Research Article | OPEN ACCESS
Bioinspired Engineering and Complex Systems Engineering: A Theoretical Framework for Water Management
Rodriguez Miranda, Juan Pablo, Garcia-Ubaque, Cesar Augusto and Sanchez Cespedes, Juan Manuel
Francisco Jose de Caldas District University, Bogota D.C., Carrera 7 # 40 B-53, 110221, Colombia
Research Journal of Applied Sciences, Engineering and Technology 2018 8:281-287
Received: October 20, 2017 | Accepted: February 7, 2018 | Published: August 15, 2018
Abstract
This study reviews the state of the art of different existing modeling tools applied in management water systems. Initially, is carried a conceptual review of several modeling systems, applied by the scientific community to model phenomena presented in reality. Emphasizing the modeling tools that serve to describe non-linear systems with high levels of complexity, such as Bio-inspired engineering and Complex Systems. Among the tools described are artificial intelligence, bio-inspired algorithms, artificial neuronal network, evolutionary algorithms, fuzzy logic, data mining, Bayesian networks, genetic algorithms, emerging systems, ant colony, particle swarm and cellular automata. Then, a review of scientific articles is carried out, showing how these tools have been used for water management systems, for example, making decision in water resources systems, consumption prediction models, quantity, flow, water quality, also for analysis of water pollution sources and wastewater treatment systems, among others. Then, it presents some future guidelines, in which it proposed that these modeling tools could be very useful for the planning and environmental planning of watersheds. Finally, it concluded that these tools have used to solve problems of water resources optimization but very little in the water environmental planning of a river basin.
Keywords:
Decision making, forecasting models, modeling, neural networks, optimization, water management,
References
-
Afshar, A., A.K. Ziaraty, A. Kaveh and F. Sharifi, 2009. Nondominated archiving multicolony ant algorithm in time-cost trade-off optimization. J. Constr. Eng. M., 135(7).https://doi.org/10.1061/(ASCE)0733-9364(2009)135:7(668)
CrossRef -
Alarcón, F., M.M.E. Alemany, C. Lario and R.F. Oltra, 2009. Modelo conceptual para el desarrollo de modelos matemáticos de ayuda a la toma de decisiones en el proceso colaborativo de comprometer pedidos. Proceeding of the XIII Congreso de Ingeniería de Organización. Barcelona, pp: 1-12.
Direct Link -
Armendáriz, E., P. Carbo, C.A. Hernández Bocanegra J.L. Hernandez, E. Martínez Peña, E. Rocha-Rangel and J.A. Rodríguez Garcia, 2014. Ingeniería Bioinspirada. Universidad Politécnica de Victoria, México D.F.
Direct Link -
Babea, I., 2010. El problema del agua y la inteligencia artificial. Universidad Carlos III de Madrid, Madrid.
-
Bakhtiari, A.S. and N. Bougila, 2014. A variational Bayes model for count data learning and classification. Eng. Appl. Artif. Intel., 35: 176-186.
CrossRef -
Beck, M.B., 2005. Vulnerability of water quality in intensively developing urban watersheds. Environ. Modell. Softw., 20(4): 381-400.
CrossRef -
Benítez, R., 2013. Inteligencia Artificial Avanzada. Fundación Universidad Oberta de Catalu-a, Barcelona.
-
Bonini, C.P., W.H. Hausman, H. Bierman and C.A. de Baroón, 2000. Análisis Cuantitativo Para Los Negocios. 9th Edn., McGraw-Hill, Santafeé de Bogotá.
-
Borges, C., 2013. Algoritmos Bioinspirados. Universidad de Cantabria, Cantabria.
-
Cano Esquibel, J.G., 2014. Metódos de inteligencia artificial aplicados a química computacional en entornos de computación de alto rendimiento. Ph.D. Thesis, Universidad de Alicante, Alicante.
-
Cárdenas Cardona, A., 2012. Inteligencia Artificial, métodos Bio-Inspitados: Un enfoque funcional para las ciencias de la computación. Thesis, Universidad Tecnológica de Pereira, Pereira.
Direct Link -
Carvajal Serna, L.F., 2013. Un modelo de gestión de la calidad y cantidad de agua con lógica difusa gris para el río Aburrá. Revista Ingenierías Universidad de Medellín, 12(22): 59-74.https://doi.org/10.22395/rium.v12n22a5
CrossRef -
Chen, S.H. and C.H. Yeh, 1997. Toward a computable approach to the efficient market hypothesis: An application of genetic programming. J. Econ. Dynam. Control, 21(6): 1043-1063.https://doi.org/10.1016/S0165-1889(97)82991-0
CrossRef -
Dogan, E., B. Sengorur and R. Koklu, 2009. Modeling biological oxygen demand of the Melen River in Turkey using an artificial neural network technique. J. Environ. Manage., 90(2): 1229-1235.https://doi.org/10.1016/j.jenvman.2008.06.004.PMid:18691805
CrossRef PMid:18691805 -
Dominguez, E., 2000. Protocolo para la modelación matemática de procesos hidrológicos. Meteorol. Colomb., 2:33-38.
-
Domokos, G. and I. Scheuring, 2004. Discrete and continuous state population models in a noisy world. J. Theor. Biol., 227(4): 535-545.https://doi.org/10.1016/j.jtbi.2003.08.017.PMid:15038988
CrossRef PMid:15038988 -
Dumedah, G., 2015. Toward essential union between evolutionary strategy and data assimilation for model diagnostics: An application for reducing the search space of optimization problems using hydrologic genome map. Environ. Modell. Softw., 69: 342-352.https://doi.org/10.1016/j.envsoft.2014.09.025
CrossRef -
Elbeltagi, E., T. Hegazy and D. Grierson, 2005. Comparison among five evolutionary-based optimization algorithms. Adv. Eng. Inform., 19(1): 43-53.https://doi.org/10.1016/j.aei.2005.01.004
CrossRef -
Farmani, R., G. Walters and D. Savic, 2006. Evolutionary multi-objective optimization of the design and operation of water distribution network: Total cost vs. reliability vs. water quality. J. Hydroinform., 8(3): 165-179.https://doi.org/10.2166/hydro.2006.019b
CrossRef -
Fernández Mejuto, M., A. Vela Mayorga and S. Casta-o Fernández, 1997. La utilización de modelos en hidrología. Ensayos: Revista de la Facultad de Educación de Albacete, 12: 305-318.
-
Gómez, J.A.L., 2015. Algoritmos bioinspirados aplicados al control de formaciones de múltiples nanorobots móviles. Tech Degree Work. Escuela Superior de Informática, Universidad de Castilla- La Mancha, Ciudad Real.
-
Gómez, N. and C. Maldonado, 2011. Sistemas Bio-Inspirados: un marco teórico para la ingeniería de sistemas complejos. Editorial Universidad del Rosario, Bogotá, D.C.
-
Grieu, S., A. Traoré, M. Polit and J. Colprim, 2005. Prediction of parameters characterizing the state of a pollution removal biologic process. Eng. Appl. Artif. Intel., 18(5): 559-573.
CrossRef -
Hamed, M.M., M.G. Khalafallah and E.A. Hassanien, 2004. Prediction of wastewater treatment plant performance using artificial neural networks. Environ. Modell. Softw., 19(10): 919-928.https://doi.org/10.1016/j.envsoft.2003.10.005
CrossRef -
Hamoda, M.F., I.A. Al-Ghusain and A.H. Hassan, 1999. Integrated wastewater treatment plant performance evaluation using artificial neural networks. Water Sci. Technol., 40(7): 55-65.https://doi.org/10.2166/wst.1999.0327
CrossRef -
Harrell, L.J. and R. Ranjithan, 2003. Detention pond design and land use planning for watershed management. J. Water Res. Pl., 129(2).https://doi.org/10.1061/(ASCE)0733-9496(2003)129:2(98)
CrossRef -
Hong, Y.S.T., M.R. Rosen and R. Bhamidimarri, 2003. Analysis of a municipal wastewater treatment plant using a neural network-based pattern. Water Res., 37(7): 1608-1618.https://doi.org/10.1016/S0043-1354(02)00494-3
CrossRef -
Hurtado, J., 2000. Metodología de la Investigación Holística. 3rd Edn., Fundación Sypal, Caracas.
-
Ito, K., Z.X. Xu, K. Jinno, T. Kohiri and A. Kawamura, 2001. Decision support system for surface water planning in river basins. J. Water Res. Plan. Man., 127(4): 272-276.https://doi.org/10.1061/(ASCE)0733-9496(2001)127:4(272)
CrossRef -
Izquierdo, J., I. Montalvo, R. Pérez and V.S. Fuertes, 2008. Design optimization of wastewater collection networks by PSO. Comput. Math. Appl., 56(3): 777-784.https://doi.org/10.1016/j.camwa.2008.02.007
CrossRef -
Kar, A.K., 2016. Bio inspired computing-A review of algorithms and scope of applications. Expert Syst. Appl., 59: 20-32.https://doi.org/10.1016/j.eswa.2016.04.018
CrossRef -
Kaveh, A. and S. Talatahari, 2009. Particle swarm optimizer, ant colony strategy and harmony search scheme hybridized for optimization of truss structures. Comput. Struct., 87(5-6): 267-283.https://doi.org/10.1016/j.compstruc.2009.01.003
CrossRef -
Kaveh, A., B. Mirzaei and A. Jafarvand, 2015. An improved magnetic charged system search for optimization of truss structures with continuous and discrete variables. Appl. Soft Comput., 28: 400-410.https://doi.org/10.1016/j.asoc.2014.11.056
CrossRef -
Ketabchi, H. and B. Ataie-Ashtiani, 2015. Evolutionary algorithms for the optimal management of coastal groundwater: A comparative study toward future challenges. J. Hydrol., 520: 193-213.https://doi.org/10.1016/j.jhydrol.2014.11.043
CrossRef -
Lerma, N., J. Paredes-Arquiola, J. Andreu, A. Solera and G.M. Sechi, 2015. Assessment of evolutionary algorithms for optimal operating rules design in real water resource systems. Environ. Modell. Softw., 69: 425-436.https://doi.org/10.1016/j.envsoft.2014.09.024
CrossRef -
Li, M. and P. Guo, 2014. A multi-objective optimal allocation model for irrigation water resources under multiple uncertainties. Appl. Math. Model., 38(19-20): 4897-4911.https://doi.org/10.1016/j.apm.2014.03.043
CrossRef -
Li, Y.P., G.H. Huang, Y.F. Huang and H.D. Zhou, 2009. A multistage fuzzy-stochastic programming model for supporting sustainable water-resources allocation and management. Environ. Modell. Softw., 24(7): 786-797.https://doi.org/10.1016/j.envsoft.2008.11.008
CrossRef -
Maier, H.R., Z. Kapelan, J. Kasprzyk, J. Kollat, L.S. Matott, M.C. Cunha et al., 2014. Evolutionary algorithms and other metaheuristics in water resources: Current status, research challenges and future directions. Environ. Modell. Softw., 62: 271-299.https://doi.org/10.1016/j.envsoft.2014.09.013
CrossRef -
Maldonado, C. and N. Gómez, 2010. Modelamiento y simulación de sistemas complejos. Editorial Universidad del Rosario, Bogotá D.C.
-
Mediero, L., 2007. Pronóstico probabilístico de caudales de avenida mediante redes bayesianas aplicadas sobre un modelo hidrológico distribuido. Ph.D. Thesis, Universidad Politécnica de Madrid, Madrid, Espa-a.
-
Mjalli, F.S., S. Al-Asheh and H.E. Alfadala, 2007. Use of artificial neural network black-box modeling for the prediction of wastewater treatment plants performance. J. Environ. Manage., 83(3): 329-338.https://doi.org/10.1016/j.jenvman.2006.03.004.PMid:16806660
CrossRef PMid:16806660 -
Mori, J. and V. Mahalec, 2015. Planning and scheduling of steel plates production. Part I: Estimation of production times via hybrid Bayesian networks for large domain of discrete variables. Comput. Chem. Eng., 79: 113-134.https://doi.org/10.1016/j.compchemeng.2015.02.005
CrossRef -
Perez-Pedini, C., J. Limbrunner and R.M. Vogel, 2005. Optimal location of infiltration-based best management practices for storm water management. J. Water Res. Pl., 131(6).https://doi.org/10.1061/(ASCE)0733-9496(2005)131:6(441)
CrossRef -
Ponce, P., 2010. Inteligencia artificial con aplicaciones a la ingeniería. Ed. Alfaomega, Mexico.
-
Rani, D., S.K. Jain, D.K. Srivastava and M. Perumal, 2013. Genetic algorithms and their applications to water resources systems. Metaheuristics Water, Geotech. Trans. Eng., pp: 43-78.
Direct Link -
Read, L., K. Madani and B. Inanloo, 2014. Optimality versus stability in water resource allocation. J. Environ. Manage., 133: 343-354.https://doi.org/10.1016/j.jenvman.2013.11.045.PMid:24412983
CrossRef PMid:24412983 -
Refsgaard, J. and M. Abbott, 1996. The Role of Distributed Hydrological Modelling in Water Resources Management. In: Refsgaard, J. and M. Abbott (Eds.), Distributed Hydrological Modelling. Kluwer Academic Publishers, Dordrecht, pp: 1-16.
-
Roach, T., Z. Kapelan and R. Ledbetter, 2015. Comparison of info-gap and robust optimisation methods for integrated water resource management under severe uncertainty. Proc. Eng., 119: 874-883.https://doi.org/10.1016/j.proeng.2015.08.955
CrossRef -
Seleshi, Y., G.R. Damarée and J.W. Delleur, 1994. Sunspot numbers as a possible indicator of annual rainfall at Addis Ababa, Ethiopia. Int. J. Climatol., 14(8): 918-923.https://doi.org/10.1002/joc.3370140807
CrossRef -
Shi, B., H.W. Lu, L.X. Ren and L. He, 2014. A fuzzy inexact two-phase programming approach to solving optimal allocation problems in water resources management. Appl. Math. Modell., 38(23): 5502-5514.https://doi.org/10.1016/j.apm.2014.04.005
CrossRef -
Singh, K.P., A. Basant, A. Malik and G. Jain, 2009. Artificial neural network modeling of the river water quality-A case study. Ecol. Modell., 220(6): 888-895.https://doi.org/10.1016/j.ecolmodel.2009.01.004
CrossRef -
Soler, E.M., V.A. de Sousa and G.R.M. da Costa, 2012. A modified primal-dual logarithmic-barrier method for solving the optimal power flow problem with discrete and continuous control variables. Eur. J. Oper. Res., 222(3): 616-622.https://doi.org/10.1016/j.ejor.2012.05.021
CrossRef -
Southworth, J., D. Munroe and H. Nagendra, 2004. Land cover change and landscape fragmentation-comparing the utility of continuous and discrete analyses for a western Honduras region. Agr. Ecosyst. Environ., 101(2-3):185-205.https://doi.org/10.1016/j.agee.2003.09.011
CrossRef -
Tan, R., K.J. Col-long, D.C.Y. Foo, S. Hul and D.K.S. Ng, 2008. A methodology for the design of efficient resource conservation networks using adaptive swarm intelligence. J. Clean Prod., 16(7): 822-832.https://doi.org/10.1016/j.jclepro.2007.04.004
CrossRef -
Taormina, R., K.W. Chau and R. Sethi, 2012. Artificial neural network simulation of hourly groundwater levels in a coastal aquifer system of the Venice lagoon. Eng. Appl. Artif. Intel., 25(8): 1670-1676.https://doi.org/10.1016/j.engappai.2012.02.009
CrossRef -
Tomenko, V., S. Ahmed and V. Popov, 2007. Modelling constructed wetland treatment system performance. Ecol. Model., 205(3-4): 355-364.https://doi.org/10.1016/j.ecolmodel.2007.02.030
CrossRef -
Vergel, G., 2010. Metodología. Un manual para la elaboración de diseños y proyectos de investigación. Compilación y Ampliación Temática, Publicaciones Corporación Unicosta: Barranquilla.
PMCid:PMC3018654 -
West, D. and S. Dellana, 2011. An empirical analysis of neural network memory structures for basin water quality forecasting. Int. J. Forecasting, 27(3):777-803.https://doi.org/10.1016/j.ijforecast.2010.09.003
CrossRef -
Xu, J. and X. Zhou, 2013. Approximation based fuzzy multi-objective models with expected objectives and chance constraints: Application to earth-rock work allocation. Inform. Sciences, 238:75-95.https://doi.org/10.1016/j.ins.2013.02.011
CrossRef -
Yan, T., X. Zhou, J. Gang, M. Liechty, J. Xu and B. Lev, 2015. Administrative and market-based allocation mechanism for regional water resources planning. Resour. Conserv. Recy., 95:156-173.https://doi.org/10.1016/j.resconrec.2014.12.011
CrossRef -
Zeng, X.T., Y.P. Ling, W. Huang, X. Chen and A.M. Bao, 2014. Two-stage credibility-constrained programming with Hurwicz criterion (TCP-CH) for planning water resources management. Eng. Appl. Artif. Intel., 35: 164-175.https://doi.org/10.1016/j.engappai.2014.06.021
CrossRef -
Zhao, Y., A. Sharma, B. Sivakumar, L. Marshall, P. Wang and J. Jiang, 2014. A Bayesian method for multi-pollution source water quality model and seasonal water quality management in river segments. Environ. Modell. Softw., 57: 216-226.
CrossRef
Competing interests
The authors have no competing interests.
Open Access Policy
This article is distributed under the terms of the Creative Commons Attribution 4.0 International License (http://creativecommons.org/licenses/by/4.0/), which permits unrestricted use, distribution, and reproduction in any medium, provided you give appropriate credit to the original author(s) and the source, provide a link to the Creative Commons license, and indicate if changes were made.
Copyright
The authors have no competing interests.
|
|
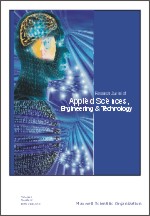 |
ISSN (Online): 2040-7467
ISSN (Print): 2040-7459 |
 |
Information |
|
|
|
Sales & Services |
|
|
|