such as performance speedup rate, false positive rate and class purity mapping rate.
Keywords:
Best first wrapper technique, binning method, dynamic network structure, feature selection, fitness function, supervised parametric clustering,
References
- Chen, Y., S. Nyemba and B. Malin, 2012. Detecting anomalous insiders in collaborative information systems. IEEE T. Depend. Secure, 9(3): 322-344.
CrossRef - Guan, R., X. Shi, M. Marchese, C. Yang and Y. Liang, 2011. Text clustering with seeds affinity propagation. IEEE T. Knowl. Data En., 23(4): 627-637.
CrossRef
-
Hammouda, K.M. and M.S. Kamel, 2009. Hierarchically distributed peer-to-peer document clustering and cluster summarization. IEEE T. Knowl. Data En., 21(5): 681-698.
CrossRef
- Lee, D. and J. Lee, 2010. Dynamic dissimilarity measure for support-based clustering. IEEE T. Knowl. Data En., 22(6): 900-905.
CrossRef
- Leung, K.W.T. and D.L. Lee, 2010. Deriving concept-based user profiles from search engine logs. IEEE T. Knowl. Data En., 22(7): 969-982.
CrossRef
- Nguyen, D.T., L. Chen and C.K. Chan, 2012. Clustering with multiviewpoint-based similarity measure. IEEE T. Knowl. Data En., 24(6): 988-1001.
CrossRef
- Rashidi, P., D.J. Cook, L.B. Holder and M. Schmitter-Edgecombe, 2011. Discovering activities to recognize and track in a smart environment. IEEE T. Knowl. Data Eng., 23(4): 527-539.
CrossRef PMid:21617742 PMCid:PMC3100559
- Shehata, S., F. Karray and M.S. Kamel, 2010. An efficient concept-based mining model for enhancing text clustering. IEEE T. Knowl. Data En., 22(10): 1360-1371.
CrossRef
- Sreelakshmi, B.L. and M. Deepa, 2014. Optic disc and optic cup detection using superpixel classification based on PCA and mathematical morphology. Int. J. Invent. Comput. Sci. Eng., 1(2).
- Yen, L., M. Saerens and F. Fouss, 2011. Link analysis extension of correspondence analysis for mining relational databases. IEEE T. Knowl. Data En., 23(4): 481-495.
CrossRef
Competing interests
The authors have no competing interests.
Open Access Policy
This article is distributed under the terms of the Creative Commons Attribution 4.0 International License (http://creativecommons.org/licenses/by/4.0/), which permits unrestricted use, distribution, and reproduction in any medium, provided you give appropriate credit to the original author(s) and the source, provide a link to the Creative Commons license, and indicate if changes were made.
Copyright
The authors have no competing interests.
|
|
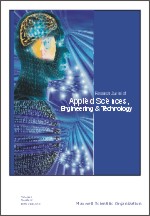 |
ISSN (Online): 2040-7467
ISSN (Print): 2040-7459 |
 |
Information |
|
|
|
Sales & Services |
|
|
|