Research Article | OPEN ACCESS
A New Clustering Algorithm of Data Mining
1Jamal Mbarki, 1El Miloud Jaara, 2Sidi Yasser Eljasouli, 1J. Mbarki and 1E.M. Jaara
1Laboratory of Computer Science Research (LARI), Faculty of Sciences, Mohamed Ier University, Oujda, Morocco
2Integrated and Efficient Solutions, IT.sprl, Belgium
Research Journal of Applied Sciences, Engineering and Technology 2016 6:427-431
Received: November 19, 2014 | Accepted: February 5, 2015 | Published: September 15, 2016
Abstract
The aim of this study is to present a new useful process of segmentation in large data, because organizing data into sensible groupings is now becoming the most fundamental modes of understanding and learning and enterprises have gathered a large amount of information over the last decades, the dilemma of managing such information by retrieving advantage in efficient way and less costing methods is becoming the key business success and takes top rows in strategy scale, different methods and techniques have been developed to reduce the data volumes to manageable structure and help enterprise to isolate the business value from the data sets. Clustering is one of those most important used data mining techniques. The algorithm that we will present can be helpful in CRM area. It can be potentially useful to better study customer profiles based on parameters called descriptor and may have a positive impact on customer retention and churn prevention, because the main aim of an ideal business is to optimise customer interactions by well remaining connected with customer.
Keywords:
Clustering, CRM, customer segmentation , similarity,
References
-
Agrawal, R., T. Imielinski and A. Swami, 1993. Database mining: A performance perspective. IEEE T. Knowl. Data En., 5(6): 914-925.
-
Fraley, C. and A.E. Raftery, 1998. How many clusters? Which clustering method? Answers via model-based cluster analysis. Comput. J., 41(8): 578-588.
CrossRef Direct Link -
Han, J. and M. Kamber, 2001. Data Mining: Concepts and Techniques. Morgan Kaufman, San Francisco, Calif.
-
Tan, P., V. Kumar and J. Srivastava, 2002. Selecting the right interestingness measure for association patterns. Technical Report 2002-112, Army High Performance Computing Research Center.
-
Tibshirani, R., 1996. Regression shrinkage and selection via the lasso. J. Roy. Stat. Soc. B Met., 58(1): 267-288.
Competing interests
The authors have no competing interests.
Open Access Policy
This article is distributed under the terms of the Creative Commons Attribution 4.0 International License (http://creativecommons.org/licenses/by/4.0/), which permits unrestricted use, distribution, and reproduction in any medium, provided you give appropriate credit to the original author(s) and the source, provide a link to the Creative Commons license, and indicate if changes were made.
Copyright
The authors have no competing interests.
|
|
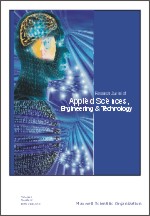 |
ISSN (Online): 2040-7467
ISSN (Print): 2040-7459 |
 |
Information |
|
|
|
Sales & Services |
|
|
|