Research Article | OPEN ACCESS
An Empirical Study of Combining Boosting-BAN and Boosting-MultiTAN
1Xiaowei Sun and 2Hongbo Zhou
1Software College, Shenyang Normal University
2BMW Brilliance Automotive Ltd. Co, Shenyang 110034 China
Research Journal of Applied Sciences, Engineering and Technology 2013 24:5550-5555
Received: September 24, 2012 | Accepted: November 12, 2012 | Published: May 30, 2013
Abstract
An ensemble consists of a set of independently trained classifiers whose predictions are combined when classifying novel instances. Previous research has shown that an ensemble as a whole is often more accurate than any of the single classifiers in the ensemble. Boosting-BAN classifier is considered stronger than Boosting-Multi TAN on noise-free data. However, there are strong empirical indications that Boosting-MultiTAN is much more robust than Boosting-BAN in noisy settings. For this reason, in this study we built an ensemble using a voting methodology of Boosting-BAN and Boosting-MultiTAN ensembles with 10 sub-classifiers in each one. We performed a comparison with Boosting-BAN and Boosting-MultiTAN ensembles with 25 sub-classifiers on standard benchmark datasets and the proposed technique was the most accurate.
Keywords:
Bayesian network classifier, combination method, data mining, boosting,
Competing interests
The authors have no competing interests.
Open Access Policy
This article is distributed under the terms of the Creative Commons Attribution 4.0 International License (http://creativecommons.org/licenses/by/4.0/), which permits unrestricted use, distribution, and reproduction in any medium, provided you give appropriate credit to the original author(s) and the source, provide a link to the Creative Commons license, and indicate if changes were made.
Copyright
The authors have no competing interests.
|
|
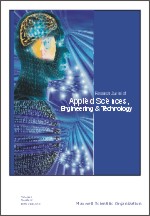 |
ISSN (Online): 2040-7467
ISSN (Print): 2040-7459 |
 |
Information |
|
|
|
Sales & Services |
|
|
|