Research Article | OPEN ACCESS
Learning to Classify Texture Objects by Particle Swarm Optimization Algorithm
1Ye Zhiwei, 1Chen Hongwei, 1Liu Wei, 1Wang Chunzhi and 2Lai Xudong
1Department of Computer Science, Hubei University of Technology, Wuhan 430068, China
2Department of Remote Sensing and Information Engineering, Wuhan University, Wuhan 430079, China
Research Journal of Applied Sciences, Engineering and Technology 2013 3:990-995
Received: June 23, 2012 | Accepted: July 28, 2012 | Published: January 21, 2013
Abstract
Texture is an important visual attribute used to describe images. There are many methods available for texture analysis. However, most of these methods are object to variant rotation and changing scale of the images. Hence, this study presents a novel approach for texture analysis. The approach applies the Particle Swarm Optimization Algorithm in learning the texture filters for texture classifications. In this approach, the texture filter is regarded as the particle; the population of particle is iteratively evaluated according to a statistical performance index corresponding to object classification ability and evolves into the optimal filter using the evolution principles of Particle Swarm Optimization Algorithm. The method has been validated on aerial images and results indicate that proposed method is feasible for texture analysis.
Keywords:
Image segmentation, particle swarm optimization algorithm, texture classification, tuned filter,
Competing interests
The authors have no competing interests.
Open Access Policy
This article is distributed under the terms of the Creative Commons Attribution 4.0 International License (http://creativecommons.org/licenses/by/4.0/), which permits unrestricted use, distribution, and reproduction in any medium, provided you give appropriate credit to the original author(s) and the source, provide a link to the Creative Commons license, and indicate if changes were made.
Copyright
The authors have no competing interests.
|
|
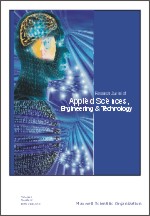 |
ISSN (Online): 2040-7467
ISSN (Print): 2040-7459 |
 |
Information |
|
|
|
Sales & Services |
|
|
|