Research Article | OPEN ACCESS
Features Selection and Pattern Classification of Electroencephalography Motor Imagery Tasks of Right Hand
1Ahmed A. Ibrahim, 2Mohammed I. Awad, 3Abdulwahab A. Alnaqi, 4Ann A. Abdel Kader and 2Farid A. Tolbah
1Department of Mechatronics Engineering, the Egyptian Academy of Engineering and
Advanced Technology
2Mechanical Engineering, Faculty of Engineering, Ain Shams University,Egypt
3Department of Automotive and Marine Engineering Technology, College of Technological Studies, The Public Authority for Applied Education and Training, Kuwait
4Department of Neurophysiology, Faculty of Medicine, Cairo University, Egypt
Research Journal of Applied Sciences, Engineering and Technology 2017 10:372-379
Received: February 3, 2017 | Accepted: March 16, 2017 | Published: October 15, 2017
Abstract
This study presentsa Brain Computer Interface (BCI) approach to detect the motor intents of the disabled people with right hand amputation. Electroencephalography (EEG) Motor Imagery (MI)-based Brain Computer Interface (BCI) systems have been recently used to improve the quality of life of disabled people. However, to naturally trigger particular applications (i.e., upper limb prostheses), independent BCIs appeal further paradigms to involve realistic motor imagery tasks. This study proposes an approach to classifying imagined hand gesture tasks, including the water glass gesture and the index pointer gesture of the right hand using OPENBCI as a consumer-grade EEG acquisition device. For three subjects, the data recorded by OPENBCI were sampled with a sampling rate of 250 Hz. The Minimum Redundancy Maximum Relevance (MRMR) technique was implemented as a feature selection method along with the Support Vector Machine (SVM) algorithm for classification. By obtaining a maximum classification accuracy of 91.7%, the results showed the feasibility of such Brain Computer Interface systems to detect different motor imagery tasks for the right hand. Consequently, upper limb prostheses could be manipulated using the intended motor imagery tasks.
Keywords:
Brain Computer Interface (BCI), ERD, feature selection, MRMR, OPENBCI,
References
- Chang, C.C. and C.J. Lin, 2011. LIBSVM: A library for support vector machines. ACM T. Intel. Syst. Tec., 2(3).
CrossRef
-
Bhagat, N.A., J. French, A. Venkatakrishnan, N. Yozbatiran, G.E. Francisco, M.K. O'Malley and J.L. Contreras-Vidal, 2014. Detecting movement intent from scalp EEG in a novel upper limb robotic rehabilitation system for stroke. Proceeding?of the 36th Annual International Conference of the IEEE Engineering in Medicine and Biology Society, pp: 4127-4130.
CrossRef
- Delorme, A. and S. Makeig, 2004. EEGLAB: An open source toolbox for analysis of single-trial EEG dynamics including independent component analysis. J. Neurosci. Method., 134(1): 9-21.
CrossRef PMid:15102499
- Dharmasena, S., K. Lalitharathne, K. Dissanayake, A. Sampath and A. Pasqual, 2013. Online classification of imagined hand movement using a consumer grade EEG device. Proceeding of the IEEE 8th International Conference on Industrial and Information Systems (ICIIS, 2013), pp: 537-541.
CrossRef
- Ding, C. and H. Peng, 2005. Minimum redundancy feature selection from microarray gene expression data. J. Bioinform. Comput. Biol., 3(2): 185-205.
CrossRef PMid:15852500
- Edelman, B., B. Baxter and B. He, 2014. Discriminating hand gesture motor imagery tasks using cortical current density estimation. Proceeding of the 36th Annual International Conference of the IEEE Engineering in Medicine and Biology Society, pp: 1314-1317.
CrossRef
-
Edelman, B.J., B. Baxter and B. He, 2016. EEG source imaging enhances the decoding of complex right-hand motor imagery tasks. IEEE T. Bio-Med. Eng., 63(1): 4-14.
Direct Link
-
Elasuty, B. and S. Eldawlatly, 2015. Dynamic bayesian networks for EEG motor imagery feature extraction. Proceeding of the 7th IEEE/EMBS Conference on Neural Engineering (NER), pp: 170-173.
CrossRef
-
He, B. and L. Ding, 2013. Neuroimaging. Springer, pp: 499-543.
-
Kamousi, B., A.N. Amini and B. He, 2007. Classification of motor imagery by means of cortical current density estimation and von neumann entropy. J. Neural Eng., 4(2): 17-25.
CrossRef PMid:17409476
- Liao, K., R. Xiao, J. Gonzalez and L. Ding, 2014. Decoding individual finger movements from one hand using human EEG signals. PLoS One, 9(1): e85192.
CrossRef PMid:24416360 PMCid:PMC3885680
- Liu, C., H. Wang, H. Pu, Y. Zhang and L. Zou, 2012. EEG feature extraction and pattern recognition during right and left hands motor imagery in brain-computer interface. Proceeding of the 5th International Conference on Biomedical Engineering and Informatics (BMEI, 2012), pp: 506-510.
CrossRef
-
Peng, H., F. Long and C. Ding, 2005. Feature selection based on mutual information: Criteria of max-dependency, max-relevance, and min-redundancy. IEEE T. Pattern Anal., 27(8): 1226-1238.
CrossRef PMid:16119262
- Pfurtscheller, G. and F.H. Lopes de Silva, 1999. Event-related EEG/MEG synchronization and desynchronization: Basic principles. Clin. Neurophysiol., 110: 1842-1857.
CrossRef
-
Renard, Y., F. Lotte, G. Gibert, M. Congedo, E. Maby, V. Delannoy, O. Bertrand and A. Lécuyer, 2010. OpenViBE: An open-source software platform to design, test, and use brain–computer interfaces in real and virtual environments. Presence, 19(1): 35-53.
CrossRef Direct Link
- Shiratori, T., H. Tsubakida, A. Ishiyama and Y. Ono, 2015. Three-class classification of motor imagery EEG data including "rest state" using filter-bank multi-class common spatial pattern. Proceeding of the 3rd International Winter Conference on Brain-Computer Interface (BCI), pp: 3-6.
CrossRef
- Soman, K., P. Reddy and H. Lakany, 2013. Detection of pre movement event — Related desynchronization from single trial EEG signal. Proceeding of the IEEE Conference on Information and Communication Technologies (ICT), pp: 788-792.
CrossRef
-
Wang, X., A. Wang, S. Zheng, Y. Lin and M. Yu, 2014. A multiple autocorrelation analysis method for motor imagery EEG feature extraction. Proceeding of the 26th Chinese Control and Decision Conference (CCDC, 2014), pp: 3000-3004.
CrossRef
Competing interests
The authors have no competing interests.
Open Access Policy
This article is distributed under the terms of the Creative Commons Attribution 4.0 International License (http://creativecommons.org/licenses/by/4.0/), which permits unrestricted use, distribution, and reproduction in any medium, provided you give appropriate credit to the original author(s) and the source, provide a link to the Creative Commons license, and indicate if changes were made.
Copyright
The authors have no competing interests.
|
|
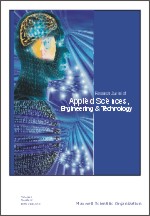 |
ISSN (Online): 2040-7467
ISSN (Print): 2040-7459 |
 |
Information |
|
|
|
Sales & Services |
|
|
|