Research Article | OPEN ACCESS
A Robust Scalable Model Using Hybrid Approach for the Detection of the Projected Outliers
1H. Sadawarti, 2G.S. Kalra and 3Kamal Malik
1RIMTIET, Punjab Technical University
2Lovely Professional University, Punjab
3MMICT and BM, MMU, Mullana, Haryana, India
Research Journal of Applied Sciences, Engineering and Technology 2016 6:642-649
Received: July 7, 2015 | Accepted: August 22, 2015 | Published: March 15, 2016
Abstract
The abnormal and anomalous observations even in the advanced technological era proves to be the biggest jolt to the concerned industry. To reduce and eliminate the outliers from the massive data streams, it is important to accurately highlight them from the higher dimensional data which is itself very challenging. In this study, a Scalable outlier detection model is proposed which is robust enough to resist and detect the projected outliers that are lying at some lower dimensional subspaces. This model exploits the problem of curse of dimensionality which is very frequent in large data streams and massive datasets. Rapid distance and density based approaches are used and then the probability density is measured by Gaussian Mixture Model. Baye's Probability is applied to the final observations so as confirm them as the projected outliers.
Keywords:
GMM, KDD, projected outliers,
References
-
Aggarwal, C.C. and P.S. Yu, 2000. Finding generalized projected clusters in higher dimensional spaces. Proceeding of the ACM SIGMOID International Conference on Management of Data, pp: 70-81.
-
Agrawal, R., J. Gehrke, D. Gunopulos and P. Raghavan, 1998. Automatic subspace clustering of high dimensional data for data mining applications. Proceeding of the ACM SIGMOD International Conference on Management of Data, Seattle, WA, pp: 94-105.
CrossRef -
Angiulli, F., S. Basta and C. Pizzuti, 2006. Distance-based detection and prediction of outliers. IEEE T. Knowl. Data En., 18(2): 145-160.
CrossRef -
Atkinson, A.C., 1994. Fast very robust methods for the detection of multiple outliers. J. Am. Stat. Assoc., 89(428): 1329-1339.
CrossRef -
Breunig, M.M., H.P. Kriegel, R.T. Ng and J. Sander, 2000. LOF: Identifying density-based local outliers. Proceeding of the 2000 ACM SIGMOD International Conference on Management of Data (SIGMOD '00). ACM, New York, USA, pp: 93-104.
CrossRef -
Danuser, G. and M. Stricker, 1998. Parametric model fitting: From inlier characterization to outlier detection. IEEE T. Pattern Anal., 20(3): 263-280.
CrossRef -
Day, N.E., 1969. Estimating the components of a mixture of normal distributions. Biometrica, 56(3): 463-474.
CrossRef -
Dempster, A.P., N.M. Liard and D.B. Rubin, 1977. Maximum likelihood from incomplete data via the EM algorithm. J. Roy. Stat. Soc. B Met., 39: 1-38.
-
Duda, R.O. and P.E. Hart, 1973. Pattern Classification and Scene Analysis. Wiley, New York.
-
Ester, M., H.P. Kriegel, J. Sander and X. Xu, 1996. A density-based algorithm for discovering clusters in large spatial databases with noise. Proceeding of the 2nd International Conference on Knowledge Discovery and Data Mining. AAAI Press, Portland, OR, pp: 226-231.
-
Fidler, S., D. Skocaj and A. Leonardis, 2006. Combining reconstructive and discriminative subspace methods for robust classification and regression by subsampling. IEEE T. Pattern Anal., 28(3): 337-350.
CrossRef PMid:16526421 -
Hasselblad, V., 1966. Estimation of parameters for a mixture of normal distributions. Technometrics, 8(3): 431-444.
CrossRef -
Hinneburg, A. and D.A. Keim, 1998. An efficient approach to clustering in large multimedia databases with noise. Proceeding of the 4th International Conference on Knowledge Discovery and Data Mining. New York City, pp: 58-65.
-
Knorr, E.M. and R.T. Ng, 1998. Algorithms for mining distance based outliers in large datasets. Proceeding of the 24th International Conference on Very Large Data Bases (VLDB, 1998), pp: 392-403.
-
Lazarevic, A., L. Ertoz, A. Ozgur, J. Srivastava and V. Kumar, 2013. A comparative study of anomaly detection schemes in network intrusion detection. Proceeding of the 3rd SIAM Conference on Data Mining.
-
Ramaswamy, S., R. Rastogi and K. Shim, 2000. Efficient algorithms for mining outliers from large data sets. Proceeding of the ACM SIGMOD International Conference on Management of Data (SIGMOD '00), pp: 427-438.
CrossRef PMid:10870986 -
Rocke, D.M. and D.L. Woodruff, 1996. Identification of outliers in multivariate data. J. Am. Stat. Assoc., 91(435): 1047-1061.
CrossRef -
Roeder, K. and L. Wasserman, 1997. Practical Bayesian density estimation using mixtures of normals. J. Am. Stat. Assoc., 92(439): 894-902.
CrossRef -
Rousseeuw, P.J. and A.M. Leory, 1987. Robust Regression and Outlier Detection. John Wiley and Sons, NY.
CrossRef -
Rousseeuw, P.J. and K. Van Driessen, 1999. A fast algorithm for the minimum covariance determinant estimator. Technometrics, 41(3): 212-223.
CrossRef -
Takeuchi, J. and K. Yamanishi, 2006. A unifying framework for detecting outliers and change points from time series. IEEE T. Knowl. Data En., 18(4): 482-492.
CrossRef -
Wolfe, J.H., 1970. Pattern clustering by multivariate mixture analysis. Multivar. Behav. Res., 5(3): 329-350.
CrossRef PMid:26812701 -
Zhang, J., 2009. Towards outlier detection for high-dimensional data streams using projected outlier analysis strategy. Ph.D. Thesis, Dalhousie University.
Competing interests
The authors have no competing interests.
Open Access Policy
This article is distributed under the terms of the Creative Commons Attribution 4.0 International License (http://creativecommons.org/licenses/by/4.0/), which permits unrestricted use, distribution, and reproduction in any medium, provided you give appropriate credit to the original author(s) and the source, provide a link to the Creative Commons license, and indicate if changes were made.
Copyright
The authors have no competing interests.
|
|
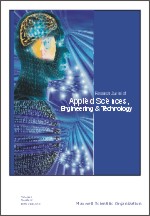 |
ISSN (Online): 2040-7467
ISSN (Print): 2040-7459 |
 |
Information |
|
|
|
Sales & Services |
|
|
|